This is the multi-page printable view of this section. Click here to print.
Postgres
- pg_exporter v1.0.0 Released – Next-Level PostgreSQL Observability
- OrioleDB is Here! 4x Performance, Zero Bloat, Decoupled Storage
- OpenHalo: PostgreSQL Now Speaks MySQL Wire Protocol!
- PGFS: Using PostgreSQL as a File System
- PostgreSQL Frontier
- Pig, The PostgreSQL Extension Wizard
- Don't Update! Rollback Issued on Release Day: PostgreSQL Faces a Major Setback
- PostgreSQL 12 Reaches End-of-Life as PG 17 Takes the Throne
- The idea way to install PostgreSQL Extensions
- PostgreSQL 17 Released: The Database That's Not Just Playing Anymore!
- Can PostgreSQL Replace Microsoft SQL Server?
- Whoever Integrates DuckDB Best Wins the OLAP World
- StackOverflow 2024 Survey: PostgreSQL Is Dominating the Field
- Self-Hosting Dify with PG, PGVector, and Pigsty
- PGCon.Dev 2024, The conf that shutdown PG for a week
- Postgres is eating the database world
- Technical Minimalism: Just Use PostgreSQL for Everything
- ParadeDB: ElasticSearch Alternative in PG Ecosystem
- The Astonishing Scalability of PostgreSQL
- PostgreSQL Crowned Database of the Year 2024!
- PostgreSQL Convention 2024
- FerretDB: When PG Masquerades as MongoDB
- PostgreSQL, The most successful database
- How Powerful Is PostgreSQL?
- Why Is PostgreSQL the Most Successful Database?
- Pigsty: The Production-Ready PostgreSQL Distribution
- Why PostgreSQL Has a Bright Future
- What Makes PostgreSQL So Awesome?
pg_exporter v1.0.0 Released – Next-Level PostgreSQL Observability
We’re delighted to announce pg_exporter v1.0.0, an advanced open-source Prometheus exporter that takes PostgreSQL observability to the next level.
Built for DBAs and developers who need deep insight, pg_exporter exposes 600 + metrics—roughly 3 K – 20 K time series per instance — covering core PostgreSQL internals, popular extensions such as TimescaleDB, Citus, pg_stat_statements, pg_wait_sampling, and even pgBouncer, all through a single, fully customizable exporter.
Unlike other exporters, pg_exporter values customizability: every metric lives in a YAML definition, so you can add, modify, or extend metrics without recompiling. The configuration allows fine-grained control over collection logic — PostgreSQL version branching, caching, timeouts, pre-condition queries, a health-check API, and live reload & replanning are all built in.
Battle-tested for more than six years in production clusters exceeding 25K+ CPU cores, pg_exporter also powers the Pigsty observability stack — see it in action in the live demo.
Version 1.0.0 brings a host of new features, including early support for PostgreSQL 18 — ready even before PG 18 beta release. Explore 50 + pre-defined collectors, or create your own (including app-specific metrics via SQL) simply by adding new configs.
Enjoy next-level insight into your PostgreSQL ecosystem with pg_exporter v1.0.0!
Features
- Highly Customizable: Define almost all metrics through declarative YAML configs
- Full Coverage: Monitor both PostgreSQL (10-18+) and pgBouncer (1.8-1.24+) in single exporter
- Fine-grained Control: Configure timeout, caching, skip conditions, and fatality per collector
- Dynamic Planning: Define multiple query branches based on different conditions
- Self-monitoring: Rich metrics about pg_exporter itself for complete observability
- Production-Ready: Battle-tested in real-world environments across 12K+ cores for 6+ years
- Auto-discovery: Automatically discover and monitor multiple databases within an instance
- Health Check APIs: Comprehensive HTTP endpoints for service health and traffic routing
- Extension Support:
timescaledb
,citus
,pg_stat_statements
,pg_wait_sampling
,…
OrioleDB is Here! 4x Performance, Zero Bloat, Decoupled Storage
OrioleDB - while the name might make you think of cookies, it’s actually named after the songbird. But whether you call it Cookie DB or Bird DB doesn’t matter - what matters is that this PG storage engine extension + kernel fork is genuinely fascinating, and it’s about to hit prime time.
As Zheap’s successor, I’ve been watching OrioleDB for quite a while. It has three major selling points: performance, operability, and cloud-native capabilities. Let me give you a quick tour of this PG kernel newcomer, along with some recent work I’ve done to help you get it up and running.
Extreme Performance: 4x Throughput
While hardware performance is overkill for most OLTP databases these days, hitting the single-node write throughput ceiling isn’t exactly rare - it’s usually what drives people to shard their databases.
OrioleDB aims to solve this. According to their homepage, they achieve 4x PostgreSQL’s read/write throughput - a pretty wild number. A 40% performance boost wouldn’t justify adopting a new storage engine, but 400%? Now that’s an interesting proposition.
Plus, OrioleDB claims to significantly reduce resource consumption in OLTP scenarios, notably lowering disk IOPS usage.
The secret sauce includes several optimizations over PG heap tables: ditching FS Cache, direct memory-to-storage page linking, lock-free memory page access, MVCC via UNDO logs/rollback segments instead of PG’s REDO, and row-level WAL that’s easier to parallelize.
Haven’t benchmarked it myself yet, but it’s tempting. Might grab a server and give it a spin soon.
Zero Headaches: Simplified Ops
PostgreSQL’s most notorious pain points are XID Wraparound and table bloat - both stemming from its MVCC design.
PostgreSQL’s default storage engine was designed with “infinite time travel” in mind, using an append-only MVCC approach - DELETEs are marked-for-deletion, and UPDATEs are delete-mark-plus-new-version.
While this design has perks - non-blocking reads/writes, instant rollbacks regardless of transaction size, and minimal replication lag - it’s given PostgreSQL users their fair share of headaches. Even with modern hardware and automatic vacuum, a high-standard PostgreSQL setup still needs to keep an eye on bloat and garbage collection.
OrioleDB tackles this with a new storage engine - think Oracle/MySQL-style approach, inheriting both their pros and cons. Using new MVCC practices, OrioleDB tables say goodbye to bloat and XID wraparound concerns.
Of course, there’s no free lunch - you inherit the downsides too: large transaction issues, slower rollbacks, and analytical performance trade-offs. But it excels at what it aims for: maximum OLTP CRUD performance.
Most importantly, it’s a PG extension - an optional storage engine that plays nice with PG’s native heap tables. You can mix and match based on your needs, letting your extreme OLTP tables shine where it counts.
-- Enable OrioleDB extension (Pigsty has it ready)
CREATE EXTENSION orioledb;
CREATE TABLE blog_post
(
id int8 NOT NULL,
title text NOT NULL,
body text NOT NULL,
PRIMARY KEY(id)
) USING orioledb; -- Use OrioleDB storage engine
Using OrioleDB is dead simple - just add the
USING
keyword when creating tables.
Currently, OrioleDB is a storage engine extension requiring a patched PG kernel, as some necessary storage engine APIs haven’t landed in PG core yet. If all goes well, PostgreSQL 18 will include these patches, eliminating the need for kernel modifications.
Name | Link | Version | |
---|---|---|---|
✅ | Add missing inequality searches to rbtree | Link | PostgreSQL 16 |
✅ | Document the ability to specify TableAM for pgbench | Link | PostgreSQL 16 |
✅ | Remove Tuplesortstate.copytup function | Link | PostgreSQL 16 |
✅ | Add new Tuplesortstate.removeabbrev function | Link | PostgreSQL 16 |
✅ | Put abbreviation logic into puttuple_common() | Link | PostgreSQL 16 |
✅ | Move memory management away from writetup() and tuplesort_put*() | Link | PostgreSQL 16 |
✅ | Split TuplesortPublic from Tuplesortstate | Link | PostgreSQL 16 |
✅ | Split tuplesortvariants.c from tuplesort.c | Link | PostgreSQL 16 |
✅ | Fix typo in comment for writetuple() function | Link | PostgreSQL 16 |
✅ | Support for custom slots in the custom executor nodes | Link | PostgreSQL 16 |
✉️ | Allow table AM to store complex data structures in rd_amcache | Link | PostgreSQL 18 |
✉️ | Allow table AM tuple_insert() method to return the different slot | Link | PostgreSQL 18 |
✉️ | Add TupleTableSlotOps.is_current_xact_tuple() method | Link | PostgreSQL 18 |
✉️ | Allow locking updated tuples in tuple_update() and tuple_delete() | Link | PostgreSQL 18 |
✉️ | Add EvalPlanQual delete returning isolation test | Link | PostgreSQL 18 |
✉️ | Generalize relation analyze in table AM interface | Link | PostgreSQL 18 |
✉️ | Custom reloptions for table AM | Link | PostgreSQL 18 |
✉️ | Let table AM insertion methods control index insertion | Link | PostgreSQL 18 |
I’ve prepared oriolepg_17
(patched PG) and orioledb_17
(extension) on EL, plus a ready-to-use config template for instant OrioleDB deployment.
Cloud-Native Storage
“Cloud-native” is an overused term that nobody quite understands. But for databases, it usually means one thing: storing data in object storage.
OrioleDB recently pivoted their slogan from “High-performance OLTP storage engine” to “Cloud-native storage engine”. I get why - Supabase acquired OrioleDB, and the sugar daddy’s needs come first.
As a “cloud database provider”, offloading cold data to “cheap” object storage instead of “premium” EBS block storage is quite profitable. Plus, it makes databases stateless “cattle” that can be freely scaled in K8s. Their motivation is crystal clear.
So I’m pretty excited that OrioleDB not only offers a new storage engine but also supports object storage. While PG-over-S3 projects exist, this is the first mature, mainline-compatible, open-source solution.
So, How Do I Try It?
OrioleDB sounds great - solving key PG issues, (future) mainline compatibility, open-source, well-funded, and led by Alexander Korotkov who has serious PG community cred.
Obviously, OrioleDB isn’t “production-ready” yet. I’ve watched it from Alpha1 three years ago to Beta10 now, each release making me more antsy. But I noticed it’s now in Supabase’s postgres mainline - release can’t be far off.
So when OrioleDB dropped beta10 on April 1st, I decided to package it. Fresh off building OpenHalo RPMs and a MySQL-compatible PG kernel, what’s one more? I created RPM packages for the patched PG kernel (oriolepg_17) and extension (orioledb_17), available for EL8/EL9 on x86/ARM64.
Better yet, I added native OrioleDB support to Pigsty, meaning OrioleDB gets the full PG ecosystem - Patroni for HA, pgBackRest for backups, pg_exporter for monitoring, pgbouncer for connection pooling, all wrapped up in a one-click production-grade RDS service:
This Qingming Festival, I released Pigsty v3.4.1 with built-in OrioleDB and OpenHalo kernel support. Spinning up an OrioleDB cluster is as simple as a regular PostgreSQL cluster:
all:
children:
pg-orio:
vars:
pg_databases:
- {name: meta ,extensions: [orioledb]}
vars:
pg_mode: oriole
pg_version: 17
pg_packages: [ orioledb, pgsql-common ]
pg_libs: 'orioledb.so, pg_stat_statements, auto_explain'
repo_extra_packages: [ orioledb ]
More Kernel Tricks
Of course, OrioleDB isn’t the only PG fork we support. You can also use:
- Microsoft SQL Server-compatible Babelfish (by AWS)
- Oracle-compatible IvorySQL (by HighGo)
- MySQL-compatible openHalo (by EsgynDB)
- Aurora RAC-flavored PolarDB (by Alibaba Cloud)
- Officially certified Oracle-compatible PolarDB O 2.0
- FerretDB + Microsoft’s DocumentDB to emulate MongoDB
- One-click local Supabase (OrioleDB’s parent!) deployment using Pigsty templates
Plus, my friend Yurii, Omnigres founder, is adding ETCD protocol support to PostgreSQL. Soon, you might be able to use PG as a better-performing, more reliable etcd for Kubernetes/Patroni.
Best of all, everything’s open-source and ready to roll in Pigsty, free of charge. So if you’re curious about OrioleDB, grab a server and give it a shot - 10-minute setup, one command. Let’s see if it lives up to the hype.
OpenHalo: PostgreSQL Now Speaks MySQL Wire Protocol!
PostgreSQL speaking MySQL? Yes, you read that right. OpenHalo, freshly open-sourced on April Fools’ Day, brings this capability to life - allowing users to read, write, and manage the same database using both MySQL and PostgreSQL clients. Built on PG 14.10, it provides MySQL 5.7 wire protocol compatibility.
OpenHalo just open-sourced their MySQL-compatible PG kernel. I’ve packaged it into RPMs and integrated it into Pigsty. The deployment is butter-smooth, and after a few code tweaks, it plays nicely with HA, monitoring, and backup components.
On the DB-Engines ranking, five databases stand head and shoulders above the rest: Oracle, SQL Server, MySQL, PostgreSQL, and MongoDB.
Here’s the kicker - PostgreSQL can now emulate all four of its top competitors:
- OpenHalo speaks MySQL
- AWS Babelfish speaks SQL Server
- IvorySQL and Alibaba PolarDB O speak Oracle
- FerretDB/Microsoft DocumentDB speaks MongoDB
Fun fact: All these capabilities are available out-of-the-box in Pigsty.
Want to Try It Out?
Pigsty now supports OpenHalo on EL systems. Here’s how to get started:
Follow the Pigsty standard installation process with the mysql
config template:
curl -fsSL https://repo.pigsty.cc/get | bash; cd ~/pigsty
./bootstrap # Prepare dependencies
./configure -c mysql # Use MySQL (OpenHalo) template
./install.yml # Install (modify passwords in pigsty.yml for prod)
Pro tip: For production deployments, modify the passwords in pigsty.yml
before running the installation playbook.
OpenHalo’s configuration mirrors PostgreSQL’s. You can use psql
to connect to the postgres
database and mysql
CLI to connect to the mysql
database.
all:
children:
pg-orio:
vars:
pg_databases:
- {name: postgres ,extensions: [aux_mysql]}
vars:
pg_mode: mysql # MySQL Compatible Mode by HaloDB
pg_version: 14 # The current HaloDB is compatible with PG Major Version 14
pg_packages: [ openhalodb, pgsql-common, mysql ] # also install mysql client shell
repo_modules: node,pgsql,infra,mysql
repo_extra_packages: [ openhalodb, mysql ] # replace default postgresql kernel with openhalo packages
MySQL’s default port is 3306, and when accessing MySQL, you’re actually connecting to the postgres
database. Note that MySQL’s “database” concept maps to PostgreSQL’s “schema”. So use mysql
actually uses the mysql
schema in the postgres
database.
MySQL credentials mirror PostgreSQL’s - manage users and permissions the PostgreSQL way. Currently, OpenHalo officially supports Navicat, though IntelliJ IDEA’s DataGrip might throw some tantrums.
mysql -h 127.0.0.1 -u dbuser_dba
Pigsty’s OpenHalo fork includes some QoL improvements over HaloTech-Co-Ltd/openHalo:
- Default database renamed from
halo0root
topostgres
- Version number simplified from
1.0.14.10
to14.10
- MySQL compatibility and port 3306 enabled by default
Disclaimer: Pigsty provides no warranty for OpenHalo - direct support queries to the original vendor.
More Kernel Tricks Up Our Sleeve
OpenHalo isn’t the only PG fork Pigsty supports. Check out:
- Babelfish by AWS (SQL Server compatibility)
- IvorySQL by Highgo (Oracle compatibility)
- OrioleDB by Supabase (OLTP performance beast)
- PolarDB by Alibaba Cloud (Aurora RAC flavor)
- PolarDB O 2.0 (Oracle-compatible with Chinese certification)
- FerretDB + Microsoft’s DocumentDB (MongoDB emulation)
- One-click Supabase local deployment (OrioleDB’s parent!)
BTW, my friend Yurii, Omnigres founder, is working on ETCD protocol support for PostgreSQL. Soon you might be able to use PG as a beefed-up etcd for Kubernetes/Patroni.
Best part? All this goodness is open-source and ready to roll in Pigsty. Want to take OpenHaloDB for a spin? Grab a server, and you’re 10 minutes away from finding out if it lives up to the hype.
PGFS: Using PostgreSQL as a File System
Recently, I received an interesting request from the Odoo community. They were grappling with a fascinating challenge: “If databases can do PITR (Point-in-Time Recovery), is there a way to roll back the file system as well?”
The Birth of “PGFS”
From a database veteran’s perspective, this is both challenging and exciting. We all know that in systems like Odoo, the most valuable asset is the core business data stored in PostgreSQL.
However, many “enterprise applications” also deal with file operations — attachments, images, documents, and the like. While these files might not be as “mission-critical” as the database, having the ability to roll back both the database and files to a specific point in time would be incredibly valuable from security, data integrity, and operational perspectives.
This led me to an intriguing thought: Could we give file systems PITR capabilities similar to databases? Traditional approaches often point to expensive and complex CDP (Continuous Data Protection) solutions, requiring specialized hardware or block-level storage logging. But I wondered: Could we solve this elegantly with open-source technology for the “rest of us”?
After much contemplation, a brilliant combination emerged: JuiceFS + PostgreSQL. By transforming PostgreSQL into a file system, all file writes would be stored in the database, sharing the same WAL logs and enabling rollback to any historical point. This might sound like science fiction, but hold on — it actually works. Let’s see how JuiceFS makes this possible.
Meet JuiceFS: When Database Becomes a File System
JuiceFS is a high-performance, cloud-native distributed file system that can mount object storage (like S3/MinIO) as a local POSIX file system. It’s incredibly lightweight to install and use, requiring just a few commands to format, mount, and start reading/writing.
For example, these commands will use SQLite as JuiceFS’s metadata store and a local path as object storage for testing:
juicefs format sqlite3:///tmp/jfs.db myjfs # Use SQLite3 for metadata, local FS for data
juicefs mount sqlite3:///tmp/jfs.db ~/jfs -d # Mount the filesystem to ~/jfs
The magic happens when you realize that JuiceFS also supports PostgreSQL as both metadata and object data storage backend! This means you can transform any PostgreSQL instance into a “file system” by simply changing JuiceFS’s backend.
So, if you have a PostgreSQL database (like one installed via Pigsty), you can spin up a “PGFS” with just a few commands:
METAURL=postgres://dbuser_meta:DBUser.Meta@:5432/meta
OPTIONS=(
--storage postgres
--bucket :5432/meta
--access-key dbuser_meta
--secret-key DBUser.Meta
${METAURL}
jfs
)
juicefs format "${OPTIONS[@]}" # Create a PG filesystem
juicefs mount ${METAURL} /data2 -d # Mount in background to /data2
juicefs bench /data2 # Test performance
juicefs umount /data2 # Unmount
Now, any data written to /data2
is actually stored in the jfs_blob
table in PostgreSQL. In other words, this file system and PG database have become one!
PGFS in Action: File System PITR
Imagine we have an Odoo instance that needs to store file data in /var/lib/odoo
or similar.
Traditionally, if you needed to roll back Odoo’s database, while the database could use WAL logs for point-in-time recovery, the file system would still rely on external snapshots or CDP.
But now, if we mount /var/lib/odoo
to PGFS, all file system writes become database writes.
The database is no longer just storing SQL data; it’s also hosting the file system information.
This means: When performing PITR, not only does the database roll back to a specific point, but files instantly “roll back” with the database to the same moment.
Some might ask, “Can’t ZFS do snapshots too?” Yes, ZFS can create and roll back snapshots, but that’s still based on specific snapshot points. For precise rollback to a specific second or minute, you need true log-based solutions or CDP capabilities. The JuiceFS+PG combination effectively writes file operation logs into the database’s WAL, which is something PostgreSQL is naturally great at.
Let’s demonstrate this with a simple experiment. First, we’ll write timestamps to the file system while continuously inserting heartbeat records into the database:
while true; do date "+%H-%M-%S" >> /data2/ts.log; sleep 1; done
/pg/bin/pg-heartbeat # Generate database heartbeat records
tail -f /data2/ts.log
Then, let’s verify the JuiceFS table in PostgreSQL:
postgres@meta:5432/meta=# SELECT min(modified),max(modified) FROM jfs_blob;
min | max
----------------------------+----------------------------
2025-03-21 02:26:00.322397 | 2025-03-21 02:40:45.688779
When we decide to roll back to, say, one minute ago (2025-03-21 02:39:00
), we just execute:
pg-pitr --time="2025-03-21 02:39:00" # Using pgbackrest to roll back to specific time, actual command:
pgbackrest --stanza=pg-meta --type=time --target='2025-03-21 02:39:00+00' restore
What? You’re asking where PITR and pgBackRest came from? Pigsty has already configured monitoring, backup, high availability, and more out of the box! You can set it up manually too, but it’s a bit more work.
Then when we check the file system logs and database heartbeat table, both have stopped at 02:39:00:
$ tail -n1 /data2/ts.log
02-38-59
$ psql -c 'select * from monitor.heartbeat'
id | ts | lsn | txid
---------+-------------------------------+-----------+------
pg-meta | 2025-03-21 02:38:59.129603+00 | 251871544 | 2546
This proves our approach works! We’ve successfully achieved FS/DB consistent PITR through PGFS!
How’s the Performance?
So we’ve got the functionality, but how does it perform?
I ran some tests on a development server with SSD using the built-in juicefs bench
, and the results look promising — more than enough for applications like Odoo:
$ juicefs bench ~/jfs # Simple single-threaded performance test
BlockSize: 1.0 MiB, BigFileSize: 1.0 GiB,
SmallFileSize: 128 KiB, SmallFileCount: 100, NumThreads: 1
Time used: 42.2 s, CPU: 687.2%, Memory: 179.4 MiB
+------------------+------------------+---------------+
| ITEM | VALUE | COST |
+------------------+------------------+---------------+
| Write big file | 178.51 MiB/s | 5.74 s/file |
| Read big file | 31.69 MiB/s | 32.31 s/file |
| Write small file | 149.4 files/s | 6.70 ms/file |
| Read small file | 545.2 files/s | 1.83 ms/file |
| Stat file | 1749.7 files/s | 0.57 ms/file |
| FUSE operation | 17869 operations | 3.82 ms/op |
| Update meta | 1164 operations | 1.09 ms/op |
| Put object | 356 operations | 303.01 ms/op |
| Get object | 256 operations | 1072.82 ms/op |
| Delete object | 0 operations | 0.00 ms/op |
| Write into cache | 356 operations | 2.18 ms/op |
| Read from cache | 100 operations | 0.11 ms/op |
+------------------+------------------+---------------+
Another sample: Alibaba Cloud ESSD PL1 basic disk test results
+------------------+------------------+---------------+
| ITEM | VALUE | COST |
+------------------+------------------+---------------+
| Write big file | 18.08 MiB/s | 56.64 s/file |
| Read big file | 98.07 MiB/s | 10.44 s/file |
| Write small file | 268.1 files/s | 3.73 ms/file |
| Read small file | 1654.3 files/s | 0.60 ms/file |
| Stat file | 7465.7 files/s | 0.13 ms/file |
| FUSE operation | 17855 operations | 4.28 ms/op |
| Update meta | 1192 operations | 16.28 ms/op |
| Put object | 357 operations | 2845.34 ms/op |
| Get object | 255 operations | 327.37 ms/op |
| Delete object | 0 operations | 0.00 ms/op |
| Write into cache | 357 operations | 2.05 ms/op |
| Read from cache | 102 operations | 0.18 ms/op |
+------------------+------------------+---------------+
While the throughput might not match native file systems, it’s more than sufficient for applications with moderate file volumes and lower access frequencies. After all, using a “database as a file system” isn’t about running large-scale storage or high-concurrency writes — it’s about keeping your database and file system “in sync through time.” If it works, it works.
Completing the Vision: One-Click “Enterprise” Deployment
Now, let’s put this all together in a practical scenario — like one-click deploying an “enterprise-grade” Odoo with “automatic” CDP capabilities for files.
Pigsty provides PostgreSQL with external high availability, automatic backup, monitoring, PITR, and more. Installing it is a breeze:
curl -fsSL https://repo.pigsty.cc/get | bash; cd ~/pigsty
./bootstrap # Install Pigsty dependencies
./configure -c app/odoo # Use Odoo configuration template
./install.yml # Install Pigsty
That’s the standard Pigsty installation process. Next, we’ll use playbooks to install Docker, create the PGFS mount, and launch stateless Odoo with Docker Compose:
./docker.yml -l odoo # Install Docker module, launch Odoo stateless components
./juice.yml -l odoo # Install JuiceFS module, mount PGFS to /data2
./app.yml -l odoo # Launch Odoo stateless components, using external PG/PGFS
Yes, it’s that simple. Everything is ready, though the key lies in the configuration file.
The pigsty.yml
configuration file would look something like this, with the only modification being the addition of JuiceFS configuration to mount PGFS to /data/odoo
:
odoo:
hosts: { 10.10.10.10: {} }
vars:
# ./juice.yml -l odoo
juice_fsname: jfs
juice_mountpoint: /data/odoo
juice_options:
- --storage postgres
- --bucket :5432/meta
- --access-key dbuser_meta
- --secret-key DBUser.Meta
- postgres://dbuser_meta:DBUser.Meta@:5432/meta
- ${juice_fsname}
# ./app.yml -l odoo
app: odoo # specify app name to be installed (in the apps)
apps: # define all applications
odoo: # app name, should have corresponding ~/app/odoo folder
file: # optional directory to be created
- { path: /data/odoo ,state: directory, owner: 100, group: 101 }
- { path: /data/odoo/webdata ,state: directory, owner: 100, group: 101 }
- { path: /data/odoo/addons ,state: directory, owner: 100, group: 101 }
conf: # override /opt/<app>/.env config file
PG_HOST: 10.10.10.10 # postgres host
PG_PORT: 5432 # postgres port
PG_USERNAME: odoo # postgres user
PG_PASSWORD: DBUser.Odoo # postgres password
ODOO_PORT: 8069 # odoo app port
ODOO_DATA: /data/odoo/webdata # odoo webdata
ODOO_ADDONS: /data/odoo/addons # odoo plugins
ODOO_DBNAME: odoo # odoo database name
ODOO_VERSION: 18.0 # odoo image version
After this, you have an “enterprise-grade” Odoo running on the same server: backend database managed by Pigsty, file system mounted via JuiceFS, with JuiceFS’s backend connected to PostgreSQL. When a “rollback need” arises, simply perform PITR on PostgreSQL, and both files and database will “roll back to the specified moment.” This approach works equally well for similar applications like Dify, Gitlab, Gitea, MatterMost, and others.
Looking back at all this, you’ll realize: What once required expensive hardware and high-end storage solutions to achieve CDP can now be accomplished with a lightweight open-source combination. While it might have a “DIY for the rest of us” feel, it’s simple, stable, and practical enough to be worth exploring in more scenarios.
PostgreSQL Frontier
Dear readers, I’m off on vacation starting today—likely no new posts for about two weeks. Let me wish everyone a Happy New Year in advance.
Of course, before heading out, I wanted to share some recent interesting developments in the Postgres (PG) ecosystem. Just yesterday, I hurried to release Pigsty 3.2.2 and Pig v0.1.3 before my break. In this new version, the number of available PG extensions has shot up from 350 to 400, bundling many fascinating toys. Below is a quick rundown:
Omnigres: Full-stack web development inside PostgreSQL
PG Mooncake: Bringing ClickHouse-level analytical performance into PG
Citus: Distributed extension for PG17—Citus 13 is out!
FerretDB: MongoDB “compatibility” layer on PG with 20x performance boost in 2.0
ParadeDB: ES-like full-text search in PG with PG block storage
Pigsty 3.2.2: Packs all of the above into one box for immediate use
Omnigres
I introduced Omnigres in a previous article, “Database as Architecture”. In short, it allows you to cram all your business logic—including a web server and the entire backend—into PostgreSQL.
For example, the following SQL will launch a web server and expose /www
as the root directory. This means you can package what’s normally a classic three-tier architecture (frontend–backend–database) entirely into a single database!
If you’re familiar with Oracle, you might notice it’s somewhat reminiscent of Oracle Apex. But in PostgreSQL, you have over twenty different languages to develop your stored procedures in—not just PL/SQL! Plus, Omnigres gives you far more than just an HTTPD server; it actually ships 33 extension plugins that function almost like a “standard library” for web development within PG.
They say, “what’s split apart will eventually recombine, what’s recombined will eventually split apart.” In ancient times, many C/S or B/S applications were basically a few clients directly reading/writing to the database. Later, as business logic grew more complex and hardware (relative to business needs) got stretched, we peeled away a lot of functionality from the database, forming the traditional three-tier model.
Now, with significant improvements in hardware performance—giving us surplus capacity on database servers—and with easier ways to write stored procedures, this “split” trend may well reverse. Business logic once stripped out of the database might come back in. I see Omnigres (and Supabase, too) as a renewed attempt at “recombination.”
If you’re running tens of thousands of TPS, dealing with tens of terabytes of data, or handling a life-critical mega-sized core system, this might not be your best approach. But if you’re developing personal projects, small websites, or an early-stage startup with an innovative, smaller-scale system, this architecture can dramatically speed up your iteration cycle, simplifying both development and operations.
Pigsty v3.2.2 comes with the Omnigres extension included—this took quite some effort. With hands-on help from the original author, Yurii, we managed to build and package it for 10 major Linux distributions. Note that these extensions come from an independent repo you can use on its own—you’re not required to run Pigsty just to get them. (Omnigres and AutoBase PG both rely on this repo for extension distribution, a terrific example of open-source ecosystems thriving on mutual benefit.)
pg_mooncake
Ever since the “DuckDB Mash-up Contest” kicked off, pg_mooncake was the last entrant. At one point, I almost thought they had gone dormant. But last week, they dropped a bombshell with their new 0.1.0 release, catapulting themselves directly into the top 10 on the ClickBench leaderboard, right alongside ClickHouse.
This is the first time a PostgreSQL setup—plus an extension—has broken into that Tier 0 bracket on an analytical leaderboard. It’s a milestone worth noting. Looks like pg_duckdb just met a fierce contender—and that’s good news for everyone, since we now have multiple ways to do high-performance analytics in PG. Internal competition keeps the ecosystem thriving, and it also widens the gap between the entire Postgres ecosystem and other DBMSs.
Most people still see PostgreSQL as a rock-solid OLTP database, rarely associating it with “real-time analytics.” Yet PostgreSQL’s extensibility allows it to transcend that image and carve out new territory in real-time analytics. The pg_mooncake team leveraged PG’s extensibility to write a native extension that embeds DuckDB’s query engine for columnar queries. This means queries can process data in batches (instead of row-by-row) and utilize SIMD instructions, yielding significant speedups in scanning, grouping, and aggregation.
pg_mooncake also employs a more efficient metadata mechanism: instead of fetching metadata and statistics externally from Parquet or some other storage, it stores them directly in PostgreSQL. This speeds up query optimization and execution, and enables higher-level features such as file-level skipping to accelerate scans.
All these optimizations have yielded impressive performance results—reportedly up to 1000x faster. That means PostgreSQL is no longer just a “heavy-duty workhorse” for OLTP. With the right engineering and optimization, it can go head-to-head with specialized analytical databases while retaining PG’s hallmark flexibility and vast ecosystem. This could simplify the entire data stack—no more complicated big-data toolkits or ETL pipelines. Top-tier analytics can happen directly inside Postgres.
Pigsty v3.2.2 now officially includes the mooncake 0.1 binary. Note that this extension conflicts with pg_duckdb since both bundle their own libduckdb
. You can only choose one of them on a given system. That’s a bit of a pity—I filed an issue suggesting they share a single libduckdb
. It’s exhausting that each extension builds DuckDB from scratch, especially when you’re compiling them both.
Finally, you can tell from the name “mooncake” that it’s led by a Chinese-speaking team. It’s awesome to see more people from China contributing and standing out in the Postgres ecosystem.
Blog: ClickBench says “Postgres is a great analytics database” https://www.mooncake.dev/blog/clickbench-v0.1
ParadeDB
ParadeDB is an old friend of Pigsty. We’ve supported ParadeDB from its very early days and watched it grow into the leading solution in the PostgreSQL ecosystem to provide an ElasticSearch-like capability.
pg_search
is ParadeDB’s extension for Postgres, implementing a custom index that supports full-text search and analytics. It’s powered underneath by a Rust-based search library Tantivy, inspired by Lucene.
pg_search just released version 0.14 in the past two weeks, switching to PG’s native block storage instead of relying on Tantivy’s own file format. This is a huge architectural shift that dramatically boosts reliability and yields multiple times the performance. It’s no longer just some “stitch-it-together hack”—it’s now deeply embedded into PG.
Prior to v0.14.0
, pg_search
did not use Postgres’s block storage or buffer cache. The extension managed its own files outside Postgres control, reading them directly from disk. While it’s not unusual for an extension to access the file system directly (see note 1), migrating to block storage delivers:
- Deep integration with Postgres WAL (write-ahead logging), enabling physical replication of indexes.
- Support for crash recovery and point-in-time recovery (PITR).
- Full support for Postgres MVCC (multi-version concurrency control).
- Integration with Postgres’s buffer cache, significantly boosting index build speed and write throughput.
The latest version of pg_search is now included in Pigsty. Of course, we also bundle other full-text search / tokenizing extensions like pgroonga
, pg_bestmatch
, hunspell
, and Chinese tokenizer zhparser
, so you can pick the best fit.
Blog: Full-text search with Postgres block storage layout https://www.paradedb.com/blog/block_storage_part_one
citus
While pg_duckdb and pg_mooncake represent the new wave of OLAP in the PG ecosystem, Citus (and Hydra) are more old-school OLAP— or perhaps HTAP—extensions. Just the day before yesterday, Citus 13.0.0 was released, officially supporting the latest PostgreSQL version 17. That means all the major extensions now have PG17-compatible releases. Full speed ahead for PG17!
Citus is a distributed extension for PG, letting you seamlessly turn a single Postgres primary–replica deployment into a horizontally scaled cluster. Microsoft acquired Citus and fully open-sourced it; the cloud version is called Hyperscale PG or CosmosDB PG.
In reality, most users nowadays don’t push the hardware to the point that they absolutely need a distributed database—but such scenarios do exist. For instance, in “Escaping from cloud fraude” (an article about someone trying to escape steep cloud costs), the user ended up considering Citus to offset expensive cloud disk usage. So, Pigsty has also updated and included full Citus support.
Typically, a distributed database is more of a headache to administer than a simple primary–replica setup. But we devised an elegant abstraction so deploying and managing Citus is pretty straightforward—just treat them as multiple horizontal PG clusters. A single configuration file can spin up a 10-node Citus cluster with one command.
I recently wrote a tutorial on how to deploy a highly available Citus cluster. Feel free to check it out: https://pigsty.cc/docs/tasks/citus/
Blog: Release notes for Citus v13.0.0: https://github.com/citusdata/citus/blob/v13.0.0/CHANGELOG.md
FerretDB
Finally, we have FerretDB 2.0. FerretDB is another old friend of Pigsty. Marcin reached out to me right away to share the excitement of the new release. Unfortunately, 2.0 is still in RC, so I couldn’t package it into the Pigsty repo in time for the v3.2.2 release. No worries—it’ll be included next time!
FerretDB turns PostgreSQL into a “wire-protocol compatible” MongoDB. It’s licensed under Apache 2.0—truly open source. FerretDB 2.0 leverages Microsoft’s newly open-sourced DocumentDB PostgreSQL extension, delivering major improvements in performance, compatibility, support, and flexibility. Highlights include:
- Over 20x performance boost
- Greater feature parity
- Vector search support
- Replication support
- Broad community backing and services
FerretDB offers a low-friction path for MongoDB users to migrate to PostgreSQL. You don’t need to touch your application code—just swap out the back end and voilà. You get the MongoDB API compatibility plus the superpowers of the entire PG ecosystem, which offers hundreds of extensions.
Blog: https://blog.ferretdb.io/ferretdb-releases-v2-faster-more-compatible-mongodb-alternative/
Pigsty 3.2.2
And that brings us to Pigsty v3.2.2. This release adds 40 brand-new extensions (33 of which come from Omnigres) and updates many existing ones (Citus, ParadeDB, PGML, etc.). We also contributed to and followed up on PolarDB PG’s ARM64 support, as well as support for Debian systems, and tracked IvorySQL’s latest 4.2 release compatible with PostgreSQL 17.2.
Sure, it may sound like a bunch of version sync chores, but if it weren’t for those chores, I wouldn’t have dropped this release a day before my vacation! Anyway, I hope you’ll give these new extensions a try. If you run into any issues, feel free to let me know—just understand I can’t guarantee a quick response while I’m off.
One more thing: some users told me the old Pigsty website was “ugly”—basically overflowing with “tech-bro aesthetic,” cramming all the info into a single dense page. They have a point, so I recently used a front-end template to give the homepage a fresh coat of paint. Now it looks a bit more “international.”
To be honest, I haven’t touched front-end in seven or eight years. Last time, it was a jQuery-fest. This time around, Next.js / Vercel / all the new stuff had me dazzled. But once I got my bearings (and thanks to GPT o1 pro plus Cursor), it all came together in a day. The productivity gains with AI these days are truly astounding.
Alright, that’s the latest news from the PostgreSQL world. I’m about to pack my bags—my flight to Thailand departs this afternoon, fingers crossed I don’t run into any phone-scam rings. Let me wish everyone a Happy New Year in advance!
Pig, The PostgreSQL Extension Wizard
Ever wished installing or upgrading PostgreSQL extensions didn’t feel like digging through outdated readmes, cryptic configure scripts, or random GitHub forks & patches? The painful truth is that Postgres’s richness of extension often comes at the cost of complicated setups—especially if you’re juggling multiple distros or CPU architectures.
Enter Pig, a Go-based package manager built to tame Postgres and its ecosystem of 400+ extensions in one fell swoop. TimescaleDB, Citus, PGVector, 20+ Rust extensions, plus every must-have piece to self-host Supabase — Pig’s unified CLI makes them all effortlessly accessible. It cuts out messy source builds and half-baked repos, offering version-aligned RPM/DEB packages that work seamlessly across Debian, Ubuntu, and RedHat flavors. No guesswork, no drama.
Instead of reinventing the wheel, Pig piggyback your system’s native package manager (APT, YUM, DNF) and follow official PGDG packaging conventions to ensure a glitch-free fit. That means you don’t have to choose between “the right way” and “the quick way”; Pig respects your existing repos, aligns with standard OS best practices, and fits neatly alongside other packages you already use.
Ready to give your Postgres superpowers without the usual hassle? Check out GitHub for documentation, installation steps, and a peek at its massive extension list. Then, watch your local Postgres instance transform into a powerhouse of specialized modules—no black magic is required. If the future of Postgres is unstoppable extensibility, Pig is the genie that helps you unlock it. Honestly, nobody ever complained that they had too many extensions.
PIG v0.1 Release | GitHub Repo | Blog: The Idea Way to deliver PG Extensions
Get Started
Install the pig
package itself with scripts or the traditional yum/apt way.
curl -fsSL https://repo.pigsty.io/pig | bash
Then it’s ready to use; assume you want to install the pg_duckdb
extension:
$ pig repo add pigsty pgdg -u # add pgdg & pigsty repo, update cache
$ pig repo set -u # overwrite all existing repos, brute but effective
$ pig ext install pg17 # install native PGDG PostgreSQL 17 kernels packages
$ pig ext install pg_duckdb # install the pg_duckdb extension (for current pg17)
Extension Management
pig ext list [query] # list & search extension
pig ext info [ext...] # get information of a specific extension
pig ext status [-v] # show installed extension and pg status
pig ext add [ext...] # install extension for current pg version
pig ext rm [ext...] # remove extension for current pg version
pig ext update [ext...] # update extension to the latest version
pig ext import [ext...] # download extension to local repo
pig ext link [ext...] # link postgres installation to path
pig ext build [ext...] # setup building env for extension
Repo Management
pig repo list # available repo list (info)
pig repo info [repo|module...] # show repo info (info)
pig repo status # show current repo status (info)
pig repo add [repo|module...] # add repo and modules (root)
pig repo rm [repo|module...] # remove repo & modules (root)
pig repo update # update repo pkg cache (root)
pig repo create # create repo on current system (root)
pig repo boot # boot repo from offline package (root)
pig repo cache # cache repo as offline package (root)
Don't Update! Rollback Issued on Release Day: PostgreSQL Faces a Major Setback
As the old saying goes, never release code on Friday. Although PostgreSQL’s recent minor release carefully avoided a Friday launch, it still gave the community a full week of extra work — PostgreSQL will release an unscheduled emergency update next Thursday: PostgreSQL 17.2, 16.6, 15.10, 14.15, 13.20, and even 12.22 for the just-EOLed PG 12.
This is the first time in a decade that such a situation has occurred: on the very day of PostgreSQL’s release, the new version was pulled due to issues discovered by the community. There are two reasons for this emergency release. First, to fix the CVE-2024-10978 security vulnerability, which isn’t a major concern. The real problem is that the new PostgreSQL minor version modified its ABI, causing extensions that depend on ABI stability — like TimescaleDB — to crash.
The issue of PostgreSQL minor version ABI compatibility was actually raised by Yuri back in June at PGConf 2024. During the extensions summit and his talk “Pushing boundaries with extensions, for extension”, he brought up this concern, but it didn’t receive much attention. Now it has exploded spectacularly, and I imagine Yuri is probably shrugging his shoulders saying: “Told you so.”
In short, the PostgreSQL community strongly recommends that users do not upgrade PostgreSQL in the coming week. Tom Lane has proposed releasing an unscheduled emergency minor version next Thursday to roll back these changes, overwriting the older 17.1, 16.5, and so on — essentially treating the problematic versions as if they “never existed.” Consequently, Pigsty 3.1, which was scheduled for release in the next couple of days and set to use the latest PostgreSQL 17.1 by default, will also be delayed by a week.
Overall, I believe this incident will have a positive impact. First, it’s not a quality issue with the core kernel itself. Second, because it was discovered early enough — on the very day of release — and promptly halted, there was no substantial impact on users. Unlike vulnerabilities in other databases/chips/operating systems that cause widespread damage upon discovery, this was caught early. Apart from a few overzealous update enthusiasts or unfortunate new installations, there shouldn’t be much impact. This is similar to the recent xz backdoor incident, which was also discovered by PG core developer Peter during PostgreSQL testing, further highlighting the vitality and insight of the PostgreSQL ecosystem.
What Happened
On the morning of November 14th, an email appeared on the PostgreSQL Hackers mailing list mentioning that the new minor version had actually broken the ABI. This isn’t a problem for the PostgreSQL database kernel itself, but the ABI change broke the convention between the PG kernel and extension plugins, causing extensions like TimescaleDB to fail on the new PG minor version.
PostgreSQL extension plugins are provided for specific major versions on specific operating system distributions. For example, PostGIS, TimescaleDB, and Citus are built for major versions like PG 12, 13, 14, 15, 16, and 17 released each year. Extensions built for PG 16.0 are generally expected to continue working on PG 16.1, 16.2, … 16.x. This means you can perform rolling upgrades of the PG kernel’s minor versions without worrying about extension plugin issues.
However, this isn’t an explicit promise but rather an implicit community understanding — ABI belongs to internal implementation details and shouldn’t have such promises or expectations. PostgreSQL has simply performed too well in the past, and everyone has grown accustomed to this behavior, making it a default working assumption reflected in various aspects including PGDG repository package naming and installation scripts.
This time, though, PG 17.1 and the backported versions to 16-12 modified the size of an internal structure, which can cause — extensions compiled for PG 17.0 when used on 17.1 — potential conflicts resulting in illegal writes or program crashes. Note that this issue doesn’t affect users of the PostgreSQL kernel itself; PostgreSQL has internal assertions to check for such situations.
However, for users of extensions like TimescaleDB, this means if you don’t use extensions recompiled for the current minor version, you’ll face such security risks. Given the current maintenance logic of PGDG repositories, extension plugins are only compiled against the latest PG minor version when a new extension version is released.
Regarding the PostgreSQL ABI issue, Marco Slot from CrunchyData wrote a detailed thread explaining it. Available for professional readers to reference.
https://x.com/marcoslot/status/1857403646134153438
How to Avoid Such Problems
As I mentioned previously in “PG’s Ultimate Achievement: The Most Complete PG Extension Repository”, I maintain a repository of many PG extension plugins for EL and Debian/Ubuntu, covering nearly half of the extensions in the entire PG ecosystem.
The PostgreSQL ABI issue was actually mentioned by Yuri before. As long as your extension plugins are compiled for the PostgreSQL minor version you’re currently using, there won’t be any problems. That’s why I recompile and package these extension plugins whenever a new minor version is released.
Last month, I had just finished compiling all the extension plugins for 17.0, and was about to start updates for compiling the 17.1 version. It looks like that won’t be necessary now, as 17.2 will roll back the ABI changes. While this means extensions compiled on 17.0 can continue to be used, I’ll still recompile and package against PG 17.2 and other main versions after 17.2 is released.
If you’re in the habit of installing PostgreSQL and extension plugins from the internet and don’t promptly upgrade minor versions, you’ll indeed face this security risk — where your newly installed extensions aren’t compiled for your older kernel version and crash due to ABI conflicts.
To be honest, I’ve encountered this problem in the real world quite early on, which is why when developing Pigsty, an out-of-the-box PostgreSQL distribution, I chose from Day 1 to first download all necessary packages and their dependencies locally, build a local software repository, and then provide Yum/Apt repositories to all nodes that need them. This approach ensures that all nodes in the environment install the same versions, and that it’s a consistent snapshot — the extension versions match the kernel version.
Moreover, this approach achieves the requirement of “independent control,” meaning that after your deployment goes live, you won’t encounter absurd situations like — the original software source shutting down or moving, or simply the upstream repository releasing an incompatible new version or new dependency, leading to major failures when setting up new machines/instances. This means you have a complete software copy for replication/expansion, with the ability to keep your services running indefinitely without worrying about someone “truly cutting off your lifeline.”
For example, when 17.1 was recently released, RedHat updated the default version of LLVM from 17 to 18 just two days prior, and unfortunately only updated EL8 without updating EL9. If users chose to install from the internet upstream at this time, it would fail directly. After I raised this issue to Devrim, he spent two hours fixing it by adding LLVM-18 to the EL9-specific patch Fix repository.
PS: If you didn’t know about this independent repository, you’d probably continue to encounter issues even after the fix, until RedHat fixed the problem themselves. But Pigsty would handle all these dirty details for you.
Some might say they could solve such version problems using Docker, which is certainly true. However, running databases in Docker comes with other issues, and these Docker images essentially use the operating system’s package manager in their Dockerfiles to download RPM/DEB packages from official repositories. Ultimately, someone has to do this work…
Of course, adapting to different operating systems means a significant maintenance workload. For example, I maintain 143 PG extension plugins for EL and 144 for Debian, each needing to be compiled for 10 major operating system versions (EL 8/9, Ubuntu 22/24, Debian 12, five major systems, amd64 and arm64) and 6 database major versions (PG 17-12). The combination of these elements means there are nearly 10,000 packages to build/test/distribute, including twenty Rust extensions that take half an hour to compile… But honestly, since it’s all semi-automated pipeline work, changing from running once a year to once every 3 months is acceptable.
Appendix: Explanation of the ABI Issue
About the PostgreSQL extension ABI issue in the latest patch versions (17.1, 16.5, etc.)
C code in PostgreSQL extensions includes headers from PostgreSQL itself. When an extension is compiled, functions from the headers are represented as abstract symbols in the binary. These symbols are linked to actual function implementations when the extension is loaded, based on function names. This way, an extension compiled for PostgreSQL 17.0 can typically still load into PostgreSQL 17.1, as long as function names and signatures in the headers haven’t changed (i.e., the Application Binary Interface or “ABI” is stable).
Headers also declare structures (passed as pointers) to functions. Strictly speaking, structure definitions are also part of the ABI, but there are more subtleties here. After compilation, structures are primarily defined by their size and field offsets, so name changes don’t affect the ABI (though they affect the API). Size changes slightly affect the ABI. In most cases, PostgreSQL uses a macro (“makeNode”) to allocate structures on the heap, which looks at the compile-time size of the structure and initializes the bytes to 0.
The difference in 17.1 is that a new boolean was added to the ResultRelInfo
structure, increasing its size. What happens next depends on who calls makeNode
. If it’s code from PostgreSQL 17.1, it uses the new size. If it’s an extension compiled for 17.0, it uses the old size. When it calls PostgreSQL functions with a pointer allocated using the old size, PostgreSQL functions still assume the new size and may write beyond the allocated block. Generally, this is quite problematic. It can lead to bytes being written to unrelated memory areas or program crashes.
When running tests, PostgreSQL has internal checks (assertions) to detect this situation and throw warnings. However, PostgreSQL uses its own allocator, which always rounds up allocated bytes to powers of 2. The ResultRelInfo
structure is 376 bytes (on my laptop), so it rounds up to 512 bytes, and similarly after the change (384 bytes on my laptop). Therefore, this particular structure change typically doesn’t affect allocation size. There might be uninitialized bytes, but this is usually resolved by calling InitResultRelInfo
.
This issue mainly raises warnings in tests or assertion-enabled builds where extensions allocate ResultRelInfo
, especially when running those tests with extension binaries compiled against older PostgreSQL versions. Unfortunately, the story doesn’t end there. TimescaleDB is a heavy user of ResultRelInfo
and indeed encountered problems with the size change. For example, in one of its code paths, it needs to find an index in an array of ResultRelInfo
pointers, for which it performs pointer arithmetic. This array is allocated by PostgreSQL (384 bytes), but the Timescale binary assumes 376 bytes, resulting in a meaningless number that triggers assertion failures or segfaults. https://github.com/timescale/timescaledb/blob/2.17.2/src/nodes/hypertable_modify.c#L1245…
The code here isn’t actually wrong, but the contract with PostgreSQL isn’t as expected. This is an interesting lesson for all of us. Similar issues might exist in other extensions, though not many extensions are as advanced as Timescale. Another advanced extension is Citus, but I’ve verified that Citus is safe. It does show assertion warnings. Everyone is advised to be cautious. The safest approach is to ensure extensions are compiled with headers from the PostgreSQL version you’re running.
PostgreSQL 12 Reaches End-of-Life as PG 17 Takes the Throne
According to PostgreSQL’s versioning policy, PostgreSQL 12, released in 2019, officially exits its support lifecycle today (November 14, 2024).
PostgreSQL 12’s final minor version, 12.21, released today (November 14, 2024), marks the end of the road for PG 12. Meanwhile, the newly released PostgreSQL 17.1 emerges as the ideal choice for new projects.
Version | Current minor | Supported | First Release | Final Release |
---|---|---|---|---|
17 | 17.1 | Yes | September 26, 2024 | November 8, 2029 |
16 | 16.5 | Yes | September 14, 2023 | November 9, 2028 |
15 | 15.9 | Yes | October 13, 2022 | November 11, 2027 |
14 | 14.14 | Yes | September 30, 2021 | November 12, 2026 |
13 | 13.17 | Yes | September 24, 2020 | November 13, 2025 |
12 | 12.21 | No | October 3, 2019 | November 14, 2024 |
Farewell to PG 12
Over the past five years, PostgreSQL 12.20 (the previous minor version) addressed 34 security issues and fixed 936 bugs compared to PostgreSQL 12.0 released five years ago.
This final release (12.21) patches four CVE security vulnerabilities and includes 17 bug fixes. From this point forward, PostgreSQL 12 enters retirement, with no further security or error fixes.
- CVE-2024-10976: PostgreSQL row security ignores user ID changes in certain contexts (e.g., subqueries)
- CVE-2024-10977: PostgreSQL libpq preserves error messages from man-in-the-middle attacks
- CVE-2024-10978: PostgreSQL SET ROLE, SET SESSION AUTHORIZATION resets to incorrect user ID
- CVE-2024-10979: PostgreSQL PL/Perl environment variable changes execute arbitrary code
The risks of running outdated versions will continue to increase over time. Users still running PG 12 or earlier versions in production should develop an upgrade plan to a supported major version (13-17).
PostgreSQL 12, released five years ago, was a milestone release in my view - the most significant since PG 10. Notably, PG 12 introduced pluggable storage engine interfaces, allowing third parties to develop new storage engines. It also delivered major observability and usability improvements, such as real-time progress reporting for various tasks and csvlog format for easier log processing and analysis. Additionally, partitioned tables saw significant performance improvements and matured considerably.
My personal connection to PG 12 runs deep - when I created Pigsty, an out-of-the-box PostgreSQL database distribution, PG 12 was the first major version we publicly supported. It’s remarkable how five years have flown by; I still vividly remember adapting features from PG 11 to PG 12.
During these five years, Pigsty evolved from a personal PG monitoring system/testing sandbox into a widely-used open source project with global community recognition. Looking back, I can’t help but feel a sense of accomplishment.
PG 17 Takes the Throne
As one version departs, another ascends. Following PostgreSQL’s versioning policy, today’s routine quarterly release brings us PostgreSQL 17.1.
My friend Longxi Shuai from Qunar likes to upgrade immediately when a new PG version drops, while I prefer to wait for the first minor release after a major version launch.
Typically, many small fixes and refinements appear in the x.1 release following a major version. Additionally, the three-month buffer provides enough time for the PostgreSQL ecosystem of extensions to catch up and support the new major version - a crucial consideration for users of the PG ecosystem.
From PG 12 to the current PG 17, the PostgreSQL community has added 48 new feature enhancements and introduced 130 performance improvements. PostgreSQL 17’s write throughput, according to official statements, shows up to a 2x improvement in some scenarios compared to previous versions - making it well worth the upgrade.
https://smalldatum.blogspot.com/2024/09/postgres-17rc1-vs-sysbench-on-small.html
I conducted a comprehensive performance evaluation of PostgreSQL 14 three years ago, and I’m planning to run a fresh benchmark on PostgreSQL 17.1.
I recently acquired a beast of a physical machine: 128 cores, 256GB RAM, with four 3.2TB Gen4 NVMe SSDs plus a hardware NVMe RAID acceleration card. I’m eager to see what performance PostgreSQL, pgvector, and various OLAP extensions can achieve on this hardware monster - stay tuned for the results.
Overall, I believe 17.1’s release represents an opportune time to upgrade. I plan to release Pigsty v3.1 in the coming days, which will promote PG 17 as Pigsty’s default major version, replacing PG 16.
Considering that PostgreSQL has offered logical replication since 10.0, and Pigsty provides a complete solution for blue-green deployment upgrades using logical replication without downtime, major version upgrades are far less challenging than they once were. I’ll soon publish a tutorial on zero-downtime major version upgrades to help users seamlessly upgrade from PostgreSQL 16 or earlier versions to PG 17.
PG 17 Extensions
One particularly encouraging development is how quickly the PostgreSQL extension ecosystem has adapted to PG 17 compared to the transition from PG 15 to PG 16.
Last year, PG 16 was released in mid-September, but it took nearly six months for major extensions to catch up. For instance, TimescaleDB, a core extension in the PG ecosystem, only completed PG 16 support in early February with version 2.13. Other extensions followed similar timelines.
Only after PG 16 had been out for six months did it reach a satisfactory state. That’s when Pigsty promoted PG 16 to its default major version, replacing PG 15.
Version | Date | Summary | Link |
---|---|---|---|
v3.1.0 | 2024-11-20 | PG 17 as default, config simplification, Ubuntu 24 & ARM support | WIP |
v3.0.4 | 2024-10-30 | PG 17 extensions, OLAP suite, pg_duckdb | v3.0.4 |
v3.0.3 | 2024-09-27 | PostgreSQL 17, Etcd maintenance optimizations, IvorySQL 3.4, PostGIS 3.5 | v3.0.3 |
v3.0.2 | 2024-09-07 | Streamlined installation, PolarDB 15 support, monitor view updates | v3.0.2 |
v3.0.1 | 2024-08-31 | Routine fixes, Patroni 4 support, Oracle compatibility improvements | v3.0.1 |
v3.0.0 | 2024-08-25 | 333 extensions, pluggable kernels, MSSQL, Oracle, PolarDB compatibility | v3.0.0 |
v2.7.0 | 2024-05-20 | Extension explosion with 20+ powerful new extensions and Docker applications | v2.7.0 |
v2.6.0 | 2024-02-28 | PG 16 as default, introduced ParadeDB and DuckDB extensions | v2.6.0 |
v2.5.1 | 2023-12-01 | Routine minor update, key PG16 extension support | v2.5.1 |
v2.5.0 | 2023-09-24 | Ubuntu/Debian support: bullseye, bookworm, jammy, focal | v2.5.0 |
v2.4.1 | 2023-09-24 | Supabase/PostgresML support & new extensions: graphql, jwt, pg_net, vault | v2.4.1 |
v2.4.0 | 2023-09-14 | PG16, RDS monitoring, service consulting, new extensions: Chinese FTS/graph/HTTP/embeddings | v2.4.0 |
v2.3.1 | 2023-09-01 | PGVector with HNSW, PG 16 RC1, docs overhaul, Chinese docs, routine fixes | v2.3.1 |
v2.3.0 | 2023-08-20 | Host VIP, ferretdb, nocodb, MySQL stub, CVE fixes | v2.3.0 |
v2.2.0 | 2023-08-04 | Dashboard & provisioning overhaul, UOS compatibility | v2.2.0 |
v2.1.0 | 2023-06-10 | Support for PostgreSQL 12 ~ 16beta | v2.1.0 |
v2.0.2 | 2023-03-31 | Added pgvector support, fixed MinIO CVE | v2.0.2 |
v2.0.1 | 2023-03-21 | v2 bug fixes, security enhancements, Grafana version upgrade | v2.0.1 |
v2.0.0 | 2023-02-28 | Major architectural upgrade, significantly improved compatibility, security, maintainability | v2.0.0 |
This time, the ecosystem adaptation from PG 16 to PG 17 has accelerated significantly - completing in less than three months what previously took six. I’m proud to say I’ve contributed substantially to this effort.
As I described in “PostgreSQL’s Ultimate Power: The Most Complete Extension Repository”, I maintain a repository that covers over half of the extensions in the PG ecosystem.
I recently completed the massive task of building over 140 extensions for PG 17 (also adding Ubuntu 24.04 and partial ARM support), while personally fixing or coordinating fixes for dozens of extensions with compatibility issues. The result: on EL systems, 301 out of 334 available extensions now work on PG 17, while on Debian systems, 302 out of 326 extensions are PG 17-compatible.
Entry / Filter | All | PGDG | PIGSTY | CONTRIB | MISC | MISS | PG17 | PG16 | PG15 | PG14 | PG13 | PG12 |
---|---|---|---|---|---|---|---|---|---|---|---|---|
RPM Extension | 334 | 115 | 143 | 70 | 4 | 6 | 301 | 330 | 333 | 319 | 307 | 294 |
DEB Extension | 326 | 104 | 144 | 70 | 4 | 14 | 302 | 322 | 325 | 316 | 303 | 293 |
Pigsty has achieved grand unification of the PostgreSQL extension ecosystem
Among major extensions, only a few remain without PG 17 support: the distributed extension Citus, the columnar storage extension Hydra, graph database extension AGE, and PGML. However, all other powerful extensions are now PG 17-ready.
Particularly noteworthy is the recent OLAP DuckDB integration competition in the PG ecosystem. ParadeDB’s pg_analytics
, personal developer Hongyan Li’s duckdb_fdw
, CrunchyData’s pg_parquet
, MooncakeLab’s pg_mooncake
, and even pg_duckdb
from Hydra and DuckDB’s parent company MotherDuck - all now support PG 17 and are available in the Pigsty extension repository.
Considering that Citus has a relatively small user base, and the columnar Hydra already has numerous DuckDB extensions as alternatives, I believe PG 17 has reached a satisfactory state for extension support and is ready for production use as the primary major version. Achieving this milestone took about half the time it required for PG 16.
About Pigsty v3.1
Pigsty is a free and open-source, out-of-the-box PostgreSQL database distribution that allows users to deploy enterprise-grade RDS cloud database services locally with a single command, helping users leverage PostgreSQL - the world’s most advanced open-source database.
PostgreSQL is undoubtedly becoming the Linux kernel of the database world, and Pigsty aims to be its Debian distribution. Our PostgreSQL database distribution offers six key value propositions:
- Provides the most comprehensive extension support in the PostgreSQL ecosystem
- Delivers the most powerful and complete monitoring system in the PostgreSQL ecosystem
- Offers out-of-the-box, user-friendly tools and best practices
- Provides self-healing, low-maintenance high availability and PITR experience
- Delivers reliable deployment directly on bare OS without containers
- No vendor lock-in, a democratized RDS experience with full control
Worth mentioning, we’ve added PG-derived kernel replacement capabilities in Pigsty v3, allowing you to use derivative PG kernels for unique features and capabilities:
- Microsoft SQL Server-compatible Babelfish kernel support
- Oracle-compatible IvorySQL 3.4 kernel support
- Alibaba Cloud PolarDB for PostgreSQL/Oracle kernel support
- Easier self-hosting of Supabase - the open-source Firebase alternative and all-in-one backend platform
If you’re looking for an authentic PostgreSQL experience, we welcome you to try our distribution - it’s open-source, free, and comes with no vendor lock-in. We also offer commercial consulting support to solve challenging issues and provide peace of mind.
The idea way to install PostgreSQL Extensions
PostgreSQL Is Eating the Database World through the power of extensibility. With 400 extensions powering PostgreSQL, we may not say it’s invincible, but it’s definitely getting much closer.
I believe the PostgreSQL community has reached a consensus on the importance of extensions. So the real question now becomes: “What should we do about it?”
What’s the primary problem with PostgreSQL extensions? In my opinion, it’s their accessibility. Extensions are useless if most users can’t easily install and enable them. But it’s not that easy.
Even the largest cloud postgres vendors are struggling with this. They have some inherent limitations (multi-tenancy, security, licensing) that make it hard for them to fully address this issue.
So here’s my plan, I’ve created a repository that hosts 400 of the most capable extensions in the PostgreSQL ecosystem, available as RPM / DEB packages on mainstream Linux OS distros. And the goal is to take PostgreSQL one solid step closer to becoming the all-powerful database and achieve the great alignment between the Debian and EL OS ecosystems.
The status quo
The PostgreSQL ecosystem is rich with extensions, but how do you actually install and use them? This initial hurdle becomes a roadblock for many. There are some existing solutions:
PGXN says, “You can download and compile extensions on the fly with pgxnclient
.”
Tembo says, “We have prepared pre-configured extension stack as Docker images.”
StackGres & Omnigres says, “We download .so
files on the fly.” All solid ideas.
While based on my experience, the vast majority of users still rely on their operating system’s package manager to install PG extensions. On-the-fly compilation and downloading shared libraries might not be a viable option for production env. Since many DB setups don’t have internet access or a proper toolchain ready.
In the meantime, Existing OS package managers like yum
/dnf
/apt
already solve issues like dependency resolution, upgrades, and version management well.
There’s no need to reinvent the wheel or disrupt existing standards. So the real question is: Who’s going to package these extensions into ready-to-use software?
PGDG has already made a fantastic effort with official YUM and APT repositories. In addition to the 70 built-in Contrib extensions bundled with PostgreSQL,the PGDG YUM repo offers 128 RPM extensions, while the APT repo offers 104 DEB extensions. These extensions are compiled and packaged in the same environment as the PostgreSQL kernel, making them easy to install alongside the PostgreSQL binary packages. In fact, even most PostgreSQL Docker images rely on the PGDG repo to install extensions.
I’m deeply grateful for Devrim’s maintenance of the PGDG YUM repo and Christoph’s work with the APT repo. Their efforts to make PostgreSQL installation and extension management seamless are incredibly valuable. But as a distribution creator myself, I’ve encountered some challenges with PostgreSQL extension distribution.
What’s the challenge?
The first major issue facing extension users is Alignment.
In the two primary Linux distro camps — Debian and EL — there’s a significant number of PostgreSQL extensions. Excluding the 70 built-in Contrib extensions bundled with PostgreSQL, the YUM repo offers 128 extensions, and the APT repo provides 104.
However, when we dig deeper, we see that alignment between the two repos is not ideal. The combined total of extensions across both repos is 153, but the overlap is just 79. That means only half of the extensions are available in both ecosystems!
Only half of the extensions are available in both EL and Debian ecosystems!
Next, we run into further alignment issues within each ecosystem itself. The availability of extensions can vary between different major OS versions.
For instance, pljava
, sequential_uuids
, and firebird_fdw
are only available in EL9, but not in EL8. Similarly, rdkit
is available in Ubuntu 22+ / Debian 12+, but not in Ubuntu 20 / Debian 11.
There’s also the issue of architecture support. For example, citus
does not provide arm64
packages in the Debian repo.
And then we have alignment issues across different PostgreSQL major versions. Some extensions won’t compile on older PostgreSQL versions, while others won’t work on newer ones. Some extensions are only available for specific PostgreSQL versions in certain distributions, and so on.
These alignment issues lead to a significant number of permutations. For example, if we consider five mainstream OS distributions (el8, el9, debian12, ubuntu22, ubuntu24),
two CPU architectures (x86_64
and arm64
), and six PostgreSQL major versions (12–17), that’s 60-70 RPM/DEB packages per extension—just for one extension!
On top of alignment, there’s the problem of completeness. PGXN lists over 375 extensions, but the PostgreSQL ecosystem could have as many as 1,000+. The PGDG repos, however, contain only about one-tenth of them.
There are also several powerful new Rust-based extensions that PGDG doesn’t include, such as pg_graphql
, pg_jsonschema
, and wrappers
for self-hosting Supabase;
pg_search
as an Elasticsearch alternative; and pg_analytics
, pg_parquet
, pg_mooncake
for OLAP processing. The reason? They are too slow to compile…
What’s the solution?
Over the past six months, I’ve focused on consolidating the PostgreSQL extension ecosystem. Recently, I reached a milestone I’m quite happy with. I’ve created a PG YUM/APT repository with a catalog of 400available PostgreSQL extensions.
Here are some key stats for the repo: It hosts 400 extensions in total. Excluding the 70 built-in extensions that come with PostgreSQL, this leaves 270 third-party extensions. Of these, about half are maintained by the official PGDG repos (126 RPM, 102 DEB). The other half (131 RPM, 143DEB) are maintained, fixed, compiled, packaged, and distributed by myself.
OS \ Entry | All | PGDG | PIGSTY | CONTRIB | MISC | MISS | PG17 | PG16 | PG15 | PG14 | PG13 | PG12 |
---|---|---|---|---|---|---|---|---|---|---|---|---|
RPM | 334 | 115 | 143 | 70 | 4 | 6 | 301 | 330 | 333 | 319 | 307 | 294 |
DEB | 326 | 104 | 144 | 70 | 4 | 14 | 302 | 322 | 325 | 316 | 303 | 293 |
For each extension, I’ve built versions for the 6 major PostgreSQL versions (12–17) across five popular Linux distributions: EL8, EL9, Ubuntu 22.04, Ubuntu 24.04, and Debian 12. I’ve also provided some limited support for older OS versions like EL7, Debian 11, and Ubuntu 20.04.
This repository also addresses most of the alignment issue. Initially, there were extensions in the APT and YUM repos that were unique to each, but I’ve worked to port as many of these unique extensions to the other ecosystem. Now, only 7 APT extensions are missing from the YUM repo, and 16 extensions are missing in APT—just 6% of the total. Many missing PGDG extensions have also been resolved.
I’ve created a comprehensive directory listing all supported extensions, with detailed info, dependency installation instructions, and other important notes.
I hope this repository can serve as the ultimate solution to the frustration users face when extensions are difficult to find, compile, or install.
How to use this repo?
Now, for a quick plug — what’s the easiest way to install and use these extensions?
The simplest option is to use the OSS PostgreSQL distribution: Pigsty. The repo is autoconfigured by default, so all you need to do is declare them in the config inventory.
For example, the self-hosting supabase template requires extensions that aren’t available in the PGDG repo. You can simply download, install, preload, config and create extensions by referring to their names.
all:
children:
pg-meta:
hosts: { 10.10.10.10: { pg_seq: 1, pg_role: primary } }
vars:
pg_cluster: pg-meta
# INSTALL EXTENSIONS
pg_extensions:
- supabase # essential extensions for supabase
- timescaledb postgis pg_graphql pg_jsonschema wrappers pg_search pg_analytics pg_parquet plv8 duckdb_fdw pg_cron pg_timetable pgqr
- supautils pg_plan_filter passwordcheck plpgsql_check pgaudit pgsodium pg_vault pgjwt pg_ecdsa pg_session_jwt index_advisor
- pgvector pgvectorscale pg_summarize pg_tiktoken pg_tle pg_stat_monitor hypopg pg_hint_plan pg_http pg_net pg_smtp_client pg_idkit
# LOAD EXTENSIONS
pg_libs: 'pg_stat_statements, plpgsql, plpgsql_check, pg_cron, pg_net, timescaledb, auto_explain, pg_tle, plan_filter'
# CONFIG EXTENSIONS
pg_parameters:
cron.database_name: postgres
pgsodium.enable_event_trigger: off
# CREATE EXTENSIONS
pg_databases:
- name: postgres
baseline: supabase.sql
schemas: [ extensions ,auth ,realtime ,storage ,graphql_public ,supabase_functions ,_analytics ,_realtime ]
extensions:
- { name: pgcrypto ,schema: extensions }
- { name: pg_net ,schema: extensions }
- { name: pgjwt ,schema: extensions }
- { name: uuid-ossp ,schema: extensions }
- { name: pgsodium }
- { name: supabase_vault }
- { name: pg_graphql }
- { name: pg_jsonschema }
- { name: wrappers }
- { name: http }
- { name: pg_cron }
- { name: timescaledb }
- { name: pg_tle }
- { name: vector }
vars:
pg_version: 17
# DOWNLOAD EXTENSIONS
repo_extra_packages:
- pgsql-main
- supabase # essential extensions for supabase
- timescaledb postgis pg_graphql pg_jsonschema wrappers pg_search pg_analytics pg_parquet plv8 duckdb_fdw pg_cron pg_timetable pgqr
- supautils pg_plan_filter passwordcheck plpgsql_check pgaudit pgsodium pg_vault pgjwt pg_ecdsa pg_session_jwt index_advisor
- pgvector pgvectorscale pg_summarize pg_tiktoken pg_tle pg_stat_monitor hypopg pg_hint_plan pg_http pg_net pg_smtp_client pg_idkit
To simply add extensions to existing clusters:
./infra.yml -t repo_build -e '{"repo_packages":[citus]}' # download
./pgsql.yml -t pg_extension -e '{"pg_extensions": ["citus"]}' # install
Through this repo was meant to be used with Pigsty, But it is not mandatory. You can still enable this repository on any EL/Debian/Ubuntu system with a simple one-liner in the shell:
APT Repo
For Ubuntu 22.04 & Debian 12 or any compatible platforms, use the following commands to add the APT repo:
curl -fsSL https://repo.pigsty.io/key | sudo gpg --dearmor -o /etc/apt/keyrings/pigsty.gpg
sudo tee /etc/apt/sources.list.d/pigsty-io.list > /dev/null <<EOF
deb [signed-by=/etc/apt/keyrings/pigsty.gpg] https://repo.pigsty.io/apt/infra generic main
deb [signed-by=/etc/apt/keyrings/pigsty.gpg] https://repo.pigsty.io/apt/pgsql/$(lsb_release -cs) $(lsb_release -cs) main
EOF
sudo apt update
YUM Repo
For EL 8/9 and compatible platforms, use the following commands to add the YUM repo:
curl -fsSL https://repo.pigsty.io/key | sudo tee /etc/pki/rpm-gpg/RPM-GPG-KEY-pigsty >/dev/null # add gpg key
curl -fsSL https://repo.pigsty.io/yum/repo | sudo tee /etc/yum.repos.d/pigsty.repo >/dev/null # add repo file
sudo yum makecache
What’s in this repo?
In this repo, all the extensions are categorized into one of the 15 categories: TIME, GIS, RAG, FTS, OLAP, FEAT, LANG, TYPE, FUNC, ADMIN, STAT, SEC, FDW, SIM, ETL, as shown below.
Check pigsty.io/ext for all the details.
Some Thoughts
Each major PostgreSQL version introduces changes, making the maintenance of 140+ extension packages a bit of a beast.
Especially when some extension authors haven’t updated their work in years. In these cases, you often have no choice but to take matters into your own hands. I’ve personally fixed several extensions and ensured they support the latest PostgreSQL major versions. For those authors I could reach, I’ve submitted numerous PRs and issues to keep things moving forward.
Back to the point: my goal with this repo is to establish a standard for PostgreSQL extension installation and distribution, solving the distribution challenges that have long troubles the users.
A recent milestone is that, the popular open-source PostgreSQL HA cluster project postgresql_cluster, has made this extension repository the default upstream for PG extension installation.
Currently, this repository (repo.pigsty.io) is hosted on Cloudflare. In the past month, the repo and its mirrors have served about 300GB of downloads. Given that most extensions are just a few KB to a few MB, that amounts to nearly a million downloads per month. Since Cloudflare doesn’t charge for traffic, I can confidently commit to keeping this repository completely free & under active maintenance for the foreseeable future, as long as cloudflare doesn’t charge me too much.
I believe my work can help PostgreSQL users worldwide and contribute to the thriving PostgreSQL ecosystem. I hope it proves useful to you as well. Enjoy PostgreSQL!
PostgreSQL 17 Released: The Database That's Not Just Playing Anymore!
The annual PostgreSQL major release is here! What surprises does PostgreSQL 17 have in store for us?
In this release announcement, the PostgreSQL global community has finally dropped the act — sorry, we’re not playing anymore — “PostgreSQL is now the world’s most advanced open-source database, becoming the preferred choice for organizations of all sizes”. While not explicitly naming names, the official announcement comes incredibly close to shouting “we’re taking down the top commercial databases” (Oracle).
In my article “PostgreSQL is Eating the Database World” published earlier this year, I argued that scalability is PostgreSQL’s unique core advantage. I’m thrilled to see this become the focus and consensus of the PostgreSQL community in just six months, as demonstrated at PGCon.Dev 2024 and this PostgreSQL 17 release.
Regarding new features, I’ve already covered them in my article “PostgreSQL 17 Beta1 Released! The Performance Tube is Bursting!”, so I won’t repeat them here. This major version brings many new features, but what impressed me most is that PG managed to double its write throughput on top of its already impressive performance — unpretentiously powerful.
However, beyond specific features, I believe the biggest transformation in the PG community is in its mindset and spirit — in this release announcement, PostgreSQL has dropped the “relational” qualifier from its slogan “the world’s most advanced open-source relational database”, becoming simply “the world’s most advanced open-source database”. And in the “About PostgreSQL” section, it states: “PG’s feature set, advanced capabilities, scalability, security, and stability now rival and even surpass top-tier commercial databases”. So I think it won’t be long before the “open-source” qualifier can be dropped too, becoming simply “the world’s most advanced database”.
The PostgreSQL beast has awakened — it’s no longer the peaceful, non-confrontational entity of the past, but has transformed into an aggressive, forward-looking force — ready to take over and conquer the entire database world. And countless capital has already flooded into the PostgreSQL ecosystem, with PG-based startups taking almost all the new money in database funding. PostgreSQL is destined to become the “Linux kernel” of the database world, and DBMS disputes might evolve into internal PostgreSQL distribution battles in the future. Let’s wait and see.
Original: PostgreSQL 17 Release Notes
Can PostgreSQL Replace Microsoft SQL Server?
Many people don’t have a clear picture of how far the PostgreSQL ecosystem has evolved. Beyond devouring the database world with its all-encompassing extension ecosystem, PostgreSQL can now directly replace Oracle, SQL Server, and MongoDB at the kernel level – and MySQL is even less of a challenge.
When it comes to mainstream databases at highest risk of displacement, Microsoft SQL Server undeniably tops the list. MSSQL faces the most comprehensive replacement – right down to the wire protocol level. And who’s leading this charge? None other than AWS, Amazon’s cloud service division.
Babelfish
While I’ve often criticized cloud vendors for free-riding on open source, I must admit this strategy is extremely effective. AWS has leveraged the open-source PostgreSQL and MySQL kernels to dominate the database market, punching Oracle and kicking Microsoft, becoming the undisputed market leader. In recent years, AWS has pulled off an even more brilliant move by developing the BabelfishPG extension, providing compatibility at the “wire protocol” level.
“Wire protocol compatibility” means clients don’t need to change anything – they can still access the SQL Server port 1433 using MSSQL drivers and command-line tools (sqlcmd) to connect to clusters equipped with BabelfishPG. Even more remarkably, you can still use PostgreSQL’s protocol language syntax to access the original 5432 port, coexisting with SQL Server clients – this creates tremendous convenience for migration.
WiltonDB
Of course, Babelfish isn’t merely a simple PG extension plugin. It makes minor modifications and adaptations to the PostgreSQL kernel and provides four extension plugins that offer TSQL syntax support, TDS wire protocol support, data types, and other function support.
Compiling and packaging such kernels and extensions on different platforms isn’t a simple task. That’s where WiltonDB – a Babelfish distribution – comes in. It compiles and packages BabelfishPG as RPM/DEB/MSI packages for EL 7/8/9, Ubuntu systems, and even Windows.
Pigsty v3
Having RPM/DEB packages alone is still far from offering production-grade services. In the recently released Pigsty v3, we provide the ability to replace the native PostgreSQL kernel with BabelfishPG.
Creating such an MSSQL cluster requires only modifying a few parameters in the cluster definition. The setup process remains fool-proof – similar to primary-replica setup, extension installation, parameter optimization, user configuration, HBA rule settings, and even service traffic distribution will automatically be established based on the configuration file.
In practice, you can treat a Babelfish cluster exactly like a regular PostgreSQL cluster for use and management. The only difference is that clients can choose whether to use TSQL protocol support on port 1433 in addition to the PGSQL protocol on port 5432.
For example, you can easily redirect the Primary service (which originally points to the primary database connection pool on port 6432) to port 1433 through configuration, achieving seamless TDS/TSQL traffic switching during failovers.
This means that capabilities originally belonging to PostgreSQL RDS – high availability, point-in-time recovery, monitoring systems, IaC control, SOP plans, and countless extension plugins – can all be grafted onto the SQL Server version kernel.
How to Migrate?
Beyond powerful kernels and extensions like Babelfish, the PostgreSQL ecosystem boasts a flourishing toolkit ecosystem. For migrations from SQL Server or MySQL to PostgreSQL, I strongly recommend a killer migration tool: PGLOADER.
This migration tool is ridiculously straightforward – under ideal conditions, you only need connection strings for both databases to complete the migration. Yes, really, without a single line of unnecessary code.
pgloader mssql://user@mshost/dbname pgsql://pguser@pghost/dbname
With MSSQL-compatible kernel extensions and migration tools, moving existing SQL Server installations becomes remarkably easy.
Beyond MSSQL…
Besides MSSQL, the PostgreSQL ecosystem offers Oracle replacements like PolarDB O and IvorySQL, MongoDB replacements like FerretDB and PongoDB, as well as over three hundred extensions providing various functionalities.
In fact, nearly the entire database world is feeling PostgreSQL’s impact – except for those that occupy different ecological niches (SQLite, DuckDB, MinIO) or are essentially PostgreSQL in disguise (Supabase, RDS, Aurora/Polar).
Our recently released open-source PostgreSQL RDS solution, Pigsty, now supports these PG replacement kernels, allowing users to provide MSSQL, Oracle, MongoDB, Firebase, and MongoDB compatibility within a single PostgreSQL deployment.
But due to space limitations, those will be topics for upcoming articles.
Whoever Integrates DuckDB Best Wins the OLAP World
In the post “PostgreSQL is Eating the World”, I posed a question: Who will ultimately unify the database world? My take is that it’ll be the PostgreSQL ecosystem coupled with a rich variety of extension plugins. And I believe that to conquer OLAP—arguably the biggest and most distinct kingdom in the database domain—this “analysis extension” absolutely has something to do with DuckDB.
I’ve long been a huge fan of PostgreSQL. But interestingly, my second favorite database over the past two years has shifted from Redis to DuckDB. DuckDB is a very compact yet powerful embedded OLAP database, achieving top-tier performance and usability in analytics. It also ranks just behind PostgreSQL in terms of extensibility.
Much like the vector database extension race two years back, the new frontier in the PG ecosystem is a competition centered around DuckDB—“Whoever integrates DuckDB into PG more elegantly will be the future champion of the OLAP world.” Although many participants are sharpening their swords for this battle, DuckDB’s official entry into the race leaves no doubt that the competition is about to ignite.
DuckDB: A Rising Challenger in the OLAP Space
DuckDB was created by database researchers Mark Raasveldt and Hannes Mühleisen at the Centrum Wiskunde & Informatica (CWI) in Amsterdam. CWI is not just a research institute—it’s arguably the hidden powerhouse behind the development of analytical databases, pioneering columnar storage engines and vectorized query execution. Products like ClickHouse, Snowflake, and Databricks all carry CWI’s influence. Fun fact: Guido van Rossum (a.k.a. the father of Python) also created the Python language while at CWI.
Now these pioneers in analytical research are directly bringing their expertise to an OLAP database, choosing a smart timing and niche by introducing DuckDB.
DuckDB was born from observing database users’ pain points: data scientists mostly rely on tools like Python and Pandas and are less familiar with traditional databases. They’re often bogged down by hassles like connectivity, authentication, data import/export, and so on. So why not build a simple, embedded analytical database for them—kinda like SQLite for analytics?
The entire DuckDB project is essentially one header file and one C++ file, which compiles into a standalone binary. The database itself is just a single file. It uses a PostgreSQL-compatible parser and syntax, making it almost frictionless for newcomers. Though DuckDB seems refreshingly simple, its most impressive feature is “simplicity without compromise”—it boasts world-class analytical performance. For instance, on ClickHouse’s own benchmark site (ClickBench), DuckDB can beat the local champion on its home turf.
Another highlight: because DuckDB’s creators are government-funded researchers, they consider offering their work to everyone for free a social responsibility. Thus, DuckDB is released under the very permissive MIT License.
I believe DuckDB’s rise is inevitable: a database that’s blazing fast, requires virtually zero setup, and is also open-source and free—it’s hard not to become popular. In StackOverflow’s 2023 Developer Survey, DuckDB made the “Most Popular Databases” list for the first time at a 0.61% usage rate (29th place, fourth from the bottom). Just one year later, in the 2024 survey, DuckDB saw a 2.3x growth in popularity (1.4%), nearly catching up to ClickHouse (1.7%).
At the same time, DuckDB has garnered an excellent reputation among its users. In terms of developer appreciation and satisfaction (69.2%), it’s second only to PostgreSQL (74.5%) among major databases. If we look at DB-Engine’s popularity trend, it’s clear that since 2022, DuckDB has been on a meteoric rise—though it’s still nowhere near PostgreSQL levels, it has already surpassed every other NewSQL product in popularity scores.
DuckDB’s Weaknesses—and the Opportunity They Present
DuckDB can be used as a standalone database, but it truly shines as an embedded analytical engine. Being “embedded” is both a strength and a weakness. While DuckDB boasts top-notch analytics performance, its biggest shortcoming is its rather minimal data-management capabilities—the stuff data scientists hate dealing with: ACID, concurrent access, access control, data persistence, HA, database import/export… Ironically, these are precisely the strong suits of classic databases and the core pain points for enterprise analytics systems.
We can expect a wave of DuckDB “sidecar” products to address these gaps. It’s reminiscent of what happened when Facebook open-sourced RocksDB (a KV store): countless “new database” projects merely slapped a thin SQL layer on top of RocksDB and sold themselves as the next big thing—Yet another SQL sidecar for RocksDB. The same phenomenon happened with the vector search library hnswlib—numerous “specialized vector databases” sprang up, all just wrapping hnswlib. And with Lucene or its next-gen replacement Tantivy, we’ve seen a flurry of “full-text search databases” that are basically wrapped versions of those engines.
In fact, this is already happening within the PostgreSQL ecosystem. Before other database companies realized what was happening, five PG players jumped into the race, including ParadeDB’s pg_lakehouse
, duckdb_fdw
by independent developer Li Hongyan, CrunchyData’s crunchy_bridge
, Hydra’s pg_quack
, and now the official DuckDB team has arrived with a PG extension—pg_duckdb
.
The Second PG Extension Grand Prix
It reminds me of the vector database extension frenzy in the PG community over the past year. As AI went mainstream, the PG world saw at least six vector extensions (pgvector
, pgvector.rs
, pg_embedding
, latern
, pase
, pgvectorscale
) racing to outdo each other. Eventually, pgvector
, boosted heavily by AWS and others, steamrolled the specialized vector-database market before Oracle/MySQL/MariaDB even rolled out their half-baked offerings.
So, who will become the “pgvector” of the PG OLAP ecosystem? Personally, I’d bet on the official extension overshadowing community ones. Although pg_duckdb
has only just arrived—it hasn’t even hit version v0.0.1 yet—its architectural design suggests it’s likely the future winner. Indeed, this extension arms race has only just started, but it’s already converging fast:
Hydra (YC W22), which originally forked Citus’ column store extension to create pg_quack
, was so impressed by DuckDB that they abandoned their own engine and teamed up with MotherDuck to build pg_duckdb
. This extension, blending Hydra’s PG know-how with DuckDB’s native expertise, can seamlessly read PG tables inside your database, use DuckDB for computation, and directly read Parquet/IceBerg formats from the filesystem/S3—thus creating a “data lakehouse” setup.
Similarly, ParadeDB (YC S23)—another YC-backed startup—originally built pg_analytics
in Rust for OLAP capabilities, achieving decent traction. They, too, switched gears to build a DuckDB-based pg_lakehouse
. Right after the pg_duckdb
announcement, ParadeDB founder Phillipe essentially waved the white flag and said they’d develop on top of pg_duckdb
rather than compete against it.
Meanwhile, Chinese independent developer Li Hongyan created duckdb_fdw
as a different approach altogether—using PostgreSQL’s foreign-data-wrapper infrastructure to connect PG and DuckDB. The official DuckDB folks publicly critiqued this, highlighting it as a “bad example,” possibly motivating the birth of “pg_duckdb”: “We have grand visions for uniting PG and Duck, but you moved too fast—here’s the official shock and awe.”
As for CrunchyData’s crunchy_bridge
or any other closed-source wrappers, I suspect they’ll struggle to gain broader adoption.
Of course, as the author of the PostgreSQL distribution Pigsty, my position is simply—let them race. I’ll bundle all these extensions and distribute them to end users, so they can pick whatever suits them best. Just like when vector databases were on the rise, I bundled pgvector
, pg_embedding
, pase
, pg_sparse
, etc.—the most promising candidates. It doesn’t matter who ultimately wins; PG and Pigsty will always be the ones reaping the spoils.
Speed trumps all, so in Pigsty v3 I’ve already integrated the three most promising extensions: pg_duckdb
, pg_lakehouse
, and duckdb_fdw
, plus the main duckdb
binary—all ready to go out of the box. Users can experience a one-stop PostgreSQL solution that handles both OLTP and OLAP—truly an all-conquering HTAP dream come true.
StackOverflow 2024 Survey: PostgreSQL Is Dominating the Field
2024 StackOverflow Global Developer Survey Results have just been released, featuring high-quality responses from 60,000 developers in 185 countries and regions. Naturally, as a seasoned database aficionado, the part I’m most interested in is the “Database” section of the survey:
Popularity
First up is database popularity: Database usage among professional developers
A technology’s popularity is measured by the proportion of overall respondents who used it in the past year. This figure reflects accumulated usage over that period—think of it as the market “stock” metric, the most fundamental indicator of current status.
In terms of usage, PostgreSQL leads the pack among professional developers at 51.9%, clinching the top spot for the third consecutive year—and surpassing the 50% mark for the first time ever! The gap between PostgreSQL and the second-place MySQL (39.4%) has widened to 12.5 percentage points (it was 8.5 points last year).
Looking at the entire developer community (including non-professionals), PostgreSQL has become the world’s most popular database for the second year in a row, at 48.7% usage—pulling 8.4 points ahead of MySQL (40.3%), compared to a 4.5-point lead in the previous year.
Plotting the past eight years of survey data on a scatter chart shows PostgreSQL enjoying a near-linear, high-speed growth trend.
Besides PostgreSQL, other databases seeing notable growth include SQLite, DuckDB, Supabase, BigQuery, Snowflake, and Databricks SQL. BigQuery, Snowflake, and Databricks are the darlings of the big data analytics realm, while SQLite and DuckDB occupy a unique embedded database niche that doesn’t really clash with traditional relational databases. Supabase, on the other hand, encapsulates PostgreSQL under the hood as its backend development platform.
All other databases have, to varying degrees, felt the impact of PostgreSQL’s rise.
Admiration & Demand
Next, let’s look at a database’s admiration (in red) and demand (in blue): Which databases are most loved and most wanted by all developers over the past year, sorted by demand.
So-called “reputation” (the red dot)—Loved/Admired—tells us what percentage of current users would be happy to keep using the technology. It’s like an annual “retention rate” that captures the perception and valuation of a database, reflecting its future growth potential.
In terms of reputation, PostgreSQL remains at the top for the second consecutive year, with a 74.5% admiration rate. Two other databases worth noting are SQLite and DuckDB, both of which have seen a significant uptick in user admiration in the past year, while TiDB experienced a dramatic drop (from 64.33 down to 48.8).
The proportion of all respondents who say they want to use a given technology next year is the “Wanted” or “Desired” rate (the blue dot). This metric indicates how many plan to adopt that technology in the coming year, making it a solid predictor of near-future growth. Hence the survey ranks databases by this demand figure.
This is PostgreSQL’s third consecutive year at the top, now boasting a commanding lead over the runners-up. Perhaps driven by recent interest in vector databases, PostgreSQL’s demand has skyrocketed—from 19% in 2022 to 47% in 2024. By contrast, MySQL’s demand fell behind even SQLite, slipping from second place in 2023 to third this year.
This demand metric—representing explicit statements like, “I plan to use this database next year”—accurately forecasts next year’s new adoption. That means this spike in demand for PostgreSQL will likely be reflected in higher popularity numbers next year.
Conclusion
For the second consecutive year, PostgreSQL has unequivocally staked its claim as the world’s most popular, most loved, and most in-demand database.
Looking at the eight-year trend and next year’s demand projections, it seems unlikely any rival will be able to topple PostgreSQL’s ascendancy.
MySQL, once PostgreSQL’s main competitor, clearly shows signs of decline, and other databases are feeling the pressure too. Those that continue to grow either avoid going head-to-head with PostgreSQL by occupying a different niche, or they’ve rebranded themselves into PostgreSQL-compatible or PostgreSQL-based solutions.
PostgreSQL is on track to become the Linux kernel of the database world, with the “PostgreSQL distribution wars” set to begin in earnest.
Self-Hosting Dify with PG, PGVector, and Pigsty
Dify – The Innovation Engine for GenAI Applications
Dify is an open-source LLM app development platform. Orchestrate LLM apps from agents to complex AI workflows, with an RAG engine. Which claims to be more production-ready than LangChain.
Of course, a workflow orchestration software like this needs a database underneath — Dify uses PostgreSQL for meta data storage, as well as Redis for caching and a dedicated vector database. You can pull the Docker images and play locally, but for production deployment, this setup won’t suffice — there’s no HA, backup, PITR, monitoring, and many other things.
Fortunately, Pigsty provides a battery-include production-grade highly available PostgreSQL cluster, along with the Redis and S3 (MinIO) capabilities that Dify needs, as well as Nginx to expose the Web service, making it the perfect companion for Dify.
Off-load the stateful part to Pigsty, you only need to pull up the stateless blue circle part with a simple
docker compose up
.
BTW, I have to criticize the design of the Dify template. Since the metadata is already stored in PostgreSQL, why not add pgvector
to use it as a vector database? What’s even more baffling is that pgvector
is a separate image and container. Why not just use a PG image with pgvector
included?
Dify “supports” a bunch of flashy vector databases, but since PostgreSQL is already chosen, using pgvector
as the default vector database is the natural choice. Similarly, I think the Dify team should consider removing Redis. Celery task queues can use PostgreSQL as backend storage, so having multiple databases is unnecessary. Entities should not be multiplied without necessity.
Therefore, the Pigsty-provided Dify Docker Compose template has made some adjustments to the official example. It removes the db
and redis
database images, using instances managed by Pigsty. The vector database is fixed to use pgvector
, reusing the same PostgreSQL instance.
In the end, the architecture is simplified to three stateless containers: dify-api
, dify-web
, and dify-worker
, which can be created and destroyed at will. There are also two optional containers, ssrf_proxy
and nginx
, for providing proxy and some security features.
There’s a bit of state management left with file system volumes, storing things like private keys. Regular backups are sufficient.
Reference:
Pigsty Preparation
Let’s take the single-node installation of Pigsty as an example. Suppose you have a machine with the IP address 10.10.10.10
and already pigsty installed.
We need to define the database clusters required in the Pigsty configuration file pigsty.yml
.
Here, we define a cluster named pg-meta
, which includes a superuser named dbuser_dify
(the implementation is a bit rough as the Migration script executes CREATE EXTENSION
which require dbsu privilege for now),
And there’s a database named dify
with the pgvector
extension installed, and a specific firewall rule allowing users to access the database from anywhere using a password (you can also restrict it to a more precise range, such as the Docker subnet 172.0.0.0/8
).
Additionally, a standard single-instance Redis cluster redis-dify
with the password redis.dify
is defined.
pg-meta:
hosts: { 10.10.10.10: { pg_seq: 1, pg_role: primary } }
vars:
pg_cluster: pg-meta
pg_users: [ { name: dbuser_dify ,password: DBUser.Dify ,superuser: true ,pgbouncer: true ,roles: [ dbrole_admin ] } ]
pg_databases: [ { name: dify, owner: dbuser_dify, extensions: [ { name: pgvector } ] } ]
pg_hba_rules: [ { user: dbuser_dify , db: all ,addr: world ,auth: pwd ,title: 'allow dify user world pwd access' } ]
redis-dify:
hosts: { 10.10.10.10: { redis_node: 1 , redis_instances: { 6379: { } } } }
vars: { redis_cluster: redis-dify ,redis_password: 'redis.dify' ,redis_max_memory: 64MB }
For demonstration purposes, we use single-instance configurations. You can refer to the Pigsty documentation to deploy high availability PG and Redis clusters. After defining the clusters, use the following commands to create the PG and Redis clusters:
bin/pgsql-add pg-meta # create the dify database cluster
bin/redis-add redis-dify # create redis cluster
Alternatively, you can define a new business user and business database on an existing PostgreSQL cluster, such as pg-meta
, and create them with the following commands:
bin/pgsql-user pg-meta dbuser_dify # create dify biz user
bin/pgsql-db pg-meta dify # create dify biz database
You should be able to access PostgreSQL and Redis with the following connection strings, adjusting the connection information as needed:
psql postgres://dbuser_dify:DBUser.Dify@10.10.10.10:5432/dify -c 'SELECT 1'
redis-cli -u redis://redis.dify@10.10.10.10:6379/0 ping
Once you confirm these connection strings are working, you’re all set to start deploying Dify.
For demonstration purposes, we’re using direct IP connections. For a multi-node high availability PG cluster, please refer to the service access section.
The above assumes you are already a Pigsty user familiar with deploying PostgreSQL and Redis clusters. You can skip the next section and proceed to see how to configure Dify.
Starting from Scratch
If you’re already familiar with setting up Pigsty, feel free to skip this section.
Prepare a fresh Linux x86_64 node that runs compatible OS, then run as a sudo-able user:
curl -fsSL https://repo.pigsty.io/get | bash
It will download Pigsty source to your home, then perform configure and install to finish the installation.
cd ~/pigsty # get pigsty source and entering dir
./bootstrap # download bootstrap pkgs & ansible [optional]
./configure # pre-check and config templating [optional]
# change pigsty.yml, adding those cluster definitions above into all.children
./install.yml # install pigsty according to pigsty.yml
You should insert the above PostgreSQL cluster and Redis cluster definitions into the pigsty.yml
file, then run install.yml
to complete the installation.
Redis Deploy
Pigsty will not deploy redis in install.yml
, so you have to run redis.yml
playbook to install Redis explicitly:
./redis.yml
Docker Deploy
Pigsty will not deploy Docker by default, so you need to install Docker with the docker.yml
playbook.
./docker.yml
Dify Confiugration
You can configure dify in the .env
file:
All parameters are self-explanatory and filled in with default values that work directly in the Pigsty sandbox env. Fill in the database connection information according to your actual conf, consistent with the PG/Redis cluster configuration above.
Changing the SECRET_KEY
field is recommended. You can generate a strong key with openssl rand -base64 42
:
# meta parameter
DIFY_PORT=8001 # expose dify nginx service with port 8001 by default
LOG_LEVEL=INFO # The log level for the application. Supported values are `DEBUG`, `INFO`, `WARNING`, `ERROR`, `CRITICAL`
SECRET_KEY=sk-9f73s3ljTXVcMT3Blb3ljTqtsKiGHXVcMT3BlbkFJLK7U # A secret key for signing and encryption, gen with `openssl rand -base64 42`
# postgres credential
PG_USERNAME=dbuser_dify
PG_PASSWORD=DBUser.Dify
PG_HOST=10.10.10.10
PG_PORT=5432
PG_DATABASE=dify
# redis credential
REDIS_HOST=10.10.10.10
REDIS_PORT=6379
REDIS_USERNAME=''
REDIS_PASSWORD=redis.dify
# minio/s3 [OPTIONAL] when STORAGE_TYPE=s3
STORAGE_TYPE=local
S3_ENDPOINT='https://sss.pigsty'
S3_BUCKET_NAME='infra'
S3_ACCESS_KEY='dba'
S3_SECRET_KEY='S3User.DBA'
S3_REGION='us-east-1'
Now we can pull up dify with docker compose:
cd pigsty/app/dify && make up
Expose Dify Service via Nginx
Dify expose web/api via its own nginx through port 80 by default, while pigsty uses port 80 for its own Nginx. T
herefore, we expose Dify via port 8001
by default, and use Pigsty’s Nginx to forward to this port.
Change infra_portal
in pigsty.yml
, with the new dify
line:
infra_portal: # domain names and upstream servers
home : { domain: h.pigsty }
grafana : { domain: g.pigsty ,endpoint: "${admin_ip}:3000" , websocket: true }
prometheus : { domain: p.pigsty ,endpoint: "${admin_ip}:9090" }
alertmanager : { domain: a.pigsty ,endpoint: "${admin_ip}:9093" }
blackbox : { endpoint: "${admin_ip}:9115" }
loki : { endpoint: "${admin_ip}:3100" }
dify : { domain: dify.pigsty ,endpoint: "10.10.10.10:8001", websocket: true }
Then expose dify web service via Pigsty’s Nginx server:
./infra.yml -t nginx
Don’t forget to add dify.pigsty
to your DNS or local /etc/hosts
/ C:\Windows\System32\drivers\etc\hosts
to access via domain name.
PGCon.Dev 2024, The conf that shutdown PG for a week
PGCon.Dev, once known as PGCon—the annual must-attend gathering for PostgreSQL hackers and key forum for its future direction, has been held in Ottawa since its inception in 2007.
This year marks a new chapter as the original organizer, Dan, hands over the reins to a new team, and the event moves to SFU’s Harbour Centre in Vancouver, kicking off a new era with grandeur.
How engaging was this event? Peter Eisentraut, member of the PostgreSQL core team, noted that during PGCon.Dev, there were no code commits to PostgreSQL – resulting in the longest pause in twenty years, a whopping week! a historic coding ceasefire! Why? Because all the developers were at the conference!
Considering the last few interruptions that occurred in the early days of the project twenty years ago,
I’ve been embracing PostgreSQL for a decade, but attending a global PG Hacker conference in person was a first for me, and I’m immensely grateful for the organizer’s efforts. PGCon.Dev 2024 wrapped up on May 31st, though this post comes a bit delayed as I’ve been exploring Vancouver and Banff National Park ;)
Day Zero: Extension Summit
Day zero is for leadership meetings, and I’ve signed up for the afternoon’s Extension Ecosystem Summit.
Maybe this summit is somewhat subtly related to my recent post, “Postgres is eating the database world,” highlighting PostgreSQL’s thriving extension ecosystem as a unique and critical success factor and drawing the community’s attention.
I participated in David Wheeler’s Binary Packing session along with other PostgreSQL community leaders. Despite some hesitation to new standards like PGXN v2 from current RPM/APT maintainers. In the latter half of the summit, I attended a session led by Yurii Rashkovskii, discussing extension directory structures, metadata, naming conflicts, version control, and binary distribution ideas.
Prior to this summit, the PostgreSQL community had held six mini-summits discussing these topics intensely, with visions for the extension ecosystem’s future development shared by various speakers. Recordings of these sessions are available on YouTube.
And after the summit, I had a chance to chat with Devrim, the RPM maintainer, about extension packing, which was quite enlightening.
“Keith Fan Group” – from Devrim on Extension Summit
Day One: Brilliant Talks and Bar Social
The core of PGCon.Dev lies in its sessions. Unlike some China domestic conferences with mundane product pitches or irrelevant tech details, PGCon.Dev presentations are genuinely engaging and substantive. The official program kicked off on May 29th, after a day of closed-door leadership meetings and the Ecosystem Summit on the 28th.
The opening was co-hosted by Jonathan Katz, 1 of the 7 core PostgreSQL team members and a chief product manager at AWS RDS, and Melanie Plageman, a recent PG committer from Microsoft. A highlight was when Andres Freund, the developer who uncovered the famous xz
backdoor, was celebrated as a superhero on stage.
Following the opening, the regular session tracks began. Although conference videos aren’t out yet, I’m confident they’ll “soon” be available on YouTube. Most sessions had three tracks running simultaneously; here are some highlights I chose to attend.
Pushing the Boundaries of PG Extensions
Yurii’s talk, “Pushing the Boundaries of PG Extensions,” tackled what kind of extension APIs PostgreSQL should offer. PostgreSQL boasts robust extensibility, but the current extension API set is decades old, from the 9.x era. Yurii’s proposal aims to address issues with the existing extension mechanisms. Challenges such as installing multiple versions of an extension simultaneously, avoiding database restarts post-extension installations, managing extensions as seamlessly as data, and handling dependencies among extensions were discussed.
Yurii and Viggy, founders of Omnigres, aim to transform PostgreSQL into a full-fledged application development platform, including hosting HTTP servers directly within the database. They designed a new extension API and management system for PostgreSQL to achieve this. Their innovative improvements represent the forefront of exploration into PostgreSQL’s core extension mechanisms.
I had a great conversation with Viggy and Yurii. Yurii walked me through compiling and installing Omni. I plan to support the Omni extension series in the next version of Pigsty, making this powerful application development framework plug-and-play.
Anarchy in DBMS
Abigale Kim from CMU, under the mentorship of celebrity professor Andy Pavlo, delivered the talk “Anarchy in the Database—A Survey and Evaluation of DBMS Extensibility.” This topic intrigued me since Pigsty’s primary value proposition is about PostgreSQL’s extensibility.
Kim’s research revealed interesting insights: PostgreSQL is the most extensible DBMS, supporting 9 out of 10 extensibility points, closely followed by DuckDB. With over 375+ available extensions, PostgreSQL significantly outpaces other databases.
Kim’s quantitative analysis of compatibility levels among these extensions resulted in a compatibility matrix, unveiling conflicts—most notably, powerful extensions like TimescaleDB and Citus are prone to clashes. This information is very valuable for users and distribution maintainers. Read the detailed study.
I joked with Kim that — now I could brag about PostgreSQL’s extensibility with her research data.
How PostgreSQL is Misused and Abused
The first-afternoon session featured Karen Jex from CrunchyData, an unusual perspective from a user — and a female DBA. Karen shared common blunders by PostgreSQL beginners. While I knew all of what was discussed, it reaffirmed that beginners worldwide make similar mistakes — an enlightening perspective for PG Hackers, who found the session quite engaging.
PostgreSQL and the AI Ecosystem
The second-afternoon session by Bruce Momjian, co-founder of the PGDG and a core committee member from the start, was unexpectedly about using PostgreSQL’s multi-dimensional arrays and queries to implement neural network inference and training.
Haha, some ArgParser code. I see it
During the lunch, Bruce explained that Jonathan Katz needed a topic to introduce the vector database extension PGVector in the PostgreSQL ecosystem, so Bruce was roped in to “fill the gap.” Check out Bruce’s presentation.
PB-Level PostgreSQL Deployments
The third afternoon session by Chris Travers discussed their transition from using ElasticSearch for data storage—with a poor experience and high maintenance for 1PB over 30 days retention, to a horizontally scaled PostgreSQL cluster perfectly handling 10PB of data. Normally, PostgreSQL comfort levels on a single machine range from several dozen to a few hundred TB. Deployments at the PB scale, especially at 10PB, even within a horizontally scaled cluster, are exceptionally rare. While the practice itself is standard—partitioning and sharding—the scale of data managed is truly impressive.
Highlight: When Hardware and Database Collide
Undoubtedly, the standout presentation of the event, Margo Seltzer’s talk “When Hardware and Database Collide” was not only the most passionate and compelling talk I’ve attended live but also a highlight across all conferences.
Professor Margo Seltzer, formerly of Harvard and now at UBC, a member of the National Academy of Engineering and the creator of BerkeleyDB, delivered a powerful discourse on the core challenges facing databases today. She pinpointed that the bottleneck for databases has shifted from disk I/O to main memory speed. Emerging hardware technologies like HBM and CXL could be the solution, posing new challenges for PostgreSQL hackers to tackle.
This was a refreshing divergence from China’s typically monotonous academic talks, leaving a profound impact and inspiration. Once the conference video is released, I highly recommend checking out her energizing presentation.
WetBar Social
Following Margo’s session, the official Social Event took place at Rogue Kitchen & Wetbar, just a street away from the venue at Waterfront Station, boasting views of the Pacific and iconic Vancouver landmarks.
The informal setting was perfect for engaging with new and old peers. Conversations with notable figures like Devrim, Tomasz, Yurii, and Keith were particularly enriching. As an RPM maintainer, I had an extensive and fruitful discussion with Devrim, resolving many longstanding queries.
The atmosphere was warm and familiar, with many reconnecting after long periods. A couple of beers in, conversations flowed even more freely among fellow PostgreSQL enthusiasts. The event concluded with an invitation from Melanie for a board game session, which I regretfully declined due to my limited English in such interactive settings.
Day 2: Debate, Lunch, and Lighting Talks
Multi-Threading Postgres
The warmth from the previous night’s socializing carried over into the next day, marked by the eagerly anticipated session on “Multi-threaded PostgreSQL,” which was packed to capacity. The discussion, initiated by Heikki, centered on the pros and cons of PostgreSQL’s process and threading models, along with detailed implementation plans and current progress.
The threading model promises numerous benefits: cheaper connections (akin to a built-in connection pool), shared relation and plan caches, dynamic adjustment of shared memory, config changes without restarts, more aggressive Vacuum operations, runtime Explain Analyze, and easier memory usage limits per connection. However, there’s significant opposition, maybe led by Tom Lane, concerned about potential bugs, loss of isolation benefits from the multi-process model, and extensive incompatibilities requiring many extensions to be rewritten.
Heikki laid out a detailed plan to transition to the threading model over five to seven years, aiming for a seamless shift without intermediate states. Intriguingly, he cited Tom Lane’s critical comment in his presentation:
For the record, I think this will be a disaster. There is far too much code that will get broken, largely silently, and much of it is not under our control. – regards, tom lane
Although Tom Lane smiled benignly without voicing any objections, the strongest dissent at the conference came not from him but from an extension maintainer. The elder developer, who maintained several extensions, raised concerns about compatibility, specifically regarding memory allocation and usage. Heikki suggested that extension authors should adapt their work to a new model during a transition grace period of about five years. This suggestion visibly upset the maintainer, who left the meeting in anger.
Given the proposed threading model’s significant impact on the existing extension ecosystem, I’m skeptical about this change. At the conference, I consulted on the threading model with Heikki, Tom Lane, and other hackers. The community’s overall stance is one of curious & cautious observation. So far, the only progress is in PG 17, where the fork-exec-related code has been refactored and global variables marked for future modifications. Any real implementation would likely not occur until at least PG 20+.
Hallway Track
The sessions on the second day were slightly less intense than the first, so many attendees chose the “Hallway Track”—engaging in conversations in the corridors and lobby. I’m usually not great at networking as an introvert, but the vibrant atmosphere quickly drew me in. Eye contact alone was enough to spark conversations, like triggering NPC dialogue in an RPG. I also managed to subtly promote Pigsty to every corner of the PG community.
Despite being a first-timer at PGCon.Dev, I was surprised by the recognition and attention I received, largely thanks to the widely read article, “PostgreSQL is eating the Database world.” Many recognized me by my badge Vonng / Pigsty.
A simple yet effective networking trick is never to underestimate small gifts’ effect. I handed out gold-plated Slonik pins, PostgreSQL’s mascot, which became a coveted item at the conference. Everyone who talked with me received one, and those who didn’t have one were left asking where to get one. LOL
Anyway, I’m glad to have made many new friends and connections.
Multinational Community Lunch
As for lunch, HighGo hosted key participants from the American, European, Japanese, and Chinese PostgreSQL communities at a Cantonese restaurant in Vancouver. The conversation ranged from serious technical discussions to lighter topics. I’ve made acquaintance with Tatsuro Yamada, who gives a talk, “Advice is seldom welcome but efficacious”, and Kyotaro Horiguchi, a core contributor to PostgreSQL known for his work on WAL replication and multibyte string processing and the author of pg_hint_plan.
Another major contributor to the PostgreSQL community, Mark Wong organizes PGUS and has developed a series of PostgreSQL monitoring extensions. He also manages community merchandise like contributor coins, shirts, and stickers. He even handcrafted a charming yarn elephant mascot, which was so beloved that one was sneakily “borrowed” at the last PG Conf US.
Bruce, already a familiar face in the PG Chinese community, Andreas Scherbaum from Germany, organizer of the European PG conferences, and Miao Jian, founder of Han Gao, representing the only Chinese database company at PGCon.Dev, all shared insightful stories and discussions about the challenges and nuances of developing databases in their respective regions.
On returning to the conference venue, I had a conversation with Jan Wieck, a PostgreSQL Hackers Emeritus. He shared his story of participating in the PostgreSQL project from the early days and encouraged me to get more involved in the PostgreSQL community, reminding me its future depends on the younger generation.
Making PG Hacking More Inclusive
At PGCon.Dev, a special session on community building chaired by Robert Hass, featured three new PostgreSQL contributors sharing their journey and challenges, notably the barriers for non-native English speakers, timezone differences, and emotionally charged email communications.
Robert emphasized in a post-conference blog his desire to see more developers from India and Japan rise to senior positions within PostgreSQL’s ranks, noting the underrepresentation from these countries despite their significant developer communities.
While we’re at it, I’d really like to see more people from India and Japan in senior positions within the project. We have very large developer communities from both countries, but there is no one from either of those countries on the core team, and they’re also underrepresented in other senior positions. At the risk of picking specific examples to illustrate a general point, there is no one from either country on the infrastructure team or the code of conduct committee. We do have a few committers from those countries, which is very good, and I was pleased to see Amit Kapila on the 2024.pgconf.dev organizing commitee, but, overall, I think we are still not where we should be. Part of getting people involved is making them feel like they are not alone, and part of it is also making them feel like progression is possible. Let’s try harder to do that.
Frankly, the lack of mention of China in discussions about inclusivity at PGCon.Dev, in favor of India and Japan, left a bittersweet taste. But I think China deserves the snub, given its poor international community engagement.
China has hundreds of “domestic/national” databases, many mere forks of PostgreSQL, yet there’s only a single notable Chinese contributor to PostgreSQL is Richard Guo from PieCloudDB, recently promoted to PG Committer. At the conference, the Chinese presence was minimal, summing up to five attendees, including myself. It’s regrettable that China’s understanding and adoption of PostgreSQL lag behind the global standard by about 10-15 years.
I hope my involvement can bootstrap and enhance Chinese participation in the global PostgreSQL ecosystem, making their users, developers, products, and open-source projects more recognized and accepted worldwide.
Lightning Talks
Yesterday’s event closed with a series of lightning talks—5 minutes max per speaker, or you’re out. Concise and punchy, the session wrapped up 11 topics in just 45 minutes. Keith shared improvements to PG Monitor, and Peter Eisentraut discussed SQL standard updates. But from my perspective, the highlight was Devrim Gündüz’s talk on PG RPMs, which lived up to his promise of a “big reveal” made at the bar the previous night, packing a 75-slide presentation into 5 lively minutes.
Speaking of PostgreSQL, despite being open-source, most users rely on official pre-compiled binaries packages rather than building from source. I maintain 34 RPM extensions for Pigsty, my Postgres distribution, but much of the ecosystem, including over a hundred other extensions, is managed by Devrim from the official PGDG repo. His efforts ensure quality for the world’s most advanced and popular database.
Devrim is a fascinating character — a Turkish native living in London, a part-time DJ, and the maintainer of the PGDG RPM repository, sporting a PostgreSQL logo tattoo. After an engaging chat about the PGDG repository, he shared insights on how extensions are added, highlighting the community-driven nature of PGXN and recent popular additions like pgvector
, (which I made the suggestion haha).
Interestingly, with the latest Pigsty v2.7 release, four of my maintained (packaging) extensions (pgsql-http
, pgsql-gzip
, pg_net
, pg_bigm
) were adopted into the PGDG official repository. Devrim admitted to scouring Pigsty’s extension list for good picks, though he humorously dismissed any hopes for my Rust pgrx extensions making the cut, reaffirming his commitment to not blending Go and Rust plugins into the official repository. Our conversation was so enriching that I’ve committed myself to becoming a “PG Extension Hunter,” scouting and recommending new plugins for official inclusion.
Day 3: Unconference
One of the highlights of PGCon.Dev is the Unconference, a self-organized meeting with no predefined agenda, driven by attendee-proposed topics. On day three, Joseph Conway facilitated the session where anyone could pitch topics for discussion, which were then voted on by participants. My proposal for a Built-in Prometheus Metrics Exporter was merged into a broader Observability topic spearheaded by Jeremy.
The top-voted topics were Multithreading (42 votes), Observability (35 votes), and Enhanced Community Engagement (35 votes). Observability features were a major focus, reflecting the community’s priority. I proposed integrating a contrib monitoring extension in PostgreSQL to directly expose metrics via HTTP endpoint, using pg_exporter
as a blueprint but embedded to overcome the limitations of external components, especially during crash recovery scenarios.
There’s a clear focus on observability among the community. As the author of pg_exporter, I proposed developing a first-party monitoring extension. This extension would integrate Prometheus monitoring endpoints directly into PostgreSQL, exposing metrics via HTTP without needing external components.
The rationale for this proposal is straightforward. While pg_exporter
works well, it’s an external component that adds management complexity. Additionally, in scenarios where PostgreSQL is recovering from a crash and cannot accept new connections, external tools struggle to access internal states. An in-kernel extension could seamlessly capture this information.
The suggested implementation involves a background worker process similar to the bgw_replstatus
extension. This process would listen on an additional port to expose monitoring metrics through HTTP, using pg_exporter as a blueprint. Metrics would primarily be defined via a Collector configuration table, except for a few critical system indicators.
This idea garnered attention from several PostgreSQL hackers at the event. Developers from EDB and CloudNativePG are evaluating whether pg_exporter
could be directly integrated into their distributions as part of their monitoring solutions. And finally, an Observability Special Interest Group (SIG) was formed by attendees interested in observability, planning to continue discussions through a mailing list.
Issue: Support for LoongArch Architecture
During the last two days, I have had some discussions with PG Hackers about some Chinese-specific issues.
A notable suggestion was supporting the LoongArch architecture in the PGDG global repository, which was backed by some enthusiastically local chip and OS manufacturers. Despite the interest, Devrim indicated a “No” due to the lack of support for LoongArch in OS Distro used in the PG community, like CentOS 7, Rocky 8/9, and Debian 10/11/12. Tomasz Rybak was more receptive, noting potential future support if LoongArch runs on Debian 13.
In summary, official PG RPMs might not yet support LoongArch, but APT has a chance, contingent on broader OS support for mainstream open-source Linux distributions.
Issue: Server-side Chinese Character Encoding
At the recent conference, Jeremy Schneider presented an insightful talk on collation rules that resonated with me. He highlighted the pitfalls of not using C.UTF8 for collation, a practice I’ve advocated for based on my own research, and which is detailed in his presentation here.
Post-talk, I discussed further with Jeremy and Peter Eisentraut the nuances of character sets in China, especially the challenges posed by the mandatory GB18030 standard, which PostgreSQL can handle on the client side but not the server side. Also, there are some issues about 20 Chinese characters not working on the convert_to
+ gb18030
encoding mapping.
Closing
The event closed with Jonathan Katz and Melanie Plageman wrapping up an exceptional conference that leaves us looking forward to next year’s PGCon.Dev 2025 in Canada, possibly in Vancouver, Toronto, Ottawa, or Montreal.
Inspired by the engagement at this conference, I’m considering presenting on Pigsty or PostgreSQL observability next year.
Notably, following the conference, Pigsty’s international CDN traffic spiked significantly, highlighting the growing global reach of our PostgreSQL distribution, which really made my day.
Pigsty CDN Traffic Growth after PGCon.Dev 2024
Some slides are available on the official site, and some blog posts about PGCon are here.Dev 2024:
Postgres is eating the database world
PostgreSQL isn’t just a simple relational database; it’s a data management framework with the potential to engulf the entire database realm. The trend of “Using Postgres for Everything” is no longer limited to a few elite teams but is becoming a mainstream best practice.
OLAP’s New Challenger
In a 2016 database meetup, I argued that a significant gap in the PostgreSQL ecosystem was the lack of a sufficiently good columnar storage engine for OLAP workloads. While PostgreSQL itself offers lots of analysis features, its performance in full-scale analysis on larger datasets doesn’t quite measure up to dedicated real-time data warehouses.
Consider ClickBench, an analytics performance benchmark, where we’ve documented the performance of PostgreSQL, its ecosystem extensions, and derivative databases. The untuned PostgreSQL performs poorly (x1050), but it can reach (x47) with optimization. Additionally, there are three analysis-related extensions: columnar store Hydra (x42), time-series TimescaleDB (x103), and distributed Citus (x262).
ClickBench c6a.4xlarge, 500gb gp2 results in relative time
This performance can’t be considered bad, especially compared to pure OLTP databases like MySQL and MariaDB (x3065, x19700); however, its third-tier performance is not “good enough,” lagging behind the first-tier OLAP components like Umbra, ClickHouse, Databend, SelectDB (x3~x4) by an order of magnitude. It’s a tough spot - not satisfying enough to use, but too good to discard.
However, the arrival of ParadeDB and DuckDB changed the game!
ParadeDB’s native PG extension pg_analytics achieves second-tier performance (x10), narrowing the gap to the top tier to just 3–4x. Given the additional benefits, this level of performance discrepancy is often acceptable - ACID, freshness and real-time data without ETL, no additional learning curve, no maintenance of separate services, not to mention its ElasticSearch grade full-text search capabilities.
DuckDB focuses on pure OLAP, pushing analysis performance to the extreme (x3.2) — excluding the academically focused, closed-source database Umbra, DuckDB is arguably the fastest for practical OLAP performance. It’s not a PG extension, but PostgreSQL can fully leverage DuckDB’s analysis performance boost as an embedded file database through projects like DuckDB FDW and pg_quack.
The emergence of ParadeDB and DuckDB propels PostgreSQL’s analysis capabilities to the top tier of OLAP, filling the last crucial gap in its analytic performance.
The Pendulum of Database Realm
The distinction between OLTP and OLAP didn’t exist at the inception of databases. The separation of OLAP data warehouses from databases emerged in the 1990s due to traditional OLTP databases struggling to support analytics scenarios’ query patterns and performance demands.
For a long time, best practice in data processing involved using MySQL/PostgreSQL for OLTP workloads and syncing data to specialized OLAP systems like Greenplum, ClickHouse, Doris, Snowflake, etc., through ETL processes.
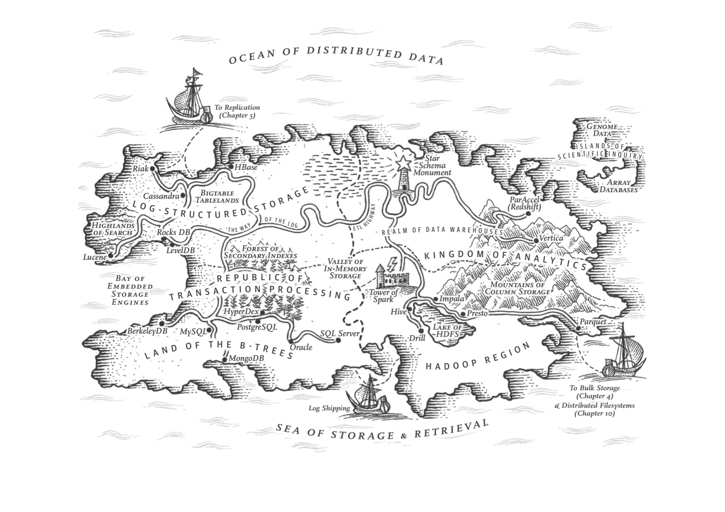
DDIA, Martin Kleppmann, ch3, The republic of OLTP & Kingdom of OLAP
Like many “specialized databases,” the strength of dedicated OLAP systems often lies in performance — achieving 1-3 orders of magnitude improvement over native PG or MySQL. The cost, however, is redundant data, excessive data movement, lack of agreement on data values among distributed components, extra labor expense for specialized skills, extra licensing costs, limited query language power, programmability and extensibility, limited tool integration, poor data integrity and availability compared with a complete DMBS.
However, as the saying goes, “What goes around comes around”. With hardware improving over thirty years following Moore’s Law, performance has increased exponentially while costs have plummeted. In 2024, a single x86 machine can have hundreds of cores (512 vCPU EPYC 9754x2), several TBs of RAM, a single NVMe SSD can hold up to 64TB, and a single all-flash rack can reach 2PB; object storage like S3 offers virtually unlimited storage.
Hardware advancements have solved the data volume and performance issue, while database software developments (PostgreSQL, ParadeDB, DuckDB) have addressed access method challenges. This puts the fundamental assumptions of the analytics sector — the so-called “big data” industry — under scrutiny.
As DuckDB’s manifesto "Big Data is Dead" suggests, the era of big data is over. Most people don’t have that much data, and most data is seldom queried. The frontier of big data recedes as hardware and software evolve, rendering “big data” unnecessary for 99% of scenarios.
If 99% of use cases can now be handled on a single machine with standalone DuckDB or PostgreSQL (and its replicas), what’s the point of using dedicated analytics components? If every smartphone can send and receive texts freely, what’s the point of pagers? (With the caveat that North American hospitals still use pagers, indicating that maybe less than 1% of scenarios might genuinely need “big data.”)
The shift in fundamental assumptions is steering the database world from a phase of diversification back to convergence, from a big bang to a mass extinction. In this process, a new era of unified, multi-modeled, super-converged databases will emerge, reuniting OLTP and OLAP. But who will lead this monumental task of reconsolidating the database field?
PostgreSQL: The Database World Eater
There are a plethora of niches in the database realm: time-series, geospatial, document, search, graph, vector databases, message queues, and object databases. PostgreSQL makes its presence felt across all these domains.
A case in point is the PostGIS extension, which sets the de facto standard in geospatial databases; the TimescaleDB extension awkwardly positions “generic” time-series databases; and the vector extension, PGVector, turns the dedicated vector database niche into a punchline.
This isn’t the first time; we’re witnessing it again in the oldest and largest subdomain: OLAP analytics. But PostgreSQL’s ambition doesn’t stop at OLAP; it’s eyeing the entire database world!
What makes PostgreSQL so capable? Sure, it’s advanced, but so is Oracle; it’s open-source, as is MySQL. PostgreSQL’s edge comes from being both advanced and open-source, allowing it to compete with Oracle/MySQL. But its true uniqueness lies in its extreme extensibility and thriving extension ecosystem.
TimescaleDB survey: what is the main reason you choose to use PostgreSQL
PostgreSQL isn’t just a relational database; it’s a data management framework capable of engulfing the entire database galaxy. Besides being open-source and advanced, its core competitiveness stems from extensibility, i.e., its infra’s reusability and extension’s composability.
The Magic of Extreme Extensibility
PostgreSQL allows users to develop extensions, leveraging the database’s common infra to deliver features at minimal cost. For instance, the vector database extension pgvector, with just several thousand lines of code, is negligible in complexity compared to PostgreSQL’s millions of lines. Yet, this “insignificant” extension achieves complete vector data types and indexing capabilities, outperforming lots of specialized vector databases.
Why? Because pgvector’s creators didn’t need to worry about the database’s general additional complexities: ACID, recovery, backup & PITR, high availability, access control, monitoring, deployment, 3rd-party ecosystem tools, client drivers, etc., which require millions of lines of code to solve well. They only focused on the essential complexity of their problem.
For example, ElasticSearch was developed on the Lucene search library, while the Rust ecosystem has an improved next-gen full-text search library, Tantivy, as a Lucene alternative. ParadeDB only needs to wrap and connect it to PostgreSQL’s interface to offer search services comparable to ElasticSearch. More importantly, it can stand on the shoulders of PostgreSQL, leveraging the entire PG ecosystem’s united strength (e.g., mixed searches with PG Vector) to “unfairly” compete with another dedicated database.
Pigsty has 255 extensions available. And there are 1000+ more in the ecosystem
The extensibility brings another huge advantage: the composability of extensions, allowing different extensions to work together, creating a synergistic effect where 1+1 » 2. For instance, TimescaleDB can be combined with PostGIS for spatio-temporal data support; the BM25 extension for full-text search can be combined with the PGVector extension, providing hybrid search capabilities.
Furthermore, the distributive extension Citus can transparently transform a standalone cluster into a horizontally partitioned distributed database cluster. This capability can be orthogonally combined with other features, making PostGIS a distributed geospatial database, PGVector a distributed vector database, ParadeDB a distributed full-text search database, and so on.
What’s more powerful is that extensions evolve independently, without the cumbersome need for main branch merges and coordination. This allows for scaling — PG’s extensibility lets numerous teams explore database possibilities in parallel, with all extensions being optional, not affecting the core functionality’s reliability. Those features that are mature and robust have the chance to be stably integrated into the main branch.
PostgreSQL achieves both foundational reliability and agile functionality through the magic of extreme extensibility, making it an outlier in the database world and changing the game rules of the database landscape.
Game Changer in the DB Arena
The emergence of PostgreSQL has shifted the paradigms in the database domain: Teams endeavoring to craft a “new database kernel” now face a formidable trial — how to stand out against the open-source, feature-rich Postgres. What’s their unique value proposition?
Until a revolutionary hardware breakthrough occurs, the advent of practical, new, general-purpose database kernels seems unlikely. No singular database can match the overall prowess of PG, bolstered by all its extensions — not even Oracle, given PG’s ace of being open-source and free.
A niche database product might carve out a space for itself if it can outperform PostgreSQL by an order of magnitude in specific aspects (typically performance). However, it usually doesn’t take long before the PostgreSQL ecosystem spawns open-source extension alternatives. Opting to develop a PG extension rather than a whole new database gives teams a crushing speed advantage in playing catch-up!
Following this logic, the PostgreSQL ecosystem is poised to snowball, accruing advantages and inevitably moving towards a monopoly, mirroring the Linux kernel’s status in server OS within a few years. Developer surveys and database trend reports confirm this trajectory.
PostgreSQL has long been the favorite database in HackerNews & StackOverflow. Many new open-source projects default to PostgreSQL as their primary, if not only, database choice. And many new-gen companies are going All in PostgreSQL.
As “Radical Simplicity: Just Use Postgres” says, Simplifying tech stacks, reducing components, accelerating development, lowering risks, and adding more features can be achieved by “Just Use Postgres.” Postgres can replace many backend technologies, including MySQL, Kafka, RabbitMQ, ElasticSearch, Mongo, and Redis, effortlessly serving millions of users. Just Use Postgres is no longer limited to a few elite teams but becoming a mainstream best practice.
What Else Can Be Done?
The endgame for the database domain seems predictable. But what can we do, and what should we do?
PostgreSQL is already a near-perfect database kernel for the vast majority of scenarios, making the idea of a kernel “bottleneck” absurd. Forks of PostgreSQL and MySQL that tout kernel modifications as selling points are essentially going nowhere.
This is similar to the situation with the Linux OS kernel today; despite the plethora of Linux distros, everyone opts for the same kernel. Forking the Linux kernel is seen as creating unnecessary difficulties, and the industry frowns upon it.
Accordingly, the main conflict is no longer the database kernel itself but two directions— database extensions and services! The former pertains to internal extensibility, while the latter relates to external composability. Much like the OS ecosystem, the competitive landscape will concentrate on database distributions. In the database domain, only those distributions centered around extensions and services stand a chance for ultimate success.
Kernel remains lukewarm, with MariaDB, the fork of MySQL’s parent, nearing delisting, while AWS, profiting from offering services and extensions on top of the free kernel, thrives. Investment has flowed into numerous PG ecosystem extensions and service distributions: Citus, TimescaleDB, Hydra, PostgresML, ParadeDB, FerretDB, StackGres, Aiven, Neon, Supabase, Tembo, PostgresAI, and our own PG distro — — Pigsty.
A dilemma within the PostgreSQL ecosystem is the independent evolution of many extensions and tools, lacking a unifier to synergize them. For instance, Hydra releases its own package and Docker image, and so does PostgresML, each distributing PostgreSQL images with their own extensions and only their own. These images and packages are far from comprehensive database services like AWS RDS.
Even service providers and ecosystem integrators like AWS fall short in front of numerous extensions, unable to include many due to various reasons (AGPLv3 license, security challenges with multi-tenancy), thus failing to leverage the synergistic amplification potential of PostgreSQL ecosystem extensions.
Extesion Category Pigsty RDS & PGDG AWS RDS PG Aliyun RDS PG Add Extension Free to Install Not Allowed Not Allowed Geo Spatial PostGIS 3.4.2 PostGIS 3.4.1 PostGIS 3.3.4 Time Series TimescaleDB 2.14.2 Distributive Citus 12.1 AI / ML PostgresML 2.8.1 Columnar Hydra 1.1.1 Vector PGVector 0.6 PGVector 0.6 pase 0.0.1 Sparse Vector PG Sparse 0.5.6 Full-Text Search pg_bm25 0.5.6 Graph Apache AGE 1.5.0 GraphQL PG GraphQL 1.5.0 Message Queue pgq 3.5.0 OLAP pg_analytics 0.5.6 DuckDB duckdb_fdw 1.1 CDC wal2json 2.5.3 wal2json 2.5 Bloat Control pg_repack 1.5.0 pg_repack 1.5.0 pg_repack 1.4.8 Point Cloud PG PointCloud 1.2.5 Ganos PointCloud 6.1 Many important extensions are not available on Cloud RDS (PG 16, 2024-02-29)
Extensions are the soul of PostgreSQL. A Postgres without the freedom to use extensions is like cooking without salt, a giant constrained.
Addressing this issue is one of our primary goals.
Our Resolution: Pigsty
Despite earlier exposure to MySQL Oracle, and MSSQL, when I first used PostgreSQL in 2015, I was convinced of its future dominance in the database realm. Nearly a decade later, I’ve transitioned from a user and administrator to a contributor and developer, witnessing PG’s march toward that goal.
Interactions with diverse users revealed that the database field’s shortcoming isn’t the kernel anymore — PostgreSQL is already sufficient. The real issue is leveraging the kernel’s capabilities, which is the reason behind RDS’s booming success.
However, I believe this capability should be as accessible as free software, like the PostgreSQL kernel itself — available to every user, not just renting from cyber feudal lords.
Thus, I created Pigsty, a battery-included, local-first PostgreSQL distribution as an open-source RDS Alternative, which aims to harness the collective power of PostgreSQL ecosystem extensions and democratize access to production-grade database services.
Pigsty stands for PostgreSQL in Great STYle, representing the zenith of PostgreSQL.
We’ve defined six core propositions addressing the central issues in PostgreSQL database services:
Extensible Postgres, Reliable Infras, Observable Graphics, Available Services, Maintainable Toolbox, and Composable Modules.
The initials of these value propositions offer another acronym for Pigsty:
Postgres, Infras, Graphics, Service, Toolbox, Yours.
Your graphical Postgres infrastructure service toolbox.
Extensible PostgreSQL is the linchpin of this distribution. In the recently launched Pigsty v2.6, we integrated DuckDB FDW and ParadeDB extensions, massively boosting PostgreSQL’s analytical capabilities and ensuring every user can easily harness this power.
Our aim is to integrate the strengths within the PostgreSQL ecosystem, creating a synergistic force akin to the Ubuntu of the database world. I believe the kernel debate is settled, and the real competitive frontier lies here.
- PostGIS: Provides geospatial data types and indexes, the de facto standard for GIS (& pgPointCloud, pgRouting).
- TimescaleDB: Adds time-series, continuous aggregates, distributed, columnar storage, and automatic compression capabilities.
- PGVector: Support AI vectors/embeddings and ivfflat, hnsw vector indexes (& pg_sparse for sparse vectors).
- Citus: Transforms classic master-slave PG clusters into horizontally partitioned distributed database clusters.
- Hydra: Adds columnar storage and analytics, rivaling ClickHouse’s analytic capabilities.
- ParadeDB: Elevates full-text search and mixed retrieval to ElasticSearch levels (& zhparser for Chinese tokenization).
- Apache AGE: Graph database extension, adding Neo4J-like OpenCypher query support to PostgreSQL.
- PG GraphQL: Adds native built-in GraphQL query language support to PostgreSQL.
- DuckDB FDW: Enables direct access to DuckDB’s powerful embedded analytic database files through PostgreSQL (& DuckDB CLI).
- Supabase: An open-source Firebase alternative based on PostgreSQL, providing a complete app development storage solution.
- FerretDB: An open-source MongoDB alternative based on PostgreSQL, compatible with MongoDB APIs/drivers.
- PostgresML: Facilitates classic machine learning algorithms, calling, deploying, and training AI models with SQL.
Developers, your choices will shape the future of the database world. I hope my work helps you better utilize the world’s most advanced open-source database kernel: PostgreSQL.
Read in Pigsty’s Blog | GitHub Repo: Pigsty | Official Website
Technical Minimalism: Just Use PostgreSQL for Everything
This article was originally published by Stephan Schmidt @ KingOfCoders and sparked heated discussions on Hacker News[1]: Using PostgreSQL as a replacement for Kafka, RabbitMQ, ElasticSearch, MongoDB, and Redis is a practical approach that can significantly reduce system complexity and maximize agility.
How to Simplify Complexity and Move Fast: Do Everything with PostgreSQL
Welcome, HN readers. Technology is the art of trade-offs. Using PostgreSQL for everything is also a strategy and compromise. Obviously, we should choose appropriate tools based on needs. In many cases, that tool is Postgres.
In assisting many startups, I’ve observed that far more companies overcomplicate their systems than those who choose overly simple tools. If you have over a million users, more than fifty developers, and you genuinely need Kafka, Spark, and Kubernetes, then go ahead. If you have more systems than developers, using only Postgres is a wise choice.
P.S. Using Postgres for everything doesn’t mean using a single instance for everything ;-)
Simply Put, Everything Can Be Solved with Postgres
It’s easy to invite complexity in, but much harder to show it the door.
However, We Have an Ultimate Simplification Solution
One way to simplify the tech stack, reduce components, speed up development, lower risks, and deliver more features in startups is to “Just Use Postgres for Everything”. Postgres can replace many backend technologies, including Kafka, RabbitMQ, ElasticSearch, MongoDB, and Redis, at least until you reach millions of users.
Use Postgres instead of Redis for caching, using UNLOGGED Tables[3] and storing JSON data in TEXT columns, with stored procedures to add and enforce expiration times, just like Redis does.
Use Postgres as a message queue, employing SKIP LOCKED[4] instead of Kafka (if you only need message queue capabilities).
Use Postgres with the TimescaleDB[5] extension as your data warehouse.
Use PostgreSQL’s JSONB[6] type to store, index, and search JSON documents, replacing MongoDB.
Use Postgres with the pg_cron[7] extension as your scheduled task daemon, executing specific tasks at certain times, such as sending emails or adding events to message queues.
Use Postgres + PostGIS for geospatial queries[8].
Use Postgres for full-text search[9], with ParadeDB replacing ElasticSearch.
Use Postgres to generate JSON in the database[10], eliminating the need for server-side code, directly serving your API.
Use a GraphQL adapter[11] to let PostgreSQL provide GraphQL services.
As I’ve said, Everything Can Be Postgres.
About the Author Stephan
As a CTO, interim CTO, CTO coach, and developer, Stephan has left his mark in the technical departments of many fast-growing startups. He learned programming in a department store around 1981 because he wanted to write video games. Stephan studied computer science at the University of Ulm, specializing in distributed systems and artificial intelligence, and also studied philosophy. When the internet came to Germany in the 90s, he was the first programming employee at several startups. He founded a venture capital-backed startup, handled architecture, processes, and growth challenges in other VC-backed fast-growing startups, held management positions at ImmoScout, and was CTO at an eBay Inc. company. After his wife successfully sold her startup, they moved to the seaside, where Stephan began CTO coaching. You can find him on LinkedIn or follow @KingOfCoders on Twitter.
Translator’s Note
Translator: Feng Ruohang, entrepreneur and PostgreSQL expert, cloud-native advocate, author of Pigsty, an open-source PostgreSQL RDS alternative that’s ready to use out of the box.
Using Postgres for everything is not a pipe dream but a best practice that’s gaining popularity. I’m very pleased about this: I saw this potential back in 2016[12] and chose to dive in, and things have developed exactly as hoped.
Tantan, where I previously worked, was a pioneer on this path - PostgreSQL for Everything. This Chinese internet app, founded by a Swedish team, used PostgreSQL at a scale and complexity that was second to none in China. Tantan’s technical architecture choices were inspired by Instagram - or even more radical, with almost all business logic implemented in PostgreSQL stored procedures (including recommendation algorithms with 100ms latency!).
Tantan’s entire system architecture was designed and built around PostgreSQL. With millions of daily active users, millions of global DB-TPS, and hundreds of TB of data, the data component only used PostgreSQL. It wasn’t until approaching ten million daily active users that they began architectural adjustments to introduce independent data warehouses, message queues, and caching. In 2017, we didn’t even use Redis for caching; 2.5 million TPS was handled directly by PostgreSQL across over a hundred servers. Message queues were also implemented in PostgreSQL, and early/mid-stage data analysis was handled by a dedicated PG cluster of several dozen TB. We had long practiced the philosophy of “using PostgreSQL for everything” and reaped many benefits.
There’s a second part to this story - the subsequent “microservices transformation” brought massive complexity, eventually bogging down the system. This made me even more certain from another angle - I deeply miss that simple, reliable, efficient, and agile state when we used PostgreSQL for everything.
PostgreSQL is not just a simple relational database but an abstract framework for data management with the potential to encompass everything and devour the entire database world. Ten years ago, this was merely potential and possibility; ten years later, it has materialized into real influence. I’m glad to have witnessed this process and helped push it forward.
Further Reading
PGSQL x Pigsty: The Database Swiss Army Knife is Here
ParadeDB: A New Player in the PG Ecosystem
FerretDB: PostgreSQL Dressed as MongoDB
AI Large Models and Vector Databases with PGVECTOR
How Powerful is PostgreSQL Really?
PostgreSQL: The World’s Most Successful Database
Why is PostgreSQL the Most Successful Database?
Why Does PostgreSQL Have a Bright Future?
Better Open Source RDS Alternative: Pigsty
References
[1]
Just use Postgres for everything: https://news.ycombinator.com/item?id=33934139[2]
Technical Minimalism Manifesto: https://www.radicalsimpli.city/[3]
UNLOGGED Table: https://www.compose.com/articles/faster-performance-with-unlogged-tables-in-postgresql/[4]
SKIP LOCKED: https://www.enterprisedb.com/blog/what-skip-locked-postgresql-95[5]
Timescale: https://www.timescale.com/[6]
JSONB: https://scalegrid.io/blog/using-jsonb-in-postgresql-how-to-effectively-store-index-json-data-in-postgresql/[7]
pg_cron: https://github.com/citusdata/pg_cron[8]
Geospatial Queries: https://postgis.net/[9]
Full-Text Search: https://supabase.com/blog/postgres-full-text-search-vs-the-rest[10]
Generate JSON in Database: https://www.amazingcto.com/graphql-for-server-development/[11]
GraphQL Adapter: https://graphjin.com/[12]
What are PostgreSQL’s Advantages over MySQL?: https://www.zhihu.com/question/20010554/answer/94999834
ParadeDB: ElasticSearch Alternative in PG Ecosystem
ParadeDB: A New Player in the PostgreSQL Ecosystem
YC S23 invested in an exciting new project called ParadeDB. Their slogan? “Postgres for Search & Analytics — Modern Elasticsearch Alternative built on Postgres.” In essence, it’s PostgreSQL optimized for search and analytics, aiming to be a drop-in replacement for Elasticsearch.
The PostgreSQL ecosystem continues to flourish with innovative extensions and derivatives. We’ve already seen FerretDB as an open-source MongoDB alternative, Babelfish for SQL Server, Supabase for Firebase, and NocoDB for AirTable. Now, we can add ParadeDB to the list as an open-source Elasticsearch alternative.
ParadeDB consists of three PostgreSQL extensions: pg_bm25
, pg_analytics
, and pg_sparse
. Each extension can be used independently. I’ve packaged these extensions (v0.5.6) and will include them by default in the next Pigsty release, making them available out of the box for users.
I’ve translated ParadeDB’s official website introduction and four blog posts to introduce this rising star in the PostgreSQL ecosystem. Today’s post is the first one — an overview.
ParadeDB
We’re thrilled to introduce ParadeDB: a PostgreSQL database optimized for search scenarios. ParadeDB is the first PostgreSQL build designed to be an Elasticsearch alternative, offering lightning-fast full-text search, semantic search, and hybrid search capabilities on PostgreSQL tables.
What Problem Does ParadeDB Solve?
For many organizations, search remains an unsolved problem — despite giants like Elasticsearch in the market. Most developers who’ve worked with Elasticsearch know the pain of running, tuning, and managing it. While other search engine services exist, integrating external services with existing databases introduces complex challenges and costs associated with rebuilding indexes and data replication.
Developers seeking a unified source of truth and search capabilities have turned to PostgreSQL. While PG offers basic full-text search through tsvector
and semantic search through pgvector
, these tools fall short when dealing with large tables or complex queries:
- Sorting and keyword searches on large tables are painfully slow
- No BM25 scoring support
- No hybrid search capabilities combining vector and full-text search
- No real-time search — data must be manually reindexed or re-embedded
- Limited support for complex queries like faceting or relevance tuning
We’ve seen many engineering teams reluctantly layer Elasticsearch on top of PostgreSQL, only to abandon it due to its bloated nature, high costs, or complexity. We wondered: what if PostgreSQL had Elasticsearch-level search capabilities built-in? This would eliminate the dilemma of choosing between using PostgreSQL with limited search capabilities or maintaining separate services for source of truth and search.
Who Is ParadeDB For?
While Elasticsearch serves a wide range of use cases, we’re not trying to cover everything — at least not yet. We’re focusing on core scenarios, specifically serving users who want to perform search within PostgreSQL. ParadeDB is ideal for you if:
- You want to use PostgreSQL as your single source of truth and hate data replication between multiple services
- You need to perform full-text search on massive documents stored in PostgreSQL without compromising performance and scalability
- You want to combine ANN/similarity search with full-text search for more precise semantic matching
ParadeDB Product Overview
ParadeDB is a fully managed Postgres database with indexing and search capabilities for PostgreSQL tables that you won’t find in any other PostgreSQL provider:
Feature | Description |
---|---|
BM25 Full-Text Search | Full-text search supporting boolean, fuzzy, boosting, and keyword queries. Search results are scored using the BM25 algorithm. |
Faceted Search | PostgreSQL columns can be defined as facets for easy bucketing and metric collection. |
Hybrid Search | Search results can be scored considering both semantic relevance (vector search) and text relevance (BM25). |
Distributed Search | Tables can be sharded for parallel query acceleration. |
Generative Search | PostgreSQL columns can be fed into large language models (LLMs) for automatic summarization, classification, or text generation. |
Real-time Search | Text indexes and vector columns automatically stay in sync with underlying data. |
Unlike managed services like AWS RDS, ParadeDB is a PostgreSQL extension plugin that requires no setup, integrates with the entire PG ecosystem, and is fully customizable. ParadeDB is open-source (AGPLv3) and provides a simple Docker Compose template for developers who need to self-host or customize.
How ParadeDB Is Built
At its core, ParadeDB is a standard Postgres database with custom extensions written in Rust that introduce enhanced search capabilities.
ParadeDB’s search engine is built on top of Tantivy, an open-source Rust search library inspired by Apache Lucene. Its indexes are stored natively in PostgreSQL as PG indexes, eliminating the need for cumbersome data replication/ETL work while maintaining transaction ACID guarantees.
ParadeDB introduces a new extension to the Postgres ecosystem: pg_bm25
. This extension implements Rust-based full-text search in PostgreSQL using the BM25 scoring algorithm. ParadeDB comes pre-installed with this extension.
What’s Next?
ParadeDB’s managed cloud version is currently in PrivateBeta. We aim to launch a self-service cloud platform in early 2024. If you’d like to access the PrivateBeta version during this period, join our waitlist.
Our core team is focused on developing the open-source version of ParadeDB, which will be released in Winter 2023.
We’re building in public and are excited to share ParadeDB with the community. Stay tuned for future blog posts where we’ll dive deeper into the fascinating technical challenges behind ParadeDB.
The Astonishing Scalability of PostgreSQL
This article outlines how Cloudflare uses 15 PostgreSQL clusters to scale up to 55 million requests per second.
In July 2009, in California, USA, a startup team created a Content Delivery Network (CDN) called Cloudflare to accelerate internet requests, making web access more stable and faster. While facing various challenges in their early development, their growth rate was astounding.
Global overview of internet traffic
Today, they handle 20% of all internet traffic, processing 55 million HTTP requests per second. And they accomplish this with just 15 PostgreSQL clusters.
Cloudflare uses PostgreSQL to store service metadata and handle OLTP workloads. However, supporting tenants with different types of workloads on the same cluster presents a challenge. A cluster is a group of database servers, while a tenant is an isolated data space dedicated to a specific user or group of users.
PostgreSQL Scalability
Here’s how they pushed PostgreSQL’s scalability to its limits.
1. Contention
Most clients compete for Postgres connections. But Postgres connections are expensive because each connection is a separate OS-level process. And since each tenant has a unique workload type, it’s difficult to create a global threshold for rate limiting.
Moreover, manually restricting misbehaving tenants is a massive undertaking. A tenant might initiate an extremely expensive query, blocking queries from neighboring tenants and starving them. Once a query reaches the database server, isolating it becomes challenging.
Connection pooling with PgBouncer
Therefore, they use PgBouncer as a connection pool in front of Postgres. PgBouncer acts as a TCP proxy, pooling Postgres connections. Tenants connect to PgBouncer rather than directly to Postgres. This limits the number of Postgres connections and prevents connection starvation.
Additionally, PgBouncer avoids the high overhead of creating and destroying database connections by using persistent connections and is used to throttle tenants that initiate high-cost queries at runtime.
2. Thundering Herd
The Thundering Herd problem occurs when many clients query the server simultaneously, leading to database performance degradation.
When applications are redeployed, their state initializes, and the applications create many database connections at once. Thus, when tenants compete for Postgres connections, it triggers the thundering herd phenomenon. Cloudflare uses PgBouncer to limit the number of Postgres connections created by specific tenants.
3. Performance
Cloudflare doesn’t run PostgreSQL in the cloud but uses bare-metal physical machines without any virtualization overhead to achieve the best performance.
Load balancing traffic between database instances
Cloudflare uses HAProxy as a layer-four load balancer. PgBouncer forwards queries to HAProxy, and the HAProxy load balancer balances traffic between the cluster’s primary instance and read-only replicas.
4. Concurrency
Performance decreases when many tenants make concurrent queries.
Congestion control throttling algorithm
Therefore, Cloudflare uses the TCP Vegas congestion control algorithm to throttle tenants. This algorithm works by first sampling each tenant’s transaction round-trip time (RTT) to Postgres, then continuously adjusting the connection pool size as long as the RTT doesn’t degrade, enabling throttling before resources are exhausted.
5. Queuing
Cloudflare queues queries at the PgBouncer level. The order of queries in the queue depends on their historical resource usage—in other words, queries requiring more resources are placed at the end of the queue.
Using priority queues to order queries
Cloudflare only enables priority queuing during peak traffic to prevent resource starvation. In other words, during normal traffic, queries won’t always end up at the back of the queue.
This approach improves latency for the vast majority of queries, though tenants initiating high-cost queries during peak traffic will observe higher latency.
6. High Availability
Cloudflare uses Stolon cluster management for Postgres high availability.
Using Stolon for database high availability
Stolon can be used to set up Postgres master-slave replication and is responsible for electing a Postgres cluster leader (primary) and handling failover when problems arise.
Each database cluster here replicates to two regions, with three instances in each region.
Write requests are routed to the primary in the main region, then asynchronously replicated to the secondary region, while read requests are routed to the secondary region for processing.
Cloudflare conducts component interconnectivity tests to actively detect network partition issues, performs chaos testing to optimize system resilience, and configures redundant network switches and routers to avoid network partitioning.
When failover completes and the primary instance comes back online, they use the pg_rewind tool to replay missed write changes to resynchronize the old primary with the cluster.
Cloudflare has over 100 Postgres primary and replica instances combined. They use a combination of OS resource management, queuing theory, congestion control algorithms, and even PostgreSQL metrics to achieve PostgreSQL scalability.
Evaluation and Discussion
This is a valuable experience-sharing article, mainly introducing how to use PgBouncer to solve PostgreSQL’s scalability issues. Fifty million QPS + 20% of internet traffic sounds like a significant scale. Although from a PostgreSQL expert’s perspective, the practices described here might seem somewhat basic and rudimentary, the article raises a meaningful question—PostgreSQL’s scalability.
Current State of PostgreSQL Scalability
PostgreSQL has a reputation for vertical and horizontal scaling capabilities. For read requests, PostgreSQL has no scalability issues—since reads and writes don’t block each other, the throughput limit for read-only queries grows almost linearly with invested resources (CPU), whether by vertically increasing CPU/memory or horizontally expanding with replica instances.
PostgreSQL’s write scalability isn’t as strong as its read capabilities. Single-machine WAL write/replay speed reaches a software bottleneck at 100 MB/s to 300 MB/s—but for regular production OLTP loads, this is already a substantial value. As a reference, an application like Tantan, with 200 million users and 10 million daily active users, has a structured data rate for all database writes of around 120 MB/s. The PostgreSQL community is also discussing ways to expand this bottleneck through DIO/AIO and parallel WAL replay. Users can also consider using Citus or other sharding middleware to achieve write scalability.
In terms of capacity, PostgreSQL’s scalability primarily depends on disk space and doesn’t have inherent bottlenecks. With today’s NVMe SSDs offering 64TB per card, supporting hundreds of terabytes of data capacity is no problem when combined with compression cards. Even larger capacities can be supported using RAID or multiple tablespaces. The community has reported numerous OLTP instances in the hundreds of terabytes range, with occasional instances at the petabyte level. The challenges with large instances are mainly in backup management and space maintenance, not performance.
In the past, a notable criticism of PostgreSQL’s scalability was its support for massive connections (significantly improved after PostgreSQL 14). PostgreSQL uses a multi-process architecture like Oracle by default. This design provides better reliability but can be a bottleneck when facing massive high-concurrency scenarios.
In internet scenarios, database access patterns primarily involve massive short connections: creating a connection for a query and destroying it after execution—PHP used to do this, making it compatible with MySQL, which uses a thread model. But for PostgreSQL, massive backend processes and frequent process creation/destruction waste considerable hardware and software resources, making its performance somewhat inadequate in these scenarios.
Connection Pooling — Solving High Concurrency Issues
PostgreSQL recommends a default connection count of about twice the number of CPU cores, typically appropriate in the range of a few dozen to a few hundred. In internet scenarios with thousands or tens of thousands of client connections directly connecting to PostgreSQL, there would be significant additional overhead. Connection pooling emerged to solve this problem—it can be said that connection pooling is a must-have for using PostgreSQL in internet scenarios, capable of transforming the ordinary into the extraordinary.
Note that PostgreSQL is not incapable of high throughput; the key issue is the number of concurrent connections. In “How Strong is PG Performance,” we achieved a sysbench point query throughput peak of 2.33 million on a 92 vCPU server using about 96 connections. Once resources are exceeded, this maximum throughput begins to slowly decline as concurrency further increases.
Using connection pooling has several significant benefits: First, tens of thousands of client connections can be pooled and buffered down to a few active server connections (using transaction-level connection pooling), greatly reducing the number of processes and overhead on the operating system, and avoiding the overhead of process creation and destruction. Second, concurrent contention is greatly reduced due to the reduction in active connections, further optimizing performance. Third, sudden load peaks will queue at the connection pool instead of overwhelming the database, reducing the probability of cascading failures and improving system stability.
Performance and Bottlenecks
I had many best practices with PgBouncer at Tantan. We had a core database cluster with 500,000 QPS, 20,000 client connections on the primary, and a write TPS of about 50,000. Such a load would immediately overwhelm Postgres if directed straight to it. Therefore, between the application and the database, there was a PgBouncer connection pooling middleware. All twenty thousand client connections, after transaction pooling, only required 5-8 active server connections to support all requests, with a CPU utilization of about 20%—a tremendous performance improvement.
PgBouncer is a lightweight connection pool that can be deployed on either the user side or the database side. PgBouncer itself, due to its single-process mode, has a QPS/TPS bottleneck of about 30,000-50,000. Therefore, to avoid PgBouncer’s single point of failure and bottleneck, we used 4 idempotent PgBouncer instances on the core primary and evenly distributed traffic through HAProxy to these four PgBouncer instances before reaching the database primary. But for most scenarios, a single PgBouncer process’s capability to handle 30,000 QPS is more than sufficient.
Management Flexibility
A huge advantage of PgBouncer is that it can provide query response time metrics (RT) at the User/Database/Instance level. This is a core metric for performance measurement, and for older versions of PostgreSQL, statistics in PgBouncer were the only way to obtain such data. Although users can obtain query group RT through the pg_stat_statements
extension, and PostgreSQL 14 and later can obtain database-level session active time to calculate transaction RT, and the newly emerged eBPF can also accomplish this, the performance monitoring data provided by PgBouncer remains a very important reference for database management.
The PgBouncer connection pool not only provides performance improvements but also offers handles for fine-grained management. For example, in online database migration without downtime, if online traffic completely accesses through the connection pool, you can simply redirect read/write traffic from the old cluster to the new one by modifying the PgBouncer configuration file, without even requiring immediate participation from the business side to change configurations and restart services. You can also, like in Cloudflare’s example above, modify Database/User parameters in the connection pool to implement throttling capabilities. If a database tenant behaves poorly, affecting the entire shared cluster, administrators can easily implement throttling and blocking capabilities in PgBouncer.
Other Alternatives
There are other connection pool products in the PostgreSQL ecosystem. PGPool-II, which emerged around the same time as PgBouncer, was once a strong competitor: it provided more powerful load balancing/read-write splitting capabilities and could fully utilize multi-core capabilities, but it had invasiveness to the PostgreSQL database itself—requiring extension installation to use, and once had significant performance penalties (30%). So in the connection pool battle, the simple and lightweight PgBouncer became the winner, occupying the mainstream ecological niche of PG connection pools.
Besides PgBouncer, new PostgreSQL connection pool projects continue to emerge, such as Odyssey, pgcat, pgagroal, ZQPool, etc. I very much look forward to a high-performance/more user-friendly in-place replacement fully compatible with PgBouncer.
Additionally, many programming language standard library database drivers now have built-in connection pools, plus PostgreSQL 14’s improvements have reduced the overhead of multiple processes. And with the exponential growth of hardware performance (there are now servers with 512 vCPUs, and memory is no longer a scarce resource), sometimes not using a connection pool and directly handling a few thousand connections is also a viable option.
Can I Use Cloudflare’s Practices?
With the continuous improvement of hardware performance, the ongoing optimization of software architecture, and the gradual popularization of management best practices—high availability, high concurrency, and high performance (scalability) are old topics for internet companies and basically not new technologies anymore.
For example, nowadays, even a junior DBA/ops person, as long as they use Pigsty to deploy a PostgreSQL cluster, can easily achieve this, including the PgBouncer connection pool mentioned by Cloudflare, and Patroni, which has replaced the high availability component Stolon, are all ready to use out of the box. As long as the hardware meets requirements, handling massive concurrent requests in the millions is not a dream.
At the beginning of this century, an Apache server could only handle a miserable one or two hundred concurrent requests. Even the most excellent software could hardly handle tens of thousands of concurrent requests—there was a famous C10K high concurrency problem in the industry; anyone who could achieve thousands of concurrent connections was an industry expert. But with the successive emergence of Epoll and Nginx in 2003/2004, “high concurrency” was no longer a difficult problem—any novice who learned to configure Nginx could achieve what masters could not even dream of a few years ago—as Swedish Marcus says in “Cloud Providers’ View of Customers: Poor, Idle, and Lacking Love”
This is just like now any novice can use Nginx to achieve the massive web requests and high concurrency that masters using httpd could not even dream of before. PostgreSQL’s scalability has also entered thousands of households with the popularization of PgBouncer.
For example, in Pigsty, PgBouncer instances are deployed 1:1 for all PostgreSQL by default, using transaction pooling mode and incorporated into monitoring. And the default Primary and Replica services also access the Postgres database through PgBouncer. Users don’t need to worry too much about details related to PgBouncer—for instance, PgBouncer’s databases and users are automatically maintained when creating Postgres databases/users through scripts. Some common configuration considerations and pitfalls are also avoided in the preset configuration templates, striving to achieve out-of-the-box usability.
Of course, for non-internet scenario applications, PgBouncer is not a must-have. And the default Transaction Pooling, although excellent in performance, comes at the cost of sacrificing some session-level functionality. So you can also configure Primary/Replica services to connect directly to Postgres, bypassing PgBouncer; or use the Session Pooling mode with the best compatibility.
Overall, PgBouncer is indeed a very practical tool in the PostgreSQL ecosystem. If your system has high requirements for PostgreSQL client concurrent connections, be sure to try this middleware when testing performance.
Original article: How Cloudflare Used 15 PG Clusters to Support 55M QPS |
PostgreSQL Crowned Database of the Year 2024!
Today, the renowned database popularity ranking site DB-Engines announced its Database of the Year for 2024. PostgreSQL has claimed this honor for the fifth time. Of course, PostgreSQL was also crowned Database of the Year in 2023, 2019, 2018, and 2017. If Snowflake hadn’t stolen the spotlight in 2020 and 2021, pushing PostgreSQL to second place, we’d be looking at a seven-year winning streak.
PostgreSQL Claims the Crown as “Database Management System of the Year” for 2024
DB-Engines has officially announced that PostgreSQL has once again been crowned “DBMS of the Year” - marking its second consecutive win and fifth overall triumph following its dominance in 2017, 2018, 2019, and 2023. Snowflake secured the runner-up position with its impressive momentum, while Microsoft claimed third place. Over the past year, PostgreSQL has emerged as the most popular database management system, surpassing all other 423 databases monitored by DB-Engines.
Rewind nearly 35 years to when “Postgres” first burst onto the scene. Since then, PostgreSQL has continuously evolved to keep pace with database technology trends, growing more powerful while maintaining rock-solid stability. PostgreSQL 17, released in September 2024, pushed this “evergreen tree” to new heights with enhanced performance and expanded replication capabilities. In today’s open-source landscape, PostgreSQL stands as a shining example of how to maintain both popularity and technical excellence.
Here’s where it gets interesting: Looking at DB-Engines’ popularity score increments this year, Snowflake gained 28 points while PostgreSQL increased by 14.5 points. Following their usual calculation rules for Database of the Year (based on popularity scores from January 2024 to January 2025), Snowflake should have technically claimed the title. Yet, the editors still chose PostgreSQL as Database of the Year.
Of course, I don’t believe DB-Engines’ editors would make such an elementary mathematical error. To be frank, given PostgreSQL’s remarkable growth and impressive metrics in 2024, if they hadn’t named PG as Database of the Year, it would have only damaged the credibility of their rankings (much like how a gaming publication would lose face if they didn’t recognize Breath of the Wild or The Witcher 3 as Game of the Year). I suspect the editors had no choice but to crown PostgreSQL as No. 1, even if it meant going against their own metrics.
To be honest, compared to first-hand, large-sample surveys like the StackOverflow Annual Global Developer Survey, popularity rankings like DB-Engines should only be taken as rough references. While their standardized methodology makes them valuable for tracking a database’s historical popularity trends (vertical comparability), they’re less reliable for comparing different databases head-to-head (horizontal comparability).
Original DB-Engines Blog Post
PostgreSQL Named Database Management System of the Year 2024
By Tom Russell, January 13, 2025
https://db-engines.com/en/blog_post/109
DB-Engines has officially announced that PostgreSQL has been crowned “DBMS of the Year” - marking its second consecutive win and fifth overall triumph following its dominance in 2017, 2018, 2019, and 2023. Snowflake secured the runner-up position with its impressive momentum, while Microsoft claimed third place. Over the past year, PostgreSQL has emerged as the most popular database management system, surpassing all other 423 databases monitored by DB-Engines.
Rewind nearly 35 years to when “Postgres” first burst onto the scene. Since then, PostgreSQL has continuously evolved to keep pace with database technology trends, growing more powerful while maintaining rock-solid stability. PostgreSQL 17, released in September 2024, pushed this “evergreen tree” to new heights with enhanced performance and expanded replication capabilities. In today’s open-source landscape, PostgreSQL stands as a shining example of how to maintain both popularity and technical excellence.
Snowflake, this year’s runner-up, is much more than just a “snowflake” - it’s a cloud-based data warehouse service that has attracted a massive following with its unique architecture separating storage and compute. Combined with multi-cloud support and data sharing capabilities, it has become an industry hot spot. Snowflake’s rising rankings clearly demonstrate its growing influence in the field.
Microsoft, securing third place, remains a “veteran” in the database arena: Azure SQL Database offers fully managed relational database services with AI-driven performance optimization and elastic scaling, while SQL Server bridges the gap between on-premises and cloud with hybrid cloud capabilities. Microsoft’s continuous database innovations, coupled with its comprehensive data service ecosystem, make it a force to be reckoned with.
PostgreSQL Convention 2024
- Background
- 0x01 Naming Convention
- 0x01 Design Convention
- 0x01 Query Convention
- 0x01 Admin Convention
Roughly translated from PostgreSQL Convention 2024 with Google.
0x00 Background
No Rules, No Lines
The functions of PostgreSQL are very powerful, but to use PostgreSQL well requires the cooperation of backend, operation and maintenance, and DBA.
This article has compiled a development/operation and maintenance protocol based on the principles and characteristics of the PostgreSQL database, hoping to reduce the confusion you encounter when using the PostgreSQL database: hello, me, everyone.
The first version of this article is mainly for PostgreSQL 9.4 - PostgreSQL 10. The latest version has been updated and adjusted for PostgreSQL 15/16.
0x01 naming convention
There are only two hard problems in computer science: cache invalidation and naming .
Generic naming rules (Generic)
- This rule applies to all objects in the database , including: library names, table names, index names, column names, function names, view names, serial number names, aliases, etc.
- The object name must use only lowercase letters, underscores, and numbers, and the first letter must be a lowercase letter.
- The length of the object name must not exceed 63 characters, and the naming
snake_case
style must be uniform. - The use of SQL reserved words is prohibited, use
select pg_get_keywords();
to obtain a list of reserved keywords. - Dollar signs are prohibited
$
, Chinese characters are prohibited, and do notpg
begin with . - Improve your wording taste and be honest and elegant; do not use pinyin, do not use uncommon words, and do not use niche abbreviations.
Cluster naming rules (Cluster)
- The name of the PostgreSQL cluster will be used as the namespace of the cluster resource and must be a valid DNS domain name without any dots or underscores.
- The cluster name should start with a lowercase letter, contain only lowercase letters, numbers, and minus signs, and conform to the regular expression:
[a-z][a-z0-9-]*
. - PostgreSQL database cluster naming usually follows a three-part structure:
pg-<biz>-<tld>
. Database type/business name/business line or environment biz
The English words that best represent the characteristics of the business should only consist of lowercase letters and numbers, and should not contain hyphens-
.- When using a backup cluster to build a delayed slave database of an existing cluster,
biz
the name should be<biz>delay
, for examplepg-testdelay
. - When branching an existing cluster, you can
biz
add a number at the end of : for example,pg-user1
you can branch frompg-user2
,pg-user3
etc. - For horizontally sharded clusters,
biz
the name should includeshard
and be preceded by the shard number, for examplepg-testshard1
,pg-testshard2
,… <tld>
It is the top-level business line and can also be used to distinguish different environments: for example-tt
,-dev
,-uat
,-prod
etc. It can be omitted if not required.
Service naming rules (Service)
- Each PostgreSQL cluster will provide 2 to 6 types of external services, which use fixed naming rules by default.
- The service name is prefixed with the cluster name and the service type is suffixed, for example
pg-test-primary
,pg-test-replica
. - Read-write services are uniformly
primary
named with the suffix, and read-only services are uniformlyreplica
named with the suffix. These two services are required. - ETL pull/individual user query is
offline
named with the suffix, and direct connection to the main database/ETL write isdefault
named with the suffix, which is an optional service. - The synchronous read service is
standby
named with the suffix, and the delayed slave library service isdelayed
named with the suffix. A small number of core libraries can provide this service.
Instance naming rules (Instance)
- A PostgreSQL cluster consists of at least one instance, and each instance has a unique instance number assigned from zero or one within the cluster.
- The instance name
-
is composed of the cluster name + instance number with hyphens , for example:pg-test-1
,pg-test-2
. - Once assigned, the instance number cannot be modified until the instance is offline and destroyed, and cannot be reassigned for use.
- The instance name will be used as a label for monitoring system data
ins
and will be attached to all data of this instance. - If you are using a host/database 1:1 exclusive deployment, the node Hostname can use the database instance name.
Database naming rules (Database)
- The database name should be consistent with the cluster and application, and must be a highly distinguishable English word.
- The naming is
<tld>_<biz>
constructed in the form of ,<tld>
which is the top-level business line. It can also be used to distinguish different environments and can be omitted if not used. <biz>
For a specific business name, for example,pg-test-tt
the cluster can use the library namett_test
ortest
. This is not mandatory, i.e. it is allowed to create<biz>
other databases with different cluster names.- For sharded libraries,
<biz>
the section mustshard
end with but should not contain the shard number, for examplepg-testshard1
,pg-testshard2
bothtestshard
should be used. - Multiple parts use
-
joins. For example:<biz>-chat-shard
,<biz>-payment
etc., no more than three paragraphs in total.
Role naming convention (Role/User)
dbsu
There is only one database super user :postgres
, the user used for streaming replication is namedreplicator
.- The users used for monitoring are uniformly named
dbuser_monitor
, and the super users used for daily management are:dbuser_dba
. - The business user used by the program/service defaults to using
dbuser_<biz>
as the username, for exampledbuser_test
. Access from different services should be differentiated using separate business users. - The database user applied for by the individual user agrees to use
dbp_<name>
, where isname
the standard user name in LDAP. - The default permission group naming is fixed as:
dbrole_readonly
,dbrole_readwrite
,dbrole_admin
,dbrole_offline
.
Schema naming rules (Schema)
- The business uniformly uses a global
<prefix>
as the schema name, as short as possible, and is set tosearch_path
the first element by default. <prefix>
You must not usepublic
,monitor
, and must not conflict with any schema name used by PostgreSQL extensions, such as:timescaledb
,citus
,repack
,graphql
,net
,cron
,… It is not appropriate to use special names:dba
,trash
.- Sharding mode naming rules adopt:
rel_<partition_total_num>_<partition_index>
. The middle is the total number of shards, which is currently fixed at 8192. The suffix is the shard number, counting from 0. Such asrel_8192_0
,…,,,rel_8192_11
etc. - Creating additional schemas, or using
<prefix>
schema names other than , will require R&D to explain their necessity.
Relationship naming rules (Relation)
- The first priority for relationship naming is to have clear meaning. Do not use ambiguous abbreviations or be too lengthy. Follow general naming rules.
- Table names should use plural nouns and be consistent with historical conventions. Words with irregular plural forms should be avoided as much as possible.
- Views use
v_
as the naming prefix, materialized views usemv_
as the naming prefix, temporary tables usetmp_
as the naming prefix. - Inherited or partitioned tables should be prefixed by the parent table name and suffixed by the child table attributes (rules, shard ranges, etc.).
- The time range partition uses the starting interval as the naming suffix. If the first partition has no upper bound, the R&D will specify a far enough time point: grade partition:
tbl_2023
, month-level partitiontbl_202304
, day-level partitiontbl_20230405
, hour-level partitiontbl_2023040518
. The default partition_default
ends with . - The hash partition is named with the remainder as the suffix of the partition table name, and the list partition is manually specified by the R&D team with a reasonable partition table name corresponding to the list item.
Index naming rules (Index)
- When creating an index, the index name should be specified explicitly and consistent with the PostgreSQL default naming rules.
- Index names are prefixed with the table name, primary key indexes
_pkey
end with , unique indexes_key
end with , ordinary indexes end_idx
with , and indexes used forEXCLUDED
constraints_excl
end with . - When using conditional index/function index, the function and condition content used should be reflected in the index name. For example
tbl_md5_title_idx
,tbl_ts_ge_2023_idx
, but the length limit cannot be exceeded.
Field naming rules (Attribute)
- It is prohibited to use system column reserved field names:
oid
,xmin
,xmax
,cmin
,cmax
,ctid
. - Primary key columns are usually named with
id
or asid
a suffix. - The conventional name is the creation time field
created_time
, and the conventional name is the last modification time field.updated_time
is_
It is recommended to use , etc. as the prefix for Boolean fieldshas_
.- Additional flexible JSONB fields are fixed using
extra
as column names. - The remaining field names must be consistent with existing table naming conventions, and any field naming that breaks conventions should be accompanied by written design instructions and explanations.
Enumeration item naming (Enum)
- Enumeration items should be used by default
camelCase
, but other styles are allowed.
Function naming rules (Function)
- Function names start with verbs:
select
,insert
,delete
,update
,upsert
,create
,…. - Important parameters can be reflected in the function name through
_by_ids
the_by_user_ids
suffix of. - Avoid function overloading and try to keep only one function with the same name.
BIGINT/INTEGER/SMALLINT
It is forbidden to overload function signatures through integer types such as , which may cause ambiguity when calling.- Use named parameters for variables in stored procedures and functions, and avoid positional parameters (
$1
,$2
,…). - If the parameter name conflicts with the object name, add before the parameter
_
, for example_user_id
.
Comment specifications (Comment)
- Try your best to provide comments (
COMMENT
) for various objects. Comments should be in English, concise and concise, and one line should be used. - When the object’s schema or content semantics change, be sure to update the annotations to keep them in sync with the actual situation.
0x02 Design Convention
To each his own
Things to note when creating a table
- The DDL statement for creating a table needs to use the standard format, with SQL keywords in uppercase letters and other words in lowercase letters.
- Use lowercase letters uniformly in field names/table names/aliases, and try not to be case-sensitive. If you encounter a mixed case, or a name that conflicts with SQL keywords, you need to use double quotation marks for quoting.
- Use specialized type (NUMERIC, ENUM, INET, MONEY, JSON, UUID, …) if applicable, and avoid using
TEXT
type as much as possible. TheTEXT
type is not conducive to the database’s understanding of the data. Use these types to improve data storage, query, indexing, and calculation efficiency, and improve maintainability. - Optimizing column layout and alignment types can have additional performance/storage gains.
- Unique constraints must be guaranteed by the database, and any unique column must have a corresponding unique constraint.
EXCLUDE
Constraints are generalized unique constraints that can be used to ensure data integrity in low-frequency update scenarios.
Partition table considerations
- If a single table exceeds hundreds of TB, or the monthly incremental data exceeds more than ten GB, you can consider table partitioning.
- A guideline for partitioning is to keep the size of each partition within the comfortable range of 1GB to 64GB.
- Tables that are conditionally partitioned by time range are first partitioned by time range. Commonly used granularities include: decade, year, month, day, and hour. The partitions required in the future should be created at least three months in advance.
- For extremely skewed data distributions, different time granularities can be combined, for example: 1900 - 2000 as one large partition, 2000 - 2020 as year partitions, and after 2020 as month partitions. When using time partitioning, the table name uses the value of the lower limit of the partition (if infinity, use a value that is far enough back).
Notes on wide tables
- Wide tables (for example, tables with dozens of fields) can be considered for vertical splitting, with mutual references to the main table through the same primary key.
- Because of the PostgreSQL MVCC mechanism, the write amplification phenomenon of wide tables is more obvious, reducing frequent updates to wide tables.
- In Internet scenarios, it is allowed to appropriately lower the normalization level and reduce multi-table connections to improve performance.
Primary key considerations
- Every table must have an identity column , and in principle it must have a primary key. The minimum requirement is to have a non-null unique constraint .
- The identity column is used to uniquely identify any tuple in the table, and logical replication and many third-party tools depend on it.
- If the primary key contains multiple columns, it should be specified using a single column after creating the field list of the table DDL
PRIMARY KEY(a,b,...)
. - In principle, it is recommended to use integer
UUID
types for primary keys, which can be used with caution and text types with limited length. Using other types requires explicit explanation and evaluation. - The primary key usually uses a single integer column. In principle, it is recommended to use it
BIGINT
. Use it with cautionINTEGER
and it is not allowedSMALLINT
. - The primary key should be used to
GENERATED ALWAYS AS IDENTITY
generate a unique primary key;SERIAL
,BIGSERIAL
which is only allowed when compatibility with PG versions below 10 is required. - The primary key can use
UUID
the type as the primary key, and it is recommended to use UUID v1/v7; use UUIDv4 as the primary key with caution, as random UUID has poor locality and has a collision probability. - When using a string column as a primary key, you should add a length limit. Generally used
VARCHAR(64)
, use of longer strings should be explained and evaluated. INSERT/UPDATE
In principle, it is forbidden to modify the value of the primary key column, andINSERT RETURNING
it can be used to return the automatically generated primary key value.
Foreign key considerations
- When defining a foreign key, the reference must explicitly set the corresponding action:
SET NULL
,SET DEFAULT
,CASCADE
, and use cascading operations with caution. - The columns referenced by foreign keys need to be primary key columns in other tables/this table.
- Internet businesses, especially partition tables and horizontal shard libraries, use foreign keys with caution and can be solved at the application layer.
Null/Default Value Considerations
- If there is no distinction between zero and null values in the field semantics, null values are not allowed and
NOT NULL
constraints must be configured for the column. - If a field has a default value semantically,
DEFAULT
the default value should be configured.
Numeric type considerations
- Used for regular numeric fields
INTEGER
. Used for numeric columns whose capacity is uncertainBIGINT
. - Don’t use it without special reasons
SMALLINT
. The performance and storage improvements are very small, but there will be many additional problems. - Note that the SQL standard does not provide unsigned integers, and values exceeding
INTMAX
but not exceedingUINTMAX
need to be upgraded and stored. Do not store moreINT64MAX
values inBIGINT
the column as it will overflow into negative numbers. REAL
Represents a 4-byte floating point number,FLOAT
represents an 8-byte floating point number. Floating point numbers can only be used in scenarios where the final precision doesn’t matter, such as geographic coordinates. Remember not to use equality judgment on floating point numbers, except for zero values .- Use exact numeric types
NUMERIC
. If possible, useNUMERIC(p)
andNUMERIC(p,s)
to set the number of significant digits and the number of significant digits in the decimal part. For example, the temperature in Celsius (37.0
) canNUMERIC(3,1)
be stored with 3 significant digits and 1 decimal place using type. - Currency value type is used
MONEY
.
Text type considerations
- PostgreSQL text types include
char(n)
,varchar(n)
,text
. By default,text
the type can be used, which does not limit the string length, but is limited by the maximum field length of 1GB. - If conditions permit, it is preferable to use
varchar(n)
the type to set a maximum string length. This will introduce minimal additional checking overhead, but can avoid some dirty data and corner cases. - Avoid use
char(n)
, this type has unintuitive behavior (padding spaces and truncation) and has no storage or performance advantages in order to be compatible with the SQL standard.
Time type considerations
- There are only two ways to store time: with time zone
TIMESTAMPTZ
and without time zoneTIMESTAMP
. - It is recommended to use one with time zone
TIMESTAMPTZ
. If you useTIMESTAMP
storage, you must use 0 time zone standard time. - Please use it to generate 0 time zone time
now() AT TIME ZONE 'UTC'
. You cannot truncate the time zone directlynow()::TIMESTAMP
. - Uniformly use ISO-8601 format input and output time type:
2006-01-02 15:04:05
to avoid DMY and MDY problems. - Users in China can use
Asia/Hong_Kong
the +8 time zone uniformly because the Shanghai time zone abbreviationCST
is ambiguous.
Notes on enumeration types
- Fields that are more stable and have a small value space (within tens to hundreds) should use enumeration types instead of integers and strings.
- Enumerations are internally implemented using dynamic integers, which have readability advantages over integers and performance, storage, and maintainability advantages over strings.
- Enumeration items can only be added, not deleted, but existing enumeration values can be renamed.
ALTER TYPE <enum_name>
Used to modify enumerations.
UUID type considerations
- Please note that the fully random UUIDv4 has poor locality when used as a primary key. Consider using UUIDv1/v7 instead if possible.
- Some UUID generation/processing functions require additional extension plug-ins, such as
uuid-ossp
,pg_uuidv7
etc. If you have this requirement, please specify it during configuration.
JSON type considerations
- Unless there is a special reason, always use the binary storage
JSONB
type and related functions instead of the text versionJSON
. - Note the subtle differences between atomic types in JSON and their PostgreSQL counterparts: the zero character
text
is not allowed in the type corresponding to a JSON string\u0000
, and the andnumeric
is not allowed in the type corresponding to a JSON numeric type . Boolean values only accept lowercase and literal values.NaN``infinity``true``false
- Please note that objects in the JSON standard
null
and null values in the SQL standardNULL
are not the same concept.
Array type considerations
- When storing a small number of elements, array fields can be used instead of individually.
- Suitable for storing data with a relatively small number of elements and infrequent changes. If the number of elements in the array is very large or changes frequently, consider using a separate table to store the data and using foreign key associations.
- For high-dimensional floating-point arrays, consider using
pgvector
the dedicated data types provided by the extension.
GIS type considerations
- The GIS type uses the srid=4326 reference coordinate system by default.
- Longitude and latitude coordinate points should use the Geography type without explicitly specifying the reference system coordinates 4326
Trigger considerations
- Triggers will increase the complexity and maintenance cost of the database system, and their use is discouraged in principle. The use of rule systems is prohibited and such requirements should be replaced by triggers.
- Typical scenarios for triggers are to automatically modify a row to the current timestamp after modifying it
updated_time
, or to record additions, deletions, and modifications of a table to another log table, or to maintain business consistency between the two tables. - Operations in triggers are transactional, meaning if the trigger or operations in the trigger fail, the entire transaction is rolled back, so test and prove the correctness of your triggers thoroughly. Special attention needs to be paid to recursive calls, deadlocks in complex query execution, and the execution sequence of multiple triggers.
Stored procedure/function considerations
-
Functions/stored procedures are suitable for encapsulating transactions, reducing concurrency conflicts, reducing network round-trips, reducing the amount of returned data, and executing a small amount of custom logic.
-
Stored procedures are not suitable for complex calculations, and are not suitable for trivial/frequent type conversion and packaging. In critical high-load systems, unnecessary computationally intensive logic in the database should be removed, such as using SQL in the database to convert WGS84 to other coordinate systems. Calculation logic closely related to data acquisition and filtering can use functions/stored procedures: for example, geometric relationship judgment in PostGIS.
-
Replaced functions and stored procedures that are no longer in use should be taken offline in a timely manner to avoid conflicts with future functions.
-
Use a unified syntax format for function creation. The signature occupies a separate line (function name and parameters), the return value starts on a separate line, and the language is the first label. Be sure to mark the function volatility level:
IMMUTABLE
,STABLE
,VOLATILE
. Add attribute tags, such as:RETURNS NULL ON NULL INPUT
,PARALLEL SAFE
,ROWS 1
etc.CREATE OR REPLACE FUNCTION nspname.myfunc(arg1_ TEXT, arg2_ INTEGER) RETURNS VOID LANGUAGE SQL STABLE PARALLEL SAFE ROWS 1 RETURNS NULL ON NULL INPUT AS $function$ SELECT 1; $function$;
Use sensible Locale options
- Used by default
en_US.UTF8
and cannot be changed without special reasons. - The default
collate
rule must beC
, to avoid string indexing problems. - https://mp.weixin.qq.com/s/SEXcyRFmdXNI7rpPUB3Zew
Use reasonable character encoding and localization configuration
- Character encoding must be used
UTF8
, any other character encoding is strictly prohibited. - Must be used
C
asLC_COLLATE
the default collation, any special requirements must be explicitly specified in the DDL/query clause to implement. - Character set
LC_CTYPE
is used by defaulten_US.UTF8
, some extensions rely on character set information to work properly, such aspg_trgm
.
Notes on indexing
- All online queries must design corresponding indexes according to their access patterns, and full table scans are not allowed except for very small tables.
- Indexes have a price, and it is not allowed to create unused indexes. Indexes that are no longer used should be cleaned up in time.
- When building a joint index, columns with high differentiation and selectivity should be placed first, such as ID, timestamp, etc.
- GiST index can be used to solve the nearest neighbor query problem, and traditional B-tree index cannot provide good support for KNN problem.
- For data whose values are linearly related to the storage order of the heap table, if the usual query is a range query, it is recommended to use the BRIN index. The most typical scenario is to only append written time series data. BRIN index is more efficient than Btree.
- When retrieving against JSONB/array fields, you can use GIN indexes to speed up queries.
Clarify the order of null values in B-tree indexes
NULLS FIRST
If there is a sorting requirement on a nullable column, it needs to be explicitly specified in the query and indexNULLS LAST
.- Note that
DESC
the default rule for sorting isNULLS FIRST
that null values appear first in the sort, which is generally not desired behavior. - The sorting conditions of the index must match the query, such as:
CREATE INDEX ON tbl (id DESC NULLS LAST);
Disable indexing on large fields
- The size of the indexed field cannot exceed 2KB (1/3 of the page capacity). You need to be careful when creating indexes on text types. The text to be indexed should use
varchar(n)
types with length constraints. - When a text type is used as a primary key, a maximum length must be set. In principle, the length should not exceed 64 characters. In special cases, the evaluation needs to be explicitly stated.
- If there is a need for large field indexing, you can consider hashing the large field and establishing a function index. Or use another type of index (GIN).
Make the most of functional indexes
- Any redundant fields that can be inferred from other fields in the same row can be replaced using functional indexes.
- For statements that often use expressions as query conditions, you can use expression or function indexes to speed up queries.
- Typical scenario: Establish a hash function index on a large field, and establish a
reverse
function index for text columns that require left fuzzy query.
Take advantage of partial indexes
- For the part of the query where the query conditions are fixed, partial indexes can be used to reduce the index size and improve query efficiency.
- If a field to be indexed in a query has only a limited number of values, several corresponding partial indexes can also be established.
- If the columns in some indexes are frequently updated, please pay attention to the expansion of these indexes.
0x03 Query Convention
The limits of my language mean the limits of my world.
—Ludwig Wittgenstein
Use service access
- Access to the production database must be through domain name access services , and direct connection using IP addresses is strictly prohibited.
- VIP is used for services and access, LVS/HAProxy shields the role changes of cluster instance members, and master-slave switching does not require application restart.
Read and write separation
- Internet business scenario: Write requests must go through the main library and be accessed through the Primary service.
- In principle, read requests go from the slave library and are accessed through the Replica service.
- Exceptions: If you need “Read Your Write” consistency guarantees, and significant replication delays are detected, read requests can access the main library; or apply to the DBA to provide Standby services.
Separation of speed and slowness
- Queries within 1 millisecond in production are called fast queries, and queries that exceed 1 second in production are called slow queries.
- Slow queries must go to the offline slave database - Offline service/instance, and a timeout should be set during execution.
- In principle, the execution time of online general queries in production should be controlled within 1ms.
- If the execution time of an online general query in production exceeds 10ms, the technical solution needs to be modified and optimized before going online.
- Online queries should be configured with a Timeout of the order of 10ms or faster to avoid avalanches caused by accumulation.
- ETL data from the primary is prohibited, and the offline service should be used to retrieve data from a dedicated instance.
Use connection pool
- Production applications must access the database through a connection pool and the PostgreSQL database through a 1:1 deployed Pgbouncer proxy. Offline service, individual users are strictly prohibited from using the connection pool directly.
- Pgbouncer connection pool uses Transaction Pooling mode by default. Some session-level functions may not be available (such as Notify/Listen), so special attention is required. Pre-1.21 Pgbouncer does not support the use of Prepared Statements in this mode. In special scenarios, you can use Session Pooling or bypass the connection pool to directly access the database, which requires special DBA review and approval.
- When using a connection pool, it is prohibited to modify the connection status, including modifying connection parameters, modifying search paths, changing roles, and changing databases. The connection must be completely destroyed after modification as a last resort. Putting the changed connection back into the connection pool will lead to the spread of contamination. Use of pg_dump to dump data via Pgbouncer is strictly prohibited.
Configure active timeout for query statements
- Applications should configure active timeouts for all statements and proactively cancel requests after timeout to avoid avalanches. (Go context)
- Statements that are executed periodically must be configured with a timeout smaller than the execution period to avoid avalanches.
- HAProxy is configured with a default connection timeout of 24 hours for rolling expired long connections. Please do not run SQL that takes more than 1 day to execute on offline instances. This requirement will be specially adjusted by the DBA.
Pay attention to replication latency
- Applications must be aware of synchronization delays between masters and slaves and properly handle situations where replication delays exceed reasonable limits.
- Under normal circumstances, replication delays are on the order of 100µs/tens of KB, but in extreme cases, slave libraries may experience replication delays of minutes/hours. Applications should be aware of this phenomenon and have corresponding degradation plans - Select Read from the main library and try again later, or report an error directly.
Retry failed transactions
- Queries may be killed due to concurrency contention, administrator commands, etc. Applications need to be aware of this and retry if necessary.
- When the application reports a large number of errors in the database, it can trigger the circuit breaker to avoid an avalanche. But be careful to distinguish the type and nature of errors.
Disconnected and reconnected
- The database connection may be terminated for various reasons, and the application must have a disconnection reconnection mechanism.
- It can be used
SELECT 1
as a heartbeat packet query to detect the presence of messages on the connection and keep it alive periodically.
Online service application code prohibits execution of DDL
- It is strictly forbidden to execute DDL in production applications and do not make big news in the application code.
- Exception scenario: Creating new time partitions for partitioned tables can be carefully managed by the application.
- Special exception: Databases used by office systems, such as Gitlab/Jira/Confluence, etc., can grant application DDL permissions.
SELECT statement explicitly specifies column names
- Avoid using it
SELECT *
, orRETURNING
use it in a clause*
. Please use a specific field list and do not return unused fields. When the table structure changes (for example, a new value column), queries that use column wildcards are likely to encounter column mismatch errors. - After the fields of some tables are maintained, the order will change. For example: after
id
upgrading the INTEGER primary key toBIGINT
,id
the column order will be the last column. This problem can only be fixed during maintenance and migration. R&D developers should resist the compulsion to adjust the column order and explicitly specify the column order in the SELECT statement. - Exception: Wildcards are allowed when a stored procedure returns a specific table row type.
Disable online query full table scan
- Exceptions: constant minimal table, extremely low-frequency operations, table/return result set is very small (within 100 records/100 KB).
- Using negative operators such as on the first-level filter condition will result in a full table scan and must be
!=
avoided .<>
Disallow long waits in transactions
- Transactions must be committed or rolled back as soon as possible after being started. Transactions that exceed 10 minutes
IDEL IN Transaction
will be forcibly killed. - Applications should enable AutoCommit to avoid
BEGIN
unpairedROLLBACK
or unpaired applications laterCOMMIT
. - Try to use the transaction infrastructure provided by the standard library, and do not control transactions manually unless absolutely necessary.
Things to note when using count
count(*)
It is the standard syntax for counting rows and has nothing to do with null values.count(col)
The count is the number of non-null recordscol
in the column . NULL values in this column will not be counted.count(distinct col)
Whencol
deduplicating columns and counting them, null values are also ignored, that is, only the number of non-null distinct values is counted.count((col1, col2))
When counting multiple columns, even if the columns to be counted are all empty, they will still be counted.(NULL,NULL)
This is valid.a(distinct (col1, col2))
For multi-column deduplication counting, even if the columns to be counted are all empty, they will be counted,(NULL,NULL)
which is effective.
Things to note when using aggregate functions
- All
count
aggregate functions exceptNULL
Butcount(col)
in this case it will be returned0
as an exception. - If returning null from an aggregate function is not expected, use
coalesce
to set a default value.
Handle null values with caution
-
Clearly distinguish between zero values and null values. Use null values
IS NULL
for equivalence judgment, and use regular=
operators for zero values for equivalence judgment. -
When a null value is used as a function input parameter, it should have a type modifier, otherwise the overloaded function will not be able to identify which one to use.
-
Pay attention to the null value comparison logic: the result of any comparison operation involving null values is
unknown
you need to pay attention tonull
the logic involved in Boolean operations:and
:TRUE or NULL
Will return due to logical short circuitTRUE
.or
:FALSE and NULL
Will return due to logical short circuitFALSE
- In other cases, as long as the operand appears
NULL
, the result isNULL
-
The result of logical judgment between null value and any value is null value, for example,
NULL=NULL
the return result isNULL
notTRUE/FALSE
. -
For equality comparisons involving null values and non-null values, please use ``IS DISTINCT FROM
-
NULL values and aggregate functions: When all input values are NULL, the aggregate function returns NULL.
Note that the serial number is empty
- When using
Serial
types,INSERT
,UPSERT
and other operations will consume sequence numbers, and this consumption will not be rolled back when the transaction fails. - When using an integer
INTEGER
as the primary key and the table has frequent insertion conflicts, you need to pay attention to the problem of integer overflow.
The cursor must be closed promptly after use
Repeated queries using prepared statements
- Prepared Statements should be used for repeated queries to eliminate the CPU overhead of database hard parsing. Pgbouncer versions earlier than 1.21 cannot support this feature in transaction pooling mode, please pay special attention.
- Prepared statements will modify the connection status. Please pay attention to the impact of the connection pool on prepared statements.
Choose the appropriate transaction isolation level
- The default isolation level is read committed , which is suitable for most simple read and write transactions. For ordinary transactions, choose the lowest isolation level that meets the requirements.
- For write transactions that require transaction-level consistent snapshots, use the Repeatable Read isolation level.
- For write transactions that have strict requirements on correctness (such as money-related), use the serializable isolation level.
- When a concurrency conflict occurs between the RR and SR isolation levels, the application should actively retry depending on the error type.
rh 09 Do not use count when judging the existence of a result.
- It is faster than Count to
SELECT 1 FROM tbl WHERE xxx LIMIT 1
judge whether there are columns that meet the conditions. SELECT exists(SELECT * FROM tbl WHERE xxx LIMIT 1)
The existence result can be converted to a Boolean value using .
Use the RETURNING clause to retrieve the modified results in one go
RETURNING
The clause can be used after theINSERT
,UPDATE
,DELETE
statement to effectively reduce the number of database interactions.
Use UPSERT to simplify logic
- When the business has an insert-failure-update sequence of operations, consider using
UPSERT
substitution.
Use advisory locks to deal with hotspot concurrency .
- For extremely high-frequency concurrent writes (spike) of single-row records, advisory locks should be used to lock the record ID.
- If high concurrency contention can be resolved at the application level, don’t do it at the database level.
Optimize IN operator
- Use
EXISTS
clause instead ofIN
operator for better performance. - Use
=ANY(ARRAY[1,2,3,4])
insteadIN (1,2,3,4)
for better results. - Control the size of the parameter list. In principle, it should not exceed 10,000. If it exceeds, you can consider batch processing.
It is not recommended to use left fuzzy search
- Left fuzzy search
WHERE col LIKE '%xxx'
cannot make full use of B-tree index. If necessary,reverse
expression function index can be used.
Use arrays instead of temporary tables
- Consider using an array instead of a temporary table, for example when obtaining corresponding records for a series of IDs.
=ANY(ARRAY[1,2,3])
Better than temporary table JOIN.
0x04 Administration Convention
Use Pigsty to build PostgreSQL cluster and infrastructure
- The production environment uses the Pigsty trunk version uniformly, and deploys the database on x86_64 machines and CentOS 7.9 / RockyLinux 8.8 operating systems.
pigsty.yml
Configuration files usually contain highly sensitive and important confidential information. Git should be used for version management and access permissions should be strictly controlled.files/pki
The CA private key and other certificates generated within the system should be properly kept, regularly backed up to a secure area for storage and archiving, and access permissions should be strictly controlled.- All passwords are not allowed to use default values, and make sure they have been changed to new passwords with sufficient strength.
- Strictly control access rights to management nodes and configuration code warehouses, and only allow DBA login and access.
Monitoring system is a must
- Any deployment must have a monitoring system, and the production environment uses at least two sets of Infra nodes to provide redundancy.
Properly plan the cluster architecture according to needs
- Any production database cluster managed by a DBA must have at least one online slave database for online failover.
- The template is used by default
oltp
, the analytical database usesolap
the template, the financial database usescrit
the template, and the micro virtual machine (within four cores) usestiny
the template. - For businesses whose annual data volume exceeds 1TB, or for clusters whose write TPS exceeds 30,000 to 50,000, you can consider building a horizontal sharding cluster.
Configure cluster high availability using Patroni and Etcd
- The production database cluster uses Patroni as the high-availability component and etcd as the DCS.
etcd
Use a dedicated virtual machine cluster, with 3 to 5 nodes, strictly scattered and distributed on different cabinets.- Patroni Failsafe mode must be turned on to ensure that the cluster main library can continue to work when etcd fails.
Configure cluster PITR using pgBackRest and MinIO
- The production database cluster uses pgBackRest as the backup recovery/PITR solution and MinIO as the backup storage warehouse.
- MinIO uses a multi-node multi-disk cluster, and can also use S3/OSS/COS services instead. Password encryption must be set for cold backup.
- All database clusters perform a local full backup every day, retain the backup and WAL of the last week, and save a full backup every other month.
- When a WAL archiving error occurs, you should check the backup warehouse and troubleshoot the problem in time.
Core business database configuration considerations
- The core business cluster needs to configure at least two online slave libraries, one of which is a dedicated offline query instance.
- The core business cluster needs to build a delayed slave cluster with a 24-hour delay for emergency data recovery.
- Core business clusters usually use asynchronous submission, while those related to money use synchronous submission.
Financial database configuration considerations
- The financial database cluster requires at least two online slave databases, one of which is a dedicated synchronization Standby instance, and Standby service access is enabled.
- Money-related libraries must use
crit
templates with RPO = 0, enable synchronous submission to ensure zero data loss, and enable Watchdog as appropriate. - Money-related libraries must be forced to turn on data checksums and, if appropriate, turn on full DML logs.
Use reasonable character encoding and localization configuration
- Character encoding must be used
UTF8
, any other character encoding is strictly prohibited. - Must be used
C
asLC_COLLATE
the default collation, any special requirements must be explicitly specified in the DDL/query clause to implement. - Character set
LC_CTYPE
is used by defaulten_US.UTF8
, some extensions rely on character set information to work properly, such aspg_trgm
.
Business database management considerations
- Multiple different databases are allowed to be created in the same cluster, and Ansible scripts must be used to create new business databases.
- All business databases must exist synchronously in the Pgbouncer connection pool.
Business user management considerations
- Different businesses/services must use different database users, and Ansible scripts must be used to create new business users.
- All production business users must be synchronized in the user list file of the Pgbouncer connection pool.
- Individual users should set a password with a default validity period of 90 days and change it regularly.
- Individual users are only allowed to access authorized cluster offline instances or slave
pg_offline_query
libraries with from the springboard machine.
Notes on extension management
yum/apt
When installing a new extension, you must first install the corresponding major version of the extension binary package in all instances of the cluster .- Before enabling the extension, you need to confirm whether the extension needs to be added
shared_preload_libraries
. If necessary, a rolling restart should be arranged. - Note that
shared_preload_libraries
in order of priority,citus
,timescaledb
,pgml
are usually placed first. pg_stat_statements
andauto_explain
are required plugins and must be enabled in all clusters.- Install extensions uniformly using , and create them
dbsu
in the business database .CREATE EXTENSION
Database XID and age considerations
- Pay attention to the age of the database and tables to avoid running out of XID transaction numbers. If the usage exceeds 20%, you should pay attention; if it exceeds 50%, you should intervene immediately.
- When processing XID, execute the table one by one in order of age from largest to smallest
VACUUM FREEZE
.
Database table and index expansion considerations
- Pay attention to the expansion rate of tables and indexes to avoid index performance degradation, and use
pg_repack
online processing to handle table/index expansion problems. - Generally speaking, indexes and tables whose expansion rate exceeds 50% can be considered for reorganization.
- When dealing with table expansion exceeding 100GB, you should pay special attention and choose business low times.
Database restart considerations
- Before restarting the database, execute it
CHECKPOINT
twice to force dirty pages to be flushed, which can speed up the restart process. - Before restarting the database, perform
pg_ctl reload
reload configuration to confirm that the configuration file is available normally. - To restart the database, use
pg_ctl restart
patronictl or patronictl to restart the entire cluster at the same time. - Use
kill -9
to shut down any database process is strictly prohibited.
Replication latency considerations
- Monitor replication latency, especially when using replication slots.
New slave database data warm-up
- When adding a new slave database instance to a high-load business cluster, the new database instance should be warmed up, and the HAProxy instance weight should be gradually adjusted and applied in gradients: 4, 8, 16, 32, 64, and 100.
pg_prewarm
Hot data can be loaded into memory using .
Database publishing process
- Online database release requires several evaluation stages: R&D self-test, supervisor review, QA review (optional), and DBA review.
- During the R&D self-test phase, R&D should ensure that changes are executed correctly in the development and pre-release environments.
- If a new table is created, the record order magnitude, daily data increment estimate, and read and write throughput magnitude estimate should be given.
- If it is a new function, the average execution time and extreme case descriptions should be given.
- If it is a mode change, all upstream and downstream dependencies must be sorted out.
- If it is a data change and record revision, a rollback SQL must be given.
- The R&D Team Leader needs to evaluate and review changes and be responsible for the content of the changes.
- The DBA evaluates and reviews the form and impact of the release, puts forward review opinions, and calls back or implements them uniformly.
Data work order format
- Database changes are made through the platform, with one work order for each change.
- The title is clear: A certain business needs
xx
to perform an action in the databaseyy
. - The goal is clear: what operations need to be performed on which instances in each step, and how to verify the results.
- Rollback plan: Any changes need to provide a rollback plan, and new ones also need to provide a cleanup script.
- Any changes need to be recorded and archived, and have complete approval records. They are first approved by the R&D superior TL Review and then approved by the DBA.
Database change release considerations
- Using a unified release window, changes of the day will be collected uniformly at 16:00 every day and executed sequentially; requirements confirmed by TL after 16:00 will be postponed to the next day. Database release is not allowed after 19:00. For emergency releases, please ask TL to make special instructions and send a copy to the CTO for approval before execution.
- Database DDL changes and DML changes are uniformly
dbuser_dba
executed remotely using the administrator user to ensure that the default permissions work properly. - When the business administrator executes DDL by himself, he must
SET ROLE dbrole_admin
first execute the release to ensure the default permissions. - Any changes require a rollback plan before they can be executed, and very few operations that cannot be rolled back need to be handled with special caution (such as enumeration of value additions)
- Database changes use
psql
command line tools, connect to the cluster main database to execute, use\i
execution scripts or\e
manual execution in batches.
Things to note when deleting tables
- The production data table
DROP
should be renamed first and allowed to cool for 1 to 3 days to ensure that it is not accessed before being removed. - When cleaning the table, you must sort out all dependencies, including directly and indirectly dependent objects: triggers, foreign key references, etc.
- The temporary table to be deleted is usually placed in
trash
Schema andALTER TABLE SET SCHEMA
the schema name is modified. - In high-load business clusters, when removing particularly large tables (> 100G), select business valleys to avoid preempting I/O.
Things to note when creating and deleting indexes
- You must use
CREATE INDEX CONCURRENTLY
concurrent index creation andDROP INDEX CONCURRENTLY
concurrent index removal. - When rebuilding an index, always create a new index first, then remove the old index, and modify the new index name to be consistent with the old index.
- After index creation fails, you should remove
INVALID
the index in time. After modifying the index, useanalyze
to re-collect statistical data on the table. - When the business is idle, you can enable parallel index creation and set it
maintenance_work_mem
to a larger value to speed up index creation.
Make schema changes carefully
- Try to avoid full table rewrite changes as much as possible. Full table rewrite is allowed for tables within 1GB. The DBA should notify all relevant business parties when the changes are made.
- When adding new columns to an existing table, you should avoid using functions in default values
VOLATILE
to avoid a full table rewrite. - When changing a column type, all functions and views that depend on that type should be rebuilt if necessary, and
ANALYZE
statistics should be refreshed.
Control the batch size of data writing
- Large batch write operations should be divided into small batches to avoid generating a large amount of WAL or occupying I/O at one time.
- After a large batch
UPDATE
is executed,VACUUM
the space occupied by dead tuples is reclaimed. - The essence of executing DDL statements is to modify the system directory, and it is also necessary to control the number of DDL statements in a batch.
Data loading considerations
- Use
COPY
load data, which can be executed in parallel if necessary. - You can temporarily shut down before loading data
autovacuum
, disable triggers as needed, and create constraints and indexes after loading. - Turn it up
maintenance_work_mem
, increase itmax_wal_size
. - Executed after loading is complete
vacuum verbose analyze table
.
Notes on database migration and major version upgrades
- The production environment uniformly uses standard migration to build script logic, and realizes requirements such as non-stop cluster migration and major version upgrades through blue-green deployment.
- For clusters that do not require downtime, you can use
pg_dump | psql
logical export and import to stop and upgrade.
Data Accidental Deletion/Accidental Update Process
- After an accident occurs, immediately assess whether it is necessary to stop the operation to stop bleeding, assess the scale of the impact, and decide on treatment methods.
- If there is a way to recover on the R&D side, priority will be given to the R&D team to make corrections through SQL publishing; otherwise, use
pageinspect
andpg_dirtyread
to rescue data from the bad table. - If there is a delayed slave library, extract data from the delayed slave library for repair. First, confirm the time point of accidental deletion, and advance the delay to extract data from the database to the XID.
- A large area was accidentally deleted and written. After communicating with the business and agreeing, perform an in-place PITR rollback to a specific time.
Data corruption processing process
- Confirm whether the slave database data can be used for recovery. If the slave database data is intact, you can switchover to the slave database first.
- Temporarily shut down
auto_vacuum
, locate the root cause of the error, replace the failed disk and add a new slave database. - If the system directory is damaged, or use to
pg_filedump
recover data from table binaries. - If the CLOG is damaged, use
dd
to generate a fake submission record.
Things to note when the database connection is full
- When the connection is full (avalanche), immediately use the kill connection query to cure the symptoms and stop the loss:
pg_cancel_backend
orpg_terminate_backend
. - Use to
pg_terminate_backend
abort all normal backend processes,psql
\watch 1
starting with once per second ( ). And confirm the connection status from the monitoring system. If the accumulation continues, continue to increase the execution frequency of the connection killing query, for example, once every 0.1 seconds until there is no more accumulation. - After confirming that the bleeding has stopped from the monitoring system, try to stop the killing connection. If the accumulation reappears, immediately resume the killing connection. Immediately analyze the root cause and perform corresponding processing (upgrade, limit current, add index, etc.)
FerretDB: When PG Masquerades as MongoDB
MongoDB was once a revolutionary technology that liberated developers from the “schema shackles” of relational databases, enabling rapid application development. However, as time passed, MongoDB gradually drifted away from its open-source roots, leaving many open-source projects and early-stage businesses in a bind.
Here’s the thing: most MongoDB users don’t actually need MongoDB’s advanced features. What they really need is a user-friendly, open-source document database solution. PostgreSQL has already evolved into a fully-featured, high-performance document database with its mature JSON capabilities: binary JSONB storage, GIN indexing for arbitrary fields, rich JSON processing functions, JSON PATH, and JSON Schema support. But having alternative functionality is one thing - providing a direct emulation is another beast entirely.
Enter FerretDB, born to fill this gap with a mission to provide a truly open-source MongoDB alternative. It’s an fascinating project with an interesting history - it was originally named “MangoDB” but changed to FerretDB for its 1.0 release to avoid any confusion with “MongoDB” (Mango DB vs Mongo DB). FerretDB offers applications using MongoDB drivers a smooth migration path to PostgreSQL.
Its magic trick? Making PostgreSQL impersonate MongoDB. It acts as a protocol translation middleware/proxy that enables PG to speak the MongoDB Wire Protocol. The last time we saw something similar was AWS’s Babelfish, which made PostgreSQL pretend to be Microsoft SQL Server by supporting the SQL Server wire protocol.
As an optional component, FerretDB significantly enriches the PostgreSQL ecosystem. Pigsty has supported FerretDB deployment via Docker templates since version 1.x and now offers native deployment support in v2.3. The Pigsty community has formed a partnership with the FerretDB community, paving the way for deeper integration and support.
This article will walk you through the installation, deployment, and usage of FerretDB.
Configuration
Before deploying a Mongo (FerretDB) cluster, you’ll need to define it in your configuration manifest. Here’s an example that uses the default single-node pg-meta
cluster’s meta
database as FerretDB’s underlying storage:
ferret:
hosts: { 10.10.10.10: { mongo_seq: 1 } }
vars:
mongo_cluster: ferret
mongo_pgurl: 'postgres://dbuser_meta:DBUser.Meta@10.10.10.10:5432/meta'
Here mongo_cluster
and mongo_seq
are essential identity parameters, and for FerretDB, another required parameter is mongo_pgurl
, specifying the underlying PG location.
You can use the pg-meta
cluster as the underlying storage for FerretDB, and deploy multiple FerretDB instance replicas with L2 VIP binding to achieve high availability at the FerretDB layer itself.
ferret-ha:
hosts:
10.10.10.45: { mongo_seq: 1 }
10.10.10.46: { mongo_seq: 2 }
10.10.10.47: { mongo_seq: 3 }
vars:
mongo_cluster: ferret
mongo_pgurl: 'postgres://test:test@10.10.10.3:5436/test'
vip_enabled: true
vip_vrid: 128
vip_address: 10.10.10.99
vip_interface: eth1
Management
Create Mongo Cluster
After defining the MONGO cluster in the configuration manifest, you can use the following command to complete the installation.
./mongo.yml -l ferret # Install "MongoDB/FerretDB" on the ferret group
Because FerretDB uses PostgreSQL as its underlying storage, repeating this playbook usually won’t cause any harm.
Remove Mongo Cluster
To remove the Mongo/FerretDB cluster, run the mongo.yml
playbook’s task: mongo_purge
, and use the mongo_purge
command line parameter:
./mongo.yml -e mongo_purge=true -t mongo_purge
Install MongoSH
You can use MongoSH as a client tool to access the FerretDB cluster
cat > /etc/yum.repos.d/mongo.repo <<EOF
[mongodb-org-6.0]
name=MongoDB Repository
baseurl=https://repo.mongodb.org/yum/redhat/$releasever/mongodb-org/6.0/$basearch/
gpgcheck=1
enabled=1
gpgkey=https://www.mongodb.org/static/pgp/server-6.0.asc
EOF
yum install -y mongodb-mongosh
Of course, you can also directly install the RPM package of mongosh
:
rpm -ivh https://mirrors.tuna.tsinghua.edu.cn/mongodb/yum/el7/RPMS/mongodb-mongosh-1.9.1.x86_64.rpm
Connect to FerretDB
You can use the MongoDB connection string to access FerretDB using any language’s MongoDB driver. Here’s an example using the mongosh
command line tool we installed:
mongosh 'mongodb://dbuser_meta:DBUser.Meta@10.10.10.10:27017?authMechanism=PLAIN'mongosh 'mongodb://test:test@10.10.10.11:27017/test?authMechanism=PLAIN'
Pigsty’s managed PostgreSQL cluster uses scram-sha-256
as the default authentication method, so you must use the PLAIN
authentication method to connect to FerretDB. See FerretDB: Authentication[17] for more details.
You can also use other PostgreSQL users to access FerretDB by specifying them in the connection string:
mongosh 'mongodb://dbuser_dba:DBUser.DBA@10.10.10.10:27017?authMechanism=PLAIN'
Quick Start
You can connect to FerretDB and pretend it’s a MongoDB cluster.
$ mongosh 'mongodb://dbuser_meta:DBUser.Meta@10.10.10.10:27017?authMechanism=PLAIN'
MongoDB commands will be translated to SQL
commands and executed in the underlying PostgreSQL:
use test # CREATE SCHEMA test;
db.dropDatabase() # DROP SCHEMA test;
db.createCollection('posts') # CREATE TABLE posts(_data JSONB,...)
db.posts.insert({ # INSERT INTO posts VALUES(...);
title: 'Post One',body: 'Body of post one',category: 'News',tags: ['news', 'events'],
user: {name: 'John Doe',status: 'author'},date: Date()}
)
db.posts.find().limit(2).pretty() # SELECT * FROM posts LIMIT 2;
db.posts.createIndex({ title: 1 }) # CREATE INDEX ON posts(_data->>'title');
If you’re not familiar with MongoDB, here’s a quick start tutorial that’s also applicable to FerretDB: Perform CRUD Operations with MongoDB Shell[18]
If you want to generate some sample load, you can use mongosh
to execute the following simple test playbook:
cat > benchmark.js <<'EOF'
const coll = "testColl";
const numDocs = 10000;
for (let i = 0; i < numDocs; i++) { // insert
db.getCollection(coll).insert({ num: i, name: "MongoDB Benchmark Test" });
}
for (let i = 0; i < numDocs; i++) { // select
db.getCollection(coll).find({ num: i });
}
for (let i = 0; i < numDocs; i++) { // update
db.getCollection(coll).update({ num: i }, { $set: { name: "Updated" } });
}
for (let i = 0; i < numDocs; i++) { // delete
db.getCollection(coll).deleteOne({ num: i });
}
EOF
mongosh 'mongodb://dbuser_meta:DBUser.Meta@10.10.10.10:27017?authMechanism=PLAIN' benchmark.js
You can check out the MongoDB commands supported by FerretDB, as well as some known differences for basic usage, which usually isn’t a big deal.
- FerretDB uses the same protocol error names and codes, but the exact error messages may be different in some cases.
- FerretDB does not support NUL (
\0
) characters in strings. - FerretDB does not support nested arrays.
- FerretDB converts
-0
(negative zero) to0
(positive zero). - Document restrictions:
- document keys must not contain
.
sign; - document keys must not start with
$
sign; - document fields of double type must not contain
Infinity
,-Infinity
, orNaN
values.
- document keys must not contain
- When insert command is called, insert documents must not have duplicate keys.
- Update command restrictions:
- update operations producing
Infinity
,-Infinity
, orNaN
are not supported.
- update operations producing
- Database and collection names restrictions:
- name cannot start with the reserved prefix
_ferretdb_
; - database name must not include non-latin letters;
- collection name must be valid UTF-8 characters;
- name cannot start with the reserved prefix
- FerretDB offers the same validation rules for the
scale
parameter in both thecollStats
anddbStats
commands. If an invalidscale
value is provided in thedbStats
command, the same error codes will be triggered as with thecollStats
command.
Playbook
Pigsty provides a built-in playbook: mongo.yml
, for installing a FerretDB cluster on a node.
mongo.yml
This playbook consists of the following tasks:
mongo_check
: Check mongo identity parameters•mongo_dbsu
: Create the mongod operating system user•mongo_install
: Install mongo/ferretdb RPM package•mongo_purge
: Clean up existing mongo/ferretdb cluster (default not executed)•mongo_config
: Configure mongo/ferretdbmongo_cert
: Issue mongo/ferretdb SSL certificatemongo_launch
: Start mongo/ferretdb service•mongo_register
:Register mongo/ferretdb to Prometheus monitoring
Monitoring
MONGO module provides a simple monitoring panel: Mongo Overview
Mongo Overview
Mongo Overview: Mongo/FerretDB cluster overview
This monitoring panel provides basic monitoring metrics about FerretDB, as FerretDB uses PostgreSQL as its underlying storage, so more monitoring metrics, please refer to PostgreSQL itself monitoring.
Parameters
MONGO
[24] module provides 9 related configuration parameters, as shown in the table below:
Parameter | Type | Level | Comment |
---|---|---|---|
mongo_seq |
int | I | mongo instance number, required identity parameter |
mongo_cluster |
string | C | mongo cluster name, required identity parameter |
mongo_pgurl |
pgurl | C/I | mongo/ferretdb underlying PGURL connection string, required |
mongo_ssl_enabled |
bool | C | Whether mongo/ferretdb enable SSL? Default is false |
mongo_listen |
ip | C | mongo listening address, default to listen to all addresses |
mongo_port |
port | C | mongo service port, default to use 27017 |
mongo_ssl_port |
port | C | mongo TLS listening port, default to use 27018 |
mongo_exporter_port |
port | C | mongo exporter port, default to use 9216 |
mongo_extra_vars |
string | C | MONGO server additional environment variables, default blank string |
PostgreSQL, The most successful database
The StackOverflow 2023 Survey, featuring feedback from 90K developers across 185 countries, is out. PostgreSQL topped all three survey categories (used, loved, and wanted), earning its title as the undisputed “Decathlete Database” – it’s hailed as the “Linux of Database”!
What makes a database “successful”? It’s a mix of features, quality, security, performance, and cost, but success is mainly about adoption and legacy. The size, preference, and needs of its user base are what truly shape its ecosystem’s prosperity. StackOverflow’s annual surveys for seven years have provided a window into tech trends.
PostgreSQL is now the world’s most popular database.
PostgreSQL is developers’ favorite database!
PostgreSQL sees the highest demand among users!
Popularity, the used
reflects the past, the loved
indicates the present, and the wanted
suggests the future. These metrics vividly showcase the vitality of a technology. PostgreSQL stands strong in both stock and potential, unlikely to be rivaled soon.
As a dedicated user, community member, expert, evangelist, and contributor to PostgreSQL, witnessing this moment is profoundly moving. Let’s delve into the “Why” and “What” behind this phenomenon.
Source: Community Survey
Developers define the success of databases, and StackOverflow’s survey, with popularity, love, and demand metrics, captures this directly.
“Which database environments have you done extensive development work in over the past year, and which do you want to work in over the next year? If you both worked with the database and want to continue to do so, please check both boxes in that row.”
Each database in the survey had two checkboxes: one for current use, marking the user as “Used,” and one for future interest, marking them as “Wanted.” Those who checked both were labeled as “Loved/Admired.”
The percentage of “Used” respondents represents popularity or usage rate, shown as a bar chart, while “Wanted” indicates demand or desire, marked with blue dots. “Loved/Admired” shows as red dots, indicating love or reputation. In 2023, PostgreSQL outstripped MySQL in popularity, becoming the world’s most popular database, and led by a wide margin in demand and reputation.
Reviewing seven years of data and plotting the top 10 databases on a scatter chart of popularity vs. net love percentage (2*love% - 100), we gain insights into the database field’s evolution and sense of scale.
X: Popularity, Y: Net Love Index (2 * loved - 100)
The 2023 snapshot shows PostgreSQL in the top right, popular and loved, while MySQL, popular yet less favored, sits in the bottom right. Redis, moderately popular but much loved, is in the top left, and Oracle, neither popular nor loved, is in the bottom left. In the middle lie SQLite, MongoDB, and SQL Server.
Trends indicate PostgreSQL’s growing popularity and love; MySQL’s love remains flat with falling popularity. Redis and SQLite are progressing, MongoDB is peaking and declining, and the commercial RDBMSs SQL Server and Oracle are on a downward trend.
The takeaway: PostgreSQL’s standing in the database realm, akin to Linux in server OS, seems unshakeable for the foreseeable future.
Historical Accumulation: Popularity
PostgreSQL — The world’s most popular database
Popularity is the percentage of total users who have used a technology in the past year. It reflects the accumulated usage over the past year and is a core metric of factual significance.
In 2023, PostgreSQL, branded as the “most advanced,” surpassed the “most popular” database MySQL with a usage rate of 45.6%, leading by 4.5% and reaching 1.1 times the usage rate of MySQL at 41.1%. Among professional developers (about three-quarters of the sample), PostgreSQL had already overtaken MySQL in 2022, with a 0.8 percentage point lead (46.5% vs 45.7%); this gap widened in 2023 to 49.1% vs 40.6%, or 1.2 times the usage rate among professional developers.
Over the past years, MySQL enjoyed the top spot in database popularity, proudly claiming the title of the “world’s most popular open-source relational database.” However, PostgreSQL has now claimed the crown. Compared to PostgreSQL and MySQL, other databases are not in the same league in terms of popularity.
The key trend to note is that among the top-ranked databases, only PostgreSQL has shown a consistent increase in popularity, demonstrating strong growth momentum, while all other databases have seen a decline in usage. As time progresses, the gap in popularity between PostgreSQL and other databases will likely widen, making it hard for any challenger to displace PostgreSQL in the near future.
Notably, the “domestic database” TiDB has entered the StackOverflow rankings for the first time, securing the 32nd spot with a 0.2% usage rate.
Popularity reflects the current scale and potential of a database, while love indicates its future growth potential.
Current Momentum: Love
PostgreSQL — The database developers love the most
Love or admiration is a measure of the percentage of users who are willing to continue using a technology, acting as an annual “retention rate” metric that reflects the user’s opinion and evaluation of the technology.
In 2023, PostgreSQL retained its title as the most loved database by developers. While Redis had been the favorite in previous years, PostgreSQL overtook Redis in 2022, becoming the top choice. PostgreSQL and Redis have maintained close reputation scores (around 70%), significantly outpacing other contenders.
In the 2022 PostgreSQL community survey, the majority of existing PostgreSQL users reported increased usage and deeper engagement, highlighting the stability of its core user base.
Redis, known for its simplicity and ease of use as a data structure cache server, is often paired with the relational database PostgreSQL, enjoying considerable popularity (20%, ranking sixth) among developers. Cross-analysis shows a strong connection between the two: 86% of Redis users are interested in using PostgreSQL, and 30% of PostgreSQL users want to use Redis. Other databases with positive reviews include SQLite, MongoDB, and SQL Server. MySQL and ElasticSearch receive mixed feedback, hovering around the 50% mark. The least favored databases include Access, IBM DB2, CouchDB, Couchbase, and Oracle.
Not all potential can be converted into kinetic energy. While user affection is significant, it doesn’t always translate into action, leading to the third metric of interest – demand.
Future Trends: Demand
PostgreSQL - The Most Wanted Database
The demand rate, or the level of desire, represents the percentage of users who will actually opt for a technology in the coming year. PostgreSQL stands out in demand/desire, significantly outpacing other databases with a 42.3% rate for the second consecutive year, showing relentless growth and widening the gap with its competitors.
In 2023, some databases saw notable demand increases, likely driven by the surge in large language model AI, spearheaded by OpenAI’s ChatGPT. This demand for intelligence has, in turn, fueled the need for robust data infrastructure. A decade ago, support for NoSQL features like JSONB/GIN laid the groundwork for PostgreSQL’s explosive growth during the internet boom. Today, the introduction of pgvector, the first vector extension built on a mature database, grants PostgreSQL a ticket into the AI era, setting the stage for growth in the next decade.
But Why?
PostgreSQL leads in demand, usage, and popularity, with the right mix of timing, location, and human support, making it arguably the most successful database with no visible challengers in the near future. The secret to its success lies in its slogan: “The World’s Most Advanced Open Source Relational Database.”
Relational databases are so prevalent and crucial that they might dwarf the combined significance of other types like key-value, document, search engine, time-series, graph, and vector databases. Typically, “database” implicitly refers to “relational database,” where no other category dares claim mainstream status. Last year’s “Why PostgreSQL Will Be the Most Successful Database?” delves into the competitive landscape of relational databases—a tripartite dominance. Excluding Microsoft’s relatively isolated SQL Server, the database scene, currently in a phase of consolidation, has three key players rooted in WireProtocol: Oracle, MySQL, and PostgreSQL, mirroring a “Three Kingdoms” saga in the relational database realm.
Oracle/MySQL are waning, while PostgreSQL is thriving. Oracle is an established commercial DB with deep tech history, rich features, and strong support, favored by well-funded, risk-averse enterprises, especially in finance. Yet, it’s pricey and infamous for litigious practices. MS SQL Server shares similar traits with Oracle. Commercial databases are facing a slow decline due to the open-source wave.
MySQL, popular yet beleaguered, lags in stringent transaction processing and data analysis compared to PostgreSQL. Its agile development approach is also outperformed by NoSQL alternatives. Oracle’s dominance, sibling rivalry with MariaDB, and competition from NewSQL players like TiDB/OB contribute to its decline.
Oracle, no doubt skilled, lacks integrity, hence “talented but unprincipled.” MySQL, despite its open-source merit, is limited in capability and sophistication, hence “limited talent, weak ethics.” PostgreSQL, embodying both capability and integrity, aligns with the open-source rise, popular demand, and advanced stability, epitomizing “talented and principled.”
Open Source & Advanced
The primary reasons for choosing PostgreSQL, as reflected in the TimescaleDB community survey, are its open-source nature and stability. Open-source implies free use, potential for modification, no vendor lock-in, and no “chokepoint” issues. Stability means reliable, consistent performance with a proven track record in large-scale production environments. Experienced developers value these attributes highly.
Broadly, aspects like extensibility, ecosystem, community, and protocols fall under “open-source.” Stability, ACID compliance, SQL support, scalability, and availability define “advanced.” These resonate with PostgreSQL’s slogan: “The world’s most advanced open source relational database.”
The Virtue of Open Source
powered by developers worldwide. Friendly BSD license, thriving ecosystem, extensive expansion. A robust Oracle alternative, leading the charge.
What is “virtue”? It’s the manifestation of “the way,” and this way is open source. PostgreSQL stands as a venerable giant among open-source projects, epitomizing global collaborative success.
Back in the day, developing software/information services required exorbitantly priced commercial databases. Just the software licensing fees could hit six or seven figures, not to mention similar costs for hardware and service subscriptions. Oracle’s licensing fee per CPU core could reach hundreds of thousands annually, prompting even giants like Alibaba to seek IOE alternatives. The rise of open-source databases like PostgreSQL and MySQL offered a fresh choice.
Open-source databases, free of charge, spurred an industry revolution: from tens of thousands per core per month for commercial licenses to a mere 20 bucks per core per month for hardware. Databases became accessible to regular businesses, enabling the provision of free information services.
Open source has been monumental: the history of the internet is a history of open-source software. The prosperity of the IT industry and the plethora of free information services owe much to open-source initiatives. Open source represents a form of successful Communism in software, with the industry’s core means of production becoming communal property, available to developers worldwide as needed. Developers contribute according to their abilities, embracing the ethos of mutual benefit.
An open-source programmer’s work encapsulates the intellect of countless top-tier developers. Programmers command high salaries because they are not mere laborers but contractors orchestrating software and hardware. They own the core means of production: software from the public domain and readily available server hardware. Thus, a few skilled engineers can swiftly tackle domain-specific problems leveraging the open-source ecosystem.
Open source synergizes community efforts, drastically reducing redundancy and propelling technical advancements at an astonishing pace. Its momentum, now unstoppable, continues to grow like a snowball. Open source dominates foundational software, and the industry now views insular development or so-called “self-reliance” in software, especially in foundational aspects, as a colossal joke.
For PostgreSQL, open source is its strongest asset against Oracle.
Oracle is advanced, but PostgreSQL holds its own. It’s the most Oracle-compatible open-source database, natively supporting 85% of Oracle’s features, with specialized distributions reaching 96% compatibility. However, the real game-changer is cost: PG’s open-source nature and significant cost advantage provide a substantial ecological niche. It doesn’t need to surpass Oracle in features; being “90% right at a fraction of the cost” is enough to outcompete Oracle.
PostgreSQL is like an open-source “Oracle,” the only real threat to Oracle’s dominance. As a leader in the “de-Oracle” movement, PG has spawned numerous “domestically controllable” database companies. According to CITIC, 36% of “domestic databases” are based on PG modifications or rebranding, with Huawei’s openGauss and GaussDB as prime examples. Crucially, PostgreSQL uses a BSD-Like license, permitting such adaptations — you can rebrand and sell without deceit. This open attitude is something Oracle-acquired, GPL-licensed MySQL can’t match.
The advanced in Talent
The talent of PG lies in its advancement. Specializing in multiple areas, PostgreSQL offers a full-stack, multi-model approach: “Self-managed, autonomous driving temporal-geospatial AI vector distributed document graph with full-text search, programmable hyper-converged, federated stream-batch processing in a single HTAP Serverless full-stack platform database”, covering almost all database needs with a single component.
PostgreSQL is not just a traditional OLTP “relational database” but a multi-modal database. For SMEs, a single PostgreSQL component can cover the vast majority of their data needs: OLTP, OLAP, time-series, GIS, tokenization and full-text search, JSON/XML documents, NoSQL features, graphs, vectors, and more.
Emperor of Databases — Self-managed, autonomous driving temporal-geospatial AI vector distributed document graph with full-text search, programmable hyper-converged, federated stream-batch processing in a single HTAP Serverless full-stack platform database.
The superiority of PostgreSQL is not only in its acclaimed kernel stability but also in its powerful extensibility. The plugin system transforms PostgreSQL from a single-threaded evolving database kernel to a platform with countless parallel-evolving extensions, exploring all possibilities simultaneously like quantum computing. PostgreSQL is omnipresent in every niche of data processing.
For instance, PostGIS for geospatial databases, TimescaleDB for time-series, Citus for distributed/columnar/HTAP databases, PGVector for AI vector databases, AGE for graph databases, PipelineDB for stream processing, and the ultimate trick — using Foreign Data Wrappers (FDW) for unified SQL access to all heterogeneous external databases. Thus, PG is a true full-stack database platform, far more advanced than a simple OLTP system like MySQL.
Within a significant scale, PostgreSQL can play multiple roles with a single component, greatly reducing project complexity and cost. Remember, designing for unneeded scale is futile and an example of premature optimization. If one technology can meet all needs, it’s the best choice rather than reimplementing it with multiple components.
Taking Tantan as an example, with 250 million TPS and 200 TB of unique TP data, a single PostgreSQL selection remains stable and reliable, covering a wide range of functions beyond its primary OLTP role, including caching, OLAP, batch processing, and even message queuing. However, as the user base approaches tens of millions daily active users, these additional functions will eventually need to be handled by dedicated components.
PostgreSQL’s advancement is also evident in its thriving ecosystem. Centered around the database kernel, there are specialized variants and “higher-level databases” built on it, like Greenplum, Supabase (an open-source alternative to Firebase), and the specialized graph database edgedb, among others. There are various open-source/commercial/cloud distributions integrating tools, like different RDS versions and the plug-and-play Pigsty; horizontally, there are even powerful mimetic components/versions emulating other databases without changing client drivers, like babelfish for SQL Server, FerretDB for MongoDB, and EnterpriseDB/IvorySQL for Oracle compatibility.
PostgreSQL’s advanced features are its core competitive strength against MySQL, another open-source relational database.
Advancement is PostgreSQL’s core competitive edge over MySQL.
MySQL’s slogan is “the world’s most popular open-source relational database,” characterized by being rough, fierce, and fast, catering to internet companies. These companies prioritize simplicity (mainly CRUD), data consistency and accuracy less than traditional sectors like banking, and can tolerate data inaccuracies over service downtime, unlike industries that cannot afford financial discrepancies.
However, times change, and PostgreSQL has rapidly advanced, surpassing MySQL in speed and robustness, leaving only “roughness” as MySQL’s remaining trait.
MySQL allows partial transaction commits by default, shocked
MySQL allows partial transaction commits by default, revealing a gap between “popular” and “advanced.” Popularity fades with obsolescence, while advancement gains popularity through innovation. In times of change, without advanced features, popularity is fleeting. Research shows MySQL’s pride in “popularity” cannot stand against PostgreSQL’s “advanced” superiority.
Advancement and open-source are PostgreSQL’s success secrets. While Oracle is advanced and MySQL is open-source, PostgreSQL boasts both. With the right conditions, success is inevitable.
Looking Ahead
The PostgreSQL database kernel’s role in the database ecosystem mirrors the Linux kernel’s in the operating system domain. For databases, particularly OLTP, the battle of kernels has settled—PostgreSQL is now a perfect engine.
However, users need more than an engine; they need the complete car, driving capabilities, and traffic services. The database competition has shifted from software to Software enabled Service—complete database distributions and services. The race for PostgreSQL-based distributions is just beginning. Who will be the PostgreSQL equivalent of Debian, RedHat, or Ubuntu?
This is why we created Pigsty — to develop an battery-included, open-source, local-first PostgreSQL distribution, making it easy for everyone to access and utilize a quality database service. Due to space limits, the detailed story is for another time.
参考阅读
2022-08 《PostgreSQL 到底有多强?》
2022-07 《为什么PostgreSQL是最成功的数据库?》
2022-06 《StackOverflow 2022数据库年度调查》
2021-05 《Why PostgreSQL Rocks!》
2021-05 《为什么说PostgreSQL前途无量?》
2018 《PostgreSQL 好处都有啥?》
2023 《更好的开源RDS替代:Pigsty》
2023 《StackOverflow 7年调研数据跟踪》
2022 《PostgreSQL 社区状态调查报告 2022》
How Powerful Is PostgreSQL?
Last time, we analyzed StackOverflow survey data to explain why PostgreSQL is the most successful database.
This time, let’s rely on performance metrics to see just how powerful “the most successful” PostgreSQL really is. We want everyone to walk away feeling, in the words of a certain meme, “I know the numbers.”
TL;DR
If you’re curious about any of the following questions, this post should be helpful:
- How fast is PostgreSQL, exactly?
Point-read queries (QPS) can exceed 600k, and in extreme conditions can even hit 2 million. For mixed read-write TPS (4 writes + 1 read in each transaction), you can reach 70k+ or even as high as 140k. - How does PostgreSQL compare with MySQL at the performance limit?
Under extreme tuning, PostgreSQL’s point-read throughput beats MySQL by a noticeable margin. In other metrics, they’re roughly on par. - How does PostgreSQL compare with other databases?
Under the same hardware specs, “distributed/NewSQL” databases often lag far behind classic databases in performance. - What about PostgreSQL versus other analytical databases in TPC-H?
As a native hybrid transaction/analysis (HTAP) database, PostgreSQL’s analytical performance is quite impressive. - Are cloud databases or cloud servers actually cost-effective?
It turns out you could purchase a c5d.metal server outright (and host it yourself for 5 years) for about the cost of renting it on the cloud for 1 year. Meanwhile, a similarly provisioned cloud database of the same spec could cost about 20 times as much as a raw EC2 box in 1 year.
All detailed test steps and raw data are on: github.com/Vonng/pgtpc
PGBENCH
Technology evolves at breakneck speed. Although benchmark articles are everywhere, it’s tough to find reliable performance data that reflect today’s newest hardware and software. Here, we used pgbench to test the latest PostgreSQL 14.5 on two types of cutting-edge hardware specs:
We ran four different hardware setups: two Apple laptops and three AWS EC2 instances, specifically:
- A 2018 15-inch top-spec MacBook Pro using an Intel 6-core i9
- A 2021 16-inch MacBook Pro powered by an M1 Max chip (10-core)
- AWS z1d.2xlarge (8C / 64G)
- AWS c5d.metal (96C / 192G)
All are readily available commercial hardware configurations.
pgbench
is a built-in benchmarking tool for PostgreSQL, based on a TPC-B–like workload, widely used to evaluate PostgreSQL (and its derivatives/compatibles). We focused on two test modes:
- Read-Only (RO)
A single SQL statement that randomly selects and returns one row from a table of 100 million rows. - Read-Write (RW)
Five SQL statements per transaction: 1 read, 1 insert, and 3 updates.
We used s=1000
for the dataset scale, then gradually increased client connections. At peak throughput, we tested for 3-5 minutes and recorded stable averages. Results are as follows:
No | Spec | Config | CPU | Freq | S | RO | RW |
---|---|---|---|---|---|---|---|
1 | Apple MBP Intel 2018 | Normal | 6 | 2.9GHz - 4.8GHz | 1000 | 113,870 | 15,141 |
2 | AWS z1d.2xlarge | Normal | 8 | 4GHz | 1000 | 162,315 | 24,808 |
3 | Apple MBP M1 Max 2021 | Normal | 10 | 600MHz - 3.22GHz | 1000 | 240,841 | 31,903 |
4 | AWS c5d.metal | Normal | 96 | 3.6GHz | 1000 | 625,849 | 71,624 |
5 | AWS c5d.metal | Extreme | 96 | 3.6GHz | 5000 | 1,998,580 | 137,127 |
Read-Write
Chart: Max read-write TPS on each hardware
Chart: TPS curves for read-write transactions
Read-Only
Chart: Max point-read QPS on each hardware
Chart: Point-read QPS vs. concurrency
These results are jaw-dropping. On a 10-core Apple M1 Max laptop, PostgreSQL hits ~32k TPS (read-write) and ~240k QPS (point lookups). On an AWS c5d.metal production-grade physical server, it goes up to ~72k TPS and ~630k QPS. With extreme config tuning, we were able to push it to 137k TPS and 2 million QPS on a single machine. Yes, a single server can do that.
By way of rough scale reference: Tantan (a major dating/social networking app in China) has a global TPS of ~400k across its entire PostgreSQL fleet. These new laptops or a few top-spec servers (costing around 100k RMB) could potentially support a large-scale app’s DB load. That’s insane compared to just a few years ago.
A Note on Costs
Take AWS c5d.metal in Ningxia region as an example. It’s one of the best overall compute options, coming with local 3.6TB NVMe SSD storage. There are seven different payment models (prices below in RMB/month or RMB/year):
Payment Model | Monthly | Up-Front | Yearly |
---|---|---|---|
On-Demand | 31,927 | 0 | 383,124 |
Standard 1-yr, no upfront | 12,607 | 0 | 151,284 |
Standard 1-yr, partial | 5,401 | 64,540 | 129,352 |
Standard 1-yr, all upfront | 0 | 126,497 | 126,497 |
Convertible 3-yr, no upfront | 11,349 | 0 | 136,188 |
Convertible 3-yr, partial | 4,863 | 174,257 | 116,442 |
Convertible 3-yr, all upfront | 0 | 341,543 | 113,847 |
Effectively, annual costs range from about 110k to 150k RMB, or 380k at on-demand retail. Meanwhile, buying a similar server outright and hosting it in a data center for 5 years might cost under 100k total. So yes, the cloud is easily ~5x more expensive if you only compare raw hardware costs. Still, if you consider the elasticity, discount programs, and coupon offsets, an EC2 instance can be “worth it,” especially if you self-manage PostgreSQL on it.
But if you want an RDS for PostgreSQL with roughly the same specs (the closest is db.m5.24xlarge, 96C/384G + 3.6T io1 @80k IOPS), the monthly cost is ~240k RMB, or 2.87 million RMB per year, nearly 20 times more than simply running PostgreSQL on the same c5d.metal instance yourself.
AWS cost calculator: https://calculator.amazonaws.cn/
SYSBENCH
So PostgreSQL alone is impressive—but how does it compare to other databases? pgbench
is built for PostgreSQL-based systems. For a broader look, we can turn to sysbench, an open-source, multi-threaded benchmarking tool that can assess transaction performance in any SQL database (commonly used for both MySQL and PostgreSQL). It includes 10 typical scenarios like:
oltp_point_select
for point-read performanceoltp_update_index
for index update performanceoltp_read_only
for transaction mixes of 16 queriesoltp_read_write
for a mix of 20 queries in a transaction (read + write)oltp_write_only
for a set of 6 insert/update statements
Because sysbench can test both MySQL and PostgreSQL, it provides a fair basis for comparing their performance. Let’s start with the most popular face-off: the world’s “most popular” open-source RDBMS—MySQL—vs. the world’s “most advanced” open-source RDBMS—PostgreSQL.
Dirty Hack
MySQL doesn’t provide official sysbench results, but there is a third-party benchmark on MySQL.com claiming 1 million QPS for point-reads, 240k for index updates, and about 39k TPS for mixed read-write.
That approach is somewhat “unethical” if you will—because reading the linked article reveals that they turned off all major safety features to get these numbers: no binlog, no fsync on commit, no double-write buffer, no checksums, forcing LATIN-1, no monitoring, etc. Great for scoreboard inflation, not so great for real production usage.
But if we’re going down that path, we can similarly do a “Dirty Hack” for PostgreSQL—shut off everything that ensures data safety—and see how high we can push the scoreboard. The result? PostgreSQL point-reads soared past 2.33 million QPS, beating MySQL’s 1M QPS by more than double.
Chart: “Unfair” Benchmark—PG vs. MySQL, everything turned off
PostgreSQL’s “extreme config” point-read test in progress
To be fair, MySQL’s test used a 48C/2.7GHz machine, whereas our PostgreSQL run was on a 96C/3.6GHz box. But because PostgreSQL uses a multi-process model (rather than MySQL’s multi-thread model), we can sample performance at c=48
to approximate performance if we only had 48 cores. That still gives ~1.5M QPS for PG on 48 cores, 43% higher than MySQL’s best number.
We’d love to see MySQL experts produce a benchmark on identical hardware for a more direct comparison.
Chart: Four sysbench metrics from MySQL’s “Dirty Hack,” with c=48 concurrency
In other tests, MySQL also reaches impressive extremes. oltp_read_only
and oltp_update_non_index
are roughly on par with PG’s c=48 scenario, and MySQL even beats PostgreSQL by a bit in oltp_read_write
. Overall, aside from a resounding win for PostgreSQL in point-reads, the two are basically neck and neck in these “unfair” scenarios.
Fair Play
In terms of features, MySQL and PostgreSQL are worlds apart. But at the performance limit, the two are close, with PostgreSQL taking the lead in point-lookups. Now, how about next-generation, distributed, or “NewSQL” databases?
Most “Fair Play” database vendors who show sysbench benchmarks do so in realistic, production-like configurations (unlike MySQL’s “dirty hack”). So let’s compare them with a fully production-configured PostgreSQL on the same c5d.metal machine. Production config obviously reduces PG’s peak throughput by about half, but it’s more appropriate for apples-to-apples comparisons.
We collected official sysbench numbers from a few representative NewSQL database websites (or at least from detailed 3rd-party tests). Not every system published results for all 10 sysbench scenarios, and the hardware/table sizes vary. However, each test environment is around 100 cores with ~160M rows (except OB, YB, or where stated). That should give us enough to see who’s who:
Database | PGSQL.C5D96C | TiDB.108C | OceanBase.96C | PolarX.64C | Cockroach | Yugabyte |
---|---|---|---|---|---|---|
oltp_point_select | 1,372,654 | 407,625 | 401,404 | 336,000 | 95,695 | |
oltp_read_only | 852,440 | 279,067 | 366,863 | 52,416 | ||
oltp_read_write | 519,069 | 124,460 | 157,859 | 177,506 | 9,740 | |
oltp_write_only | 495,942 | 119,307 | 9,090 | |||
oltp_delete | 839,153 | 67,499 | ||||
oltp_insert | 164,351 | 112,000 | 6,348 | |||
oltp_update_non_index | 217,626 | 62,084 | 11,496 | |||
oltp_update_index | 169,714 | 26,431 | 4,052 | |||
select_random_points | 227,623 | |||||
select_random_ranges | 24,632 | |||||
Machine | c5d.metal | m5.xlarge x3 i3.4xlarge x3 c5.4xlarge x3 |
ecs.hfg7.8xlarge x3 ecs.hfg7.8xlarge x1 |
Enterprise | c5d.9xlarge x3 | c5.4xlarge x3 |
Spec | 96C / 192G | 108C / 510G | 96C / 384G | 64C / 256G | 108C / 216G | 48C / 96G |
Table | 16 x 10M | 16 x 10M | 30 x 10M | 1 x 160M | N/A | 10 x 0.1M |
CPU | 96 | 108 | 96 | 64 | 108 | 48 |
Source | Vonng | TiDB 6.1 | OceanBase | PolarDB | Cockroach | YugaByte |
Chart: sysbench results (QPS, higher is better) for up to 10 tests
Chart: Normalized performance per core across different databases
Shockingly, the new wave of distributed NewSQL databases lags across the board. On similar hardware, performance can be one order of magnitude behind classic relational databases. The best among them is actually still PolarDB, which uses a classic primary-standby design. This begs the question: Should we re-examine the distributed DB / NewSQL hype?
In theory, distributed databases always trade off complexity (and sometimes stability or functionality) for unlimited scale. But we’re seeing that they often give up a lot of raw performance too. As Donald Knuth famously said: “Premature optimization is the root of all evil.” Opting for a distributed solution for data volumes you don’t actually need (like Google-scale, multi-trillion-row problems) could also be a form of premature optimization. Many real-world workloads never come close to that scale.
TPC-H Analytical Performance
Perhaps the distributed DB argument is: Sure, we’re behind in TP, but we’ll shine in AP. Indeed, many distributed databases pitch an “HTAP” story for big data. So, let’s look at the TPC-H benchmark, used to measure analytical database performance.
TPC-H simulates a data warehouse with 8 tables and 22 complex analytical queries. The performance metric is typically the time to run all 22 queries at a given data scale (often SF=100
, ~100GB). We tested TPC-H with scale factors 1, 10, 50, and 100 on a local laptop and a small AWS instance. Below is the total runtime of the 22 queries:
Scale Factor | Time (s) | CPU | Environment | Comment |
---|---|---|---|---|
1 | 8 | 10 | 10C / 64G | Apple M1 Max |
10 | 56 | 10 | 10C / 64G | Apple M1 Max |
50 | 1,327 | 10 | 10C / 64G | Apple M1 Max |
100 | 4,835 | 10 | 10C / 64G | Apple M1 Max |
1 | 13.5 | 8 | 8C / 64G | z1d.2xlarge |
10 | 133 | 8 | 8C / 64G | z1d.2xlarge |
For a broader view, we compared these results to other databases’ TPC-H data found on official or semi-official tests. Note:
- Some use a different
SF
(not always 100). - Hardware specs differ.
- We’re not always quoting from official sources.
So, it’s only a rough guide:
Database | Time | S | CPU | QPH | Environment | Source |
---|---|---|---|---|---|---|
PostgreSQL | 8 | 1 | 10 | 45.0 | 10C / 64G M1 Max | Vonng |
PostgreSQL | 56 | 10 | 10 | 64.3 | 10C / 64G M1 Max | Vonng |
PostgreSQL | 1,327 | 50 | 10 | 13.6 | 10C / 64G M1 Max | Vonng |
PostgreSQL | 4,835 | 100 | 10 | 7.4 | 10C / 64G M1 Max | Vonng |
PostgreSQL | 13.51 | 1 | 8 | 33.3 | 8C / 64G z1d.2xlarge | Vonng |
PostgreSQL | 133.35 | 10 | 8 | 33.7 | 8C / 64G z1d.2xlarge | Vonng |
TiDB | 190 | 100 | 120 | 15.8 | 120C / 570G | TiDB |
Spark | 388 | 100 | 120 | 7.7 | 120C / 570G | TiDB |
Greenplum | 436 | 100 | 288 | 2.9 | 120C / 570G | TiDB |
DeepGreen | 148 | 200 | 256 | 19.0 | 288C / 1152G | Digoal |
MatrixDB | 2,306 | 1000 | 256 | 6.1 | 256C / 1024G | MXDB |
Hive | 59,599 | 1000 | 256 | 0.2 | 256C / 1024G | MXDB |
StoneDB | 3,388 | 100 | 64 | 1.7 | 64C / 128G | StoneDB |
ClickHouse | 11,537 | 100 | 64 | 0.5 | 64C / 128G | StoneDB |
OceanBase | 189 | 100 | 96 | 19.8 | 96C / 384G | OceanBase |
PolarDB | 387 | 50 | 32 | 14.5 | 32C / 128G | Aliyun |
PolarDB | 755 | 50 | 16 | 14.9 | 16C / 64G | Aliyun |
We introduce the metric QPH = (warehouses per core per hour). That is:
QPH = (1 / Time) * (Warehouses / CPU) * 3600
References
[1] Vonng: PGTPC
[2] WHY MYSQL
[3] MySQL Performance : 1M IO-bound QPS with 8.0 GA on Intel Optane SSD !
[4] MySQL Performance : 8.0 and Sysbench OLTP_RW / Update-NoKEY
[5] MySQL Performance : The New InnoDB Double Write Buffer in Action
[6] TiDB Sysbench Performance Test Report – v6.1.0 vs. v6.0.0
[7] OceanBase 3.1 Sysbench 性能测试报告
[8] Cockroach 22.15 Benchmarking Overview
[9] Benchmark YSQL performance using sysbench (v2.15)
[10] PolarDB-X 1.0 Sysbench 测试说明
[12] Elena Milkai: “How Good is My HTAP System?",SIGMOD ’22 Session 25
[13] AWS Calculator
Why Is PostgreSQL the Most Successful Database?
When we say a database is “successful,” what exactly do we mean? Are we referring to features, performance, or ease of use? Or perhaps total cost, ecosystem, or complexity? There are many evaluation criteria, but in the end, it’s the users—developers—who make the final call.
So, what do developers prefer? Over the past six years, StackOverflow has repeatedly asked over seventy thousand developers across 180 countries three simple questions.
Looking at these survey results over that six-year period, it’s evident that, by 2022, PostgreSQL has claimed the crown in all three categories, becoming the literal “most successful database”:
- PostgreSQL became the most commonly used database among professional developers! (Used)
- PostgreSQL became the most loved database among developers! (Loved)
- PostgreSQL became the most wanted database among developers! (Wanted)
Popularity reflects the current “momentum,” demand points toward “potential energy,” and developer love signals long-term promise. Time and tide now favor PostgreSQL. Let’s take a look at the concrete data behind these results.
Most Popular
PostgreSQL—The Most Popular Database Among Professional Developers! (Used)
The first survey question examines which databases developers are actively using right now—i.e., popularity.
In previous years, MySQL consistently held the top spot as the most popular database, living up to its tagline of being “the world’s most popular open-source relational database.” However, this year, it seems that MySQL has to surrender the crown of “most popular” to PostgreSQL.
Among professional developers, PostgreSQL claimed first place for the first time with a 46.5% usage rate, surpassing MySQL’s 45.7%. These two open-source, general-purpose relational databases dominate the top two spots, significantly outpacing all other databases.
TOP 9 Database Popularity Trends (2017–2022)
PGSQL and MySQL aren’t that far apart. It’s worth noting that among junior developers, MySQL still enjoys a noticeable lead (58.4%). In fact, if we factor in all developer cohorts including juniors, MySQL retains a slim overall lead of 3.3%.
But if you look at the chart below, it’s clear that PostgreSQL is growing at a remarkable pace, whereas other databases—especially MySQL, SQL Server, and Oracle—have been on a steady decline in recent years. As time goes on, PostgreSQL’s advantage will likely become even more pronounced.
Popularity of Four Major Relational Databases Compared
Popularity represents a database’s current market presence (or “momentum”). Affection (“loved” status) signals the potential for future growth.
Most Loved
PostgreSQL—The Most Loved Database Among Developers! (Loved)
The second question StackOverflow asks is about which databases developers love and which they dread. In this survey, PostgreSQL and Redis stand head and shoulders above the rest with over 70% developer affection, significantly outpacing all other databases.
For years, Redis held the title of the most loved database. But in 2022, things changed: PostgreSQL edged out Redis for the first time and became the most loved database among developers. Redis, a super-simple, data-structure-based cache server that pairs well with relational databases, has always been a developer favorite. But apparently, developers love the much more powerful PostgreSQL just a little bit more.
In contrast, MySQL and Oracle lag behind. MySQL is basically split down the middle in terms of those who love or dread it, while only about 35% of users love Oracle—meaning nearly two-thirds of developers dislike it.
TOP 9 Database Affection Trends (2017–2022)
Logically, what people love tends to become what people use; what people dread tends to fade away. If we borrow from the Net Promoter Score (NPS) concept—(Promoters% – Detractors%)—we could define a similar “Net Love Score” (NLS): (Loved% – Dreaded%). We’d expect a positive correlation between a database’s usage growth rate and its NLS.
The data backs this up nicely: PostgreSQL boasts the highest NLS in the chart: 44%, corresponding to a whopping 460 basis-point growth every year. MySQL hovers just above breakeven with an NLS of 2.3%, translating to a modest 36 basis-point annual increase in usage. Oracle, on the other hand, scores a negative 29% NLS, tracking about 44 basis points of annual decline in usage. But it’s not even the most disliked on the list: IBM DB2 sits at an even more dismal -48%, accompanied by an average 46 basis-point annual decline.
Of course, not all potential (love) translates into actual usage growth. People might love it but never actually adopt it. That’s precisely where the third survey question comes in.
Most Wanted
PostgreSQL—The Most Wanted Database Among Developers! (Wanted)
“In the past year, which database environments did you do a lot of development work in? In the coming year, which databases do you want to work with?”
Answers to the first part led us to the “most popular” results. The second part answers the question of “most wanted.” If developer love points to a database’s growth potential, then developers’ actual desire (“want”) is a more tangible measure of next year’s growth momentum.
In this year’s survey, PostgreSQL didn’t hesitate to bump MongoDB from the top spot, becoming developers’ most desired database. A striking 19% of respondents said they want to work in a PostgreSQL environment next year. Following close behind are MongoDB (17%) and Redis (14%). These three lead the pack by a wide margin.
Previously, MongoDB consistently topped the “most wanted” ranking. Lately, though, it seems to be losing steam, for a variety of reasons—PostgreSQL itself being one of them. PostgreSQL has robust JSON support that covers most of the use cases of a document database. And there are projects like FerretDB (formerly MangoDB) that provide MongoDB’s API on top of PG, allowing you to use the same interface with a PostgreSQL engine underneath.
MongoDB and Redis were key players in the NoSQL movement. But unlike MongoDB, Redis continues to see growing demand. PostgreSQL and Redis, leaders in the SQL and NoSQL worlds respectively, are riding strong developer interest and high growth potential. The future looks bright.
Why?
PostgreSQL has come out on top in usage, demand, and developer love. It has the wind at its back on all fronts—past momentum, present energy, future potential. It’s fair to call it the most successful database.
But how did PostgreSQL achieve this level of success?
The secret is hidden in its own slogan: “The world’s most advanced open source relational database.”
A Relational Database
Relational databases are so prevalent and critical that they overshadow all other categories—key-value, document, search, time series, graph, and vector combined likely don’t add up to a fraction of the footprint of relational databases. By default, when people talk about a “database,” they usually mean a relational database. None of the other categories would dare to call themselves “mainstream” by comparison.
Take DB-Engines as an example. DB-Engines ranks databases by combining various signals—search engine results for the database name, Google Trends, Stack Overflow discussions, job postings on Indeed, user profiles on LinkedIn, mentions on Twitter, etc.—to form what you might consider a “composite popularity” metric.
DB-Engines Popularity Trend: https://db-engines.com/en/ranking_trend
From the DB-Engines trend chart, you’ll notice a deep divide. The top four databases are all relational. Including MongoDB in fifth, these five are orders of magnitude ahead of all the rest in terms of popularity. So we really need to focus on those four major relational databases: Oracle, MySQL, SQL Server, and PostgreSQL.
Because they occupy essentially the same ecological niche, relational databases compete in what is almost a zero-sum game. Let’s set aside Microsoft’s SQL Server, which largely lives in its own closed-off commercial ecosystem. Among relational databases, it’s a three-way saga.
Oracle: “talented but unethical,” MySQL: “less capable, less principled,” PostgreSQL: “noble and skillful.”
Oracle is a long-established commercial database with an extensive feature set and historical pedigree, widely chosen by enterprises that “have cash to burn and need someone to take the blame.” It has always been at the top of the database market but is also notorious for expensive licensing and aggressive litigation—earning it a reputation as the corporate world’s “toxic troll.” Microsoft’s SQL Server is basically the same style: a commercial database living within a closed ecosystem. All commercial databases have been feeling the squeeze from open-source alternatives and are slowly losing ground.
MySQL claims second place in overall popularity but finds itself hemmed in on all sides: it’s got an “adoptive father” who doesn’t exactly have its best interests at heart, and “forked offspring” plus new distributed SQL contenders biting at its heels. In rigorous transaction processing and analytics, MySQL is outclassed by PostgreSQL. When it comes to quick-and-dirty solutions, many find NoSQL simpler and more flexible. Meanwhile, Oracle (MySQL’s “foster father”) imposes constraints at the top, MariaDB and others fork from within, and “prodigal children” like TiDB replicate the MySQL interface with new distributed architectures—meaning MySQL is also slipping.
Oracle has impressive talent yet shows questionable “ethics.” MySQL might get partial credit for open source but remains limited in capability—“less capable, less principled.” Only PostgreSQL manages to combine both capability and open ethics, reaping the benefits of advanced technology plus open source licensing. Like they say, “Sometimes the quiet turtle wins the race.” PostgreSQL stayed quietly brilliant for many years, then shot to the top in one fell swoop.
Where does PostgreSQL get its “ethics”? From being open source. And where does it get its “talent”? From being advanced.
The “Open Source” Ethos
PostgreSQL’s “virtue” lies in its open source nature: a founding-father-level open-source project, born of the combined efforts of developers worldwide.
It has a friendly BSD license and a thriving ecosystem that’s branching out in all directions, making it the main flag-bearer in the quest to replace Oracle.
What do we mean by “virtue” or “ethics”? Following the “Way” (道) in software is adhering to open source.
PostgreSQL is an old-guard, foundational project in the open-source world, a shining example of global developer collaboration.
A thriving ecosystem with extensive extensions and distributions—“branching out with many descendants.”
Way back when, developing software or providing any significant IT service usually required outrageously expensive commercial database software, like Oracle or SQL Server. Licensing alone could cost six or seven figures, not to mention hardware and support fees that matched or exceeded those numbers. For example, one CPU core’s worth of Oracle licensing can easily run into tens of thousands of dollars per year. Even giants like Alibaba balked at these costs and started the infamous “IOE phase-out.” The rise of open-source databases such as PostgreSQL and MySQL offered a new choice: free-as-in-beer software.
“Free” open-source software meant you could use the database without paying a licensing fee—a seismic shift that shaped the entire industry. It ushered in an era in which a business might only pay for hardware resources—maybe 20 bucks per CPU core per month—and that was it. Relational databases became accessible even to smaller companies, paving the way for free consumer-facing online services.
Open source has done an immense service to the world. The history of the internet is the history of open source: the reason we have an explosion of free online services is in large part because these services can be built on open-source components. Open source is a real success story—“community-ism,” if you will—where developers own the means of software production, share it freely, and cooperate globally. Everyone benefits from each other’s work.
An open-source programmer can stand on the shoulders of tens of thousands of top developers. Instead of paying a fortune in software licensing, they can simply use and extend what the community provides. This synergy is why developer salaries are relatively high. Essentially, each programmer is orchestrating entire armies of code and hardware. Open source means that fundamental building blocks are public domain, drastically cutting down on repeated “reinventing the wheel” across the industry. The entire tech world gets to move faster.
The deeper and more essential the software layer, the stronger the advantage open source tends to have—an advantage PostgreSQL leverages fully against Oracle.
Oracle may be advanced, but PostgreSQL isn’t far behind. In fact, PostgreSQL is the open-source database that’s most compatible with Oracle, covering about 85% of its features out of the box. Special enterprise distributions of PostgreSQL can reach 96% feature compatibility. Meanwhile, Oracle’s massive licensing costs give PG a huge advantage in cost-effectiveness: you don’t necessarily need to exceed Oracle’s capabilities to win; being 90% as good but an order of magnitude cheaper is enough to topple Oracle.
As an “open-source Oracle,” PostgreSQL is the only database truly capable of challenging Oracle’s dominance. It’s the standard-bearer for “de-Oracle-fication” (去O). Many of the so-called “domestic proprietary databases” (especially in China) are actually based on PostgreSQL under the hood—36% of them, by one estimate. This has created a whole family of “PG descendants” and fueled a wave of “independent controllable” database vendors. PostgreSQL’s BSD licensing does not forbid such derivative works, and the community does not oppose it. This open-minded stance stands in contrast to MySQL, which was purchased by Oracle and remains under the GPL, limiting what you can do with it.
The “Advanced” Edge
PostgreSQL’s “talent” lies in being advanced. A one-stop, all-in-one, multi-purpose database—essentially an HTAP solution by design.
Spatial, GIS, distributed, time-series, documents, it’s all in there. One component can cover nearly every use case.
PostgreSQL’s “talent” is that it’s a multi-specialist. It’s a comprehensive, full-stack database that can handle both OLTP and OLAP (making it naturally HTAP). It can also handle time-series data, geospatial queries (PostGIS), JSON documents, full-text search, and more. Essentially, one PostgreSQL instance can replace up to ten different specialized tools for small to medium enterprises, covering almost all their database needs.
Among relational databases, PostgreSQL is arguably the best value for money: it can handle traditional transactional workloads (OLTP) while also excelling at analytics, which are often an afterthought in other systems. And the specialized features provide entry points into all kinds of industries: geospatial data analytics with PostGIS, time-series financial or IoT solutions with Timescale, streaming pipelines with triggers and stored procedures, full-text search, or the FDW (foreign data wrapper) architecture to unify external data sources. PostgreSQL really is a multi-specialist, going far beyond the typical single-use relational engine.
In many cases, one PostgreSQL node can do the job of multiple specialized systems, significantly reducing complexity and cutting costs. This might mean the difference between needing ten people on your team vs. just one. Of course, this doesn’t mean PG is going to destroy all specialized databases: for large-scale specialized workloads, dedicated components still shine. But for many scenarios—and especially for those not at hyperscale—it’s overkill to maintain extra systems. If a single tool does everything you need, why complicate things?
For example, at Tantan (a major dating/social app in China), a single PostgreSQL setup handled 2.5 million TPS and 200 TB of data reliably. It wore multiple hats—OLTP for transactions, caching, analytics, batch processing, and even a bit of message queue functionality. Eventually, as they scaled to tens of millions of daily active users, these specialized roles did break out into their own dedicated systems—but that was only after the user base had grown enormously.
vs. MySQL
PostgreSQL’s advanced feature set is widely recognized, and this forms its real edge against its long-standing open-source rival—MySQL.
MySQL’s tagline is “the world’s most popular open-source relational database.” Historically, it’s attracted the “quick and dirty” side of the internet developer community. The typical web startup often only needs simple CRUD operations, can tolerate some data inconsistency or loss, and prioritizes time-to-market. They want to spin up solutions quickly—think LAMP stacks—without needing highly specialized DBAs.
But the times have changed. PostgreSQL has improved at a blistering pace, with performance that rivals or surpasses MySQL. What remains of MySQL’s core advantage is its “leniency.” MySQL is known for “better to run than to fail,” or “I don’t care if your data’s messed up as long as I’m not crashing.” For instance, it even allows certain broken SQL commands to run, leading to bizarre results. One of the strangest behaviors is that MySQL may allow partial commits by default, violating the atomicity of transactions that relational databases are supposed to guarantee.
Screenshot: MySQL can silently allow partial transaction commits by default.
The advanced inevitably overtakes the old, and the once-popular eventually fades if it doesn’t evolve. Thanks to its broad, sophisticated features, PostgreSQL has left MySQL behind and is now even surpassing it in popularity. As the saying goes, “When your time comes, the world helps you move forward; when your luck runs out, not even a hero can save you.”
Between Oracle’s advanced but closed nature and MySQL’s open but limited functionality, PostgreSQL is both advanced and open-source, enjoying the best of both worlds—technical edge plus community-driven development. With the stars aligned in its favor, how could it not succeed?
The Road Ahead
“Software is eating the world. Open source is eating software. And the cloud is eating open source.”
So from this vantage point, it seems the database wars are largely settled. No other database engine is likely to seriously challenge PostgreSQL anytime soon. The real threat to the PostgreSQL open-source community no longer comes from another open-source or commercial database engine—it comes from the changing paradigm of software usage itself: the arrival of the cloud.
Initially, you needed expensive commercial software (like Oracle, SQL Server, or Unix) to build or run anything big. Then, open-source took the stage (Linux, PostgreSQL, etc.), offering a choice that was essentially free. Of course, truly getting the most out of open source requires in-house expertise, so companies ended up paying people, rather than licensing fees.
Once your DB scale grows large enough, hiring open-source DBA talent becomes more cost-effective. The challenge is that good DBAs are scarce.
That’s the open-source model: open-source contributors enhance the software for free; the software is free for all to use; users then hire open-source experts to implement and operate it, creating more open-source contributors. It’s a virtuous cycle.
Public cloud vendors, however, have disrupted this cycle by taking open-source software, wrapping it with their cloud hardware and admin tools, and selling it as a cloud service—often returning very little to the upstream open-source project. They’re effectively free-riding on open source, turning it into a service they profit from, which can undermine the community’s sustainability by consolidating the jobs and expertise within a handful of cloud giants, thus eroding software freedom for everyone.
By 2020, the primary enemy of software freedom was no longer proprietary software but cloud-hosted software.
That’s a paraphrase of Martin Kleppmann (author of Designing Data-Intensive Applications), who proposed in his “Local-First Software” movement that cloud-based solutions—like Google Docs, Trello, Slack, Figma, Notion, and crucially, cloud databases—are the new walled gardens.
How should the open-source community respond to the rise of cloud services? The Cloud Native movement offers one possible way forward. It’s a movement to reclaim software freedom from public clouds, and databases are right at the heart of it.
A grand view of the Cloud Native ecosystem—still missing the last piece of the puzzle: robust support for stateful databases!
That’s also our motivation for building Pigsty: an open-source PostgreSQL “distribution” that’s as easy to deploy as RDS or any managed cloud service, but fully under your control!
Pigsty comes with out-of-the-box RDS/PaaS/SaaS integration, featuring an unrivaled PostgreSQL monitoring system and an “auto-driving” high-availability cluster solution. It can be installed with a single command, giving you “Database as Code.” You get an experience on par with, or even better than, managed cloud databases, but you own the data, and the cost can be 50–90% lower. We hope it drastically lowers the barriers to using a good database and helps you get the most out of that database.
Of course, we’re out of space here, so the future of databases in a post-cloud era will have to wait for the next installment.
Pigsty: The Production-Ready PostgreSQL Distribution
What is Pigsty?
Pigsty is a production-ready, batteries-included PostgreSQL distribution.
A distribution, in this context, refers to a complete database solution comprised of the database kernel and a curated set of software packages. Just as Linux is an operating system kernel while RedHat, Debian, and SUSE are operating system distributions built upon it, PostgreSQL is a database kernel, and Pigsty, along with BigSQL, Percona, various cloud RDS offerings, and other database variants are database distributions built on top of it.
Pigsty distinguishes itself from other database distributions through five core features:
- A comprehensive and professional monitoring system
- A stable and reliable deployment solution
- A simple and hassle-free user interface
- A flexible and open extension mechanism
- A free and friendly open-source license
These five features make Pigsty a truly batteries-included PostgreSQL distribution.
Who Should Be Interested?
Pigsty caters to a diverse audience including DBAs, architects, ops engineers, software vendors, cloud providers, application developers, kernel developers, data engineers; those interested in data analysis and visualization; students, junior programmers, and anyone curious about databases.
For DBAs, architects, and other professional users, Pigsty offers a unique professional-grade PostgreSQL monitoring system that provides irreplaceable value for database management. Additionally, Pigsty comes with a stable and reliable, battle-tested production-grade PostgreSQL deployment solution that can automatically deploy database clusters with monitoring, alerting, log collection, service discovery, connection pooling, load balancing, VIP, and high availability in production environments.
For developers (application, kernel, or data), students, junior programmers, and database enthusiasts, Pigsty provides a low-barrier, one-click launch, one-click install local sandbox. This sandbox environment, identical to production except for machine specifications, includes a complete feature set: ready-to-use database instances and monitoring systems. It’s perfect for learning, development, testing, and data analysis scenarios.
Furthermore, Pigsty introduces a flexible extension mechanism called “Datalet”. Those interested in data analysis and visualization might be surprised to find that Pigsty can serve as an integrated development environment for data analysis and visualization. Pigsty integrates PostgreSQL with common data analysis plugins and comes with Grafana and embedded Echarts support, allowing users to write, test, and distribute data mini-applications (Datelets). Examples include “Additional monitoring dashboard packs for Pigsty”, “Redis monitoring system”, “PG log analysis system”, “Application monitoring”, “Data directory browser”, and more.
Finally, Pigsty adopts the free and friendly Apache License 2.0, making it free for commercial use. Cloud providers and software vendors are welcome to integrate and customize it for commercial use, as long as they comply with the Apache 2 License’s attribution requirements.
Comprehensive Professional Monitoring System
You can’t manage what you don’t measure.
— Peter F.Drucker
Pigsty provides a professional-grade monitoring system that delivers irreplaceable value to professional users.
To draw a medical analogy, basic monitoring systems are like heart rate monitors or pulse oximeters - tools that anyone can use without training. They show core vital signs: at least users know if the patient is about to die, but they’re not much help for diagnosis and treatment. Most monitoring systems provided by cloud vendors and software companies fall into this category: a dozen core metrics that tell you if the database is alive, giving you a rough idea and nothing more.
A professional-grade monitoring system, on the other hand, is more like a CT or MRI machine, capable of examining every detail inside the subject. Professional physicians can quickly identify diseases and potential issues from CT/MRI reports: treating what’s broken and maintaining what’s healthy. Pigsty can scrutinize every table, every index, every query in each database, providing comprehensive metrics (1,155 types) and transforming them into insights through thousands of dashboards: killing problems in their infancy and providing real-time feedback for performance optimization.
Pigsty’s monitoring system is built on industry best practices, using Prometheus and Grafana as monitoring infrastructure. It’s open-source, customizable, reusable, portable, and free from vendor lock-in. It can integrate with various existing database instances.
Stable and Reliable Deployment Solution
A complex system that works is invariably found to have evolved from a simple system that works.
—John Gall, Systemantics (1975)
If databases are software for managing data, then control systems are software for managing databases.
Pigsty includes a database control solution centered around Ansible, wrapped with command-line tools and a graphical interface. It integrates core database management functions: creating, destroying, and scaling database clusters; creating users, databases, and services. Pigsty adopts an “Infrastructure as Code” design philosophy using declarative configuration, describing and customizing databases and runtime environments through numerous optional configuration parameters, and automatically creating required database clusters through idempotent preset playbooks, providing a private cloud-like experience.
Pigsty creates distributed, highly available database clusters. Built on DCS, Patroni, and HAProxy, Pigsty’s database clusters achieve high availability. Each database instance in the cluster is idempotent in usage - any instance can provide complete read-write services through built-in load balancing components, delivering a distributed database experience. Database clusters can automatically detect failures and perform primary-replica failover, with common failures self-healing within seconds to tens of seconds, during which read-only traffic remains unaffected. During failures, as long as any instance survives, the cluster can provide complete service.
Pigsty’s architecture has been carefully designed and evaluated, focusing on achieving required functionality with minimal complexity. This solution has been validated through long-term, large-scale production environment use across internet/B/G/M/F industries.
Simple and Hassle-Free User Interface
Pigsty aims to lower PostgreSQL’s barrier to entry and has invested heavily in usability.
Installation and Deployment
Someone told me that each equation I included in the book would halve the sales.
— Stephen Hawking
Pigsty’s deployment consists of three steps: download source code, configure environment, and execute installation - all achievable through single commands. Following classic software installation patterns and providing a configuration wizard, all you need is a CentOS 7.8 machine with root access. For managing new nodes, Pigsty uses Ansible over SSH, requiring no agent installation, making deployment easy even for beginners.
Pigsty can manage hundreds or thousands of high-spec production nodes in production environments, or run independently on a local 1-core 1GB virtual machine as an out-of-the-box database instance. For local computer use, Pigsty provides a sandbox based on Vagrant and VirtualBox. It can spin up a database environment identical to production with one click, perfect for learning, development, testing, data analysis, and data visualization scenarios.
User Interface
Clearly, we must break away from the sequential and not limit the computers. We must state definitions and provide for priorities and descriptions of data. We must state relationships, not procedures.
—Grace Murray Hopper, Management and the Computer of the Future (1962)
Pigsty incorporates the essence of Kubernetes architecture design, using declarative configuration and idempotent operation playbooks. Users only need to describe “what kind of database they want” without worrying about how Pigsty creates or modifies it. Based on the user’s configuration manifest, Pigsty will create the required database cluster from bare metal nodes in minutes.
For management and usage, Pigsty provides different levels of user interfaces to meet various user needs. Novice users can use the one-click local sandbox and graphical user interface, while developers might prefer the pigsty-cli
command-line tool and configuration files for management. Experienced DBAs, ops engineers, and architects can directly control task execution through Ansible primitives for fine-grained control.
Flexible and Open Extension Mechanism
PostgreSQL’s extensibility has always been praised, with various extension plugins making it the most advanced open-source relational database. Pigsty respects this value and provides an extension mechanism called “Datalet”, allowing users and developers to further customize Pigsty and use it in “unexpected” ways, such as data analysis and visualization.
When we have a monitoring system and control solution, we also have an out-of-the-box visualization platform Grafana and a powerful database PostgreSQL. This combination packs a punch - especially for data-intensive applications. Users can perform data analysis and visualization, create data application prototypes with rich interactions, or even full applications without writing frontend or backend code.
Pigsty integrates Echarts and common map tiles, making it convenient to implement advanced visualization requirements. Compared to traditional scientific computing languages/plotting libraries like Julia, Matlab, and R, the PG + Grafana + Echarts combination allows you to create shareable, deliverable, standardized data applications or visualization works at minimal cost.
Pigsty’s monitoring system itself is a prime example of Datalet: all Pigsty advanced monitoring dashboards are published as Datelets. Pigsty also comes with some interesting Datalet examples: Redis monitoring system, COVID-19 data analysis, China’s seventh census population data analysis, PG log mining, etc. More out-of-the-box Datelets will be added in the future, continuously expanding Pigsty’s functionality and application scenarios.
Free and Friendly Open Source License
Once open source gets good enough, competing with it would be insane.
Larry Ellison —— Oracle CEO
In the software industry, open source is a major trend. The history of the internet is the history of open source software. One key reason why the IT industry is so prosperous today and people can enjoy so many free information services is open source software. Open source is a truly successful form of communism (better translated as communitarianism) composed of developers: software, the core means of production in the IT industry, becomes collectively owned by developers worldwide - all for one and one for all.
When an open source programmer works, their labor potentially embodies the wisdom of tens of thousands of top developers. Through open source, all community developers form a joint force, greatly reducing the waste of reinventing wheels. This has pushed the industry’s technical level forward at an incredible pace. The momentum of open source is like a snowball, unstoppable today. Except for some special scenarios and path dependencies, developing software behind closed doors and striving for self-reliance has become a joke.
Relying on open source and giving back to open source, Pigsty adopts the friendly Apache License 2.0, free for commercial use. Cloud providers and software vendors are welcome to integrate and customize it for commercial use, as long as they comply with the Apache 2 License’s attribution requirements.
About Pigsty
A system cannot be successful if it is too strongly influenced by a single person. Once the initial design is complete and fairly robust, the real test begins as people with many different viewpoints undertake their own experiments. — Donald Knuth
Pigsty is built around the open-source database PostgreSQL, the most advanced open-source relational database in the world, and Pigsty’s goal is to be the most user-friendly open-source PostgreSQL distribution.
Initially, Pigsty didn’t have such grand ambitions. Unable to find any monitoring system that met my needs in the market, I had to roll up my sleeves and build one myself. Surprisingly, it turned out better than expected, and many external PostgreSQL users wanted to use it. Then, deploying and delivering the monitoring system became an issue, so the database deployment and control parts were added; after production deployment, developers wanted local sandbox environments for testing, so the local sandbox was created; users found Ansible difficult to use, so the pigsty-cli
command-line tool was developed; users wanted to edit configuration files through UI, so Pigsty GUI was born. This way, as demands grew, features became richer, and Pigsty became more refined through long-term polishing, far exceeding initial expectations.
This project itself is a challenge - creating a distribution is somewhat like creating a RedHat, a SUSE, or an “RDS product”. Usually, only professional companies and teams of certain scale would attempt this. But I wanted to try: could one person do it? Actually, except for being slower, there’s nothing impossible about it. It’s an interesting experience switching between product manager, developer, and end-user roles, and the biggest advantage of “eating your own dog food” is that you’re both developer and user - you understand what you need and won’t cut corners on your own requirements.
However, as Donald Knuth said, “A system cannot be successful if it is too strongly influenced by a single person.” To make Pigsty a project with vigorous vitality, it must be open source, letting more people use it. “Once the initial design is complete and fairly robust, the real test begins as people with many different viewpoints undertake their own experiments.”
Pigsty has well solved my own problems and needs, and now I hope it can help more people and make the PostgreSQL ecosystem more prosperous and colorful.
Why PostgreSQL Has a Bright Future
Recently, everything I’ve been working on revolves around the PostgreSQL ecosystem, because I’ve always believed it’s a direction with limitless potential.
Why do I say this? Because databases are the core components of information systems, relational databases are the absolute workhorses among databases, and PostgreSQL is the most advanced open-source relational database in the world. With timing and positioning in its favor, how could greatness not be achieved?
The most important thing when doing anything is to understand the situation clearly. When the time is right, everything aligns in your favor; when it’s not, even heroes are powerless.
The Big Picture
Today’s database world is divided in three parts, with Oracle | MySQL | SQL Server in decline, their sun setting in the west. PostgreSQL follows closely behind, rising like the midday sun. Among the top four databases, the first three are heading downhill, while only PG continues to grow unabated. As one falls and another rises, the future looks boundless.
DB-Engine Database Popularity Trend (Note that this is a logarithmic scale)
Between the only two leading open-source relational databases, MySQL (2nd) holds the upper hand, but its ecological niche is gradually being encroached upon by both PostgreSQL (4th) and the non-relational document database MongoDB (5th). At the current pace, PostgreSQL’s popularity will soon break into the top three, standing shoulder to shoulder with Oracle and MySQL.
Competitive Landscape
Relational databases occupy highly overlapping ecological niches, and their relationship can be viewed as a zero-sum game. The direct competitors to PostgreSQL are Oracle and MySQL.
Oracle ranks first in popularity, an established commercial database with deep historical and technical foundations, rich features, and comprehensive support. It sits firmly in the top database chair, beloved by organizations with deep pockets. But Oracle is expensive, and its litigious behavior has made it a notorious industry parasite. SQL Server, ranking third, belongs to the relatively independent Microsoft ecosystem and is similar to Oracle in nature—both are commercial databases. Overall, commercial databases are experiencing slow decline due to pressure from open-source alternatives.
MySQL ranks second in popularity but finds itself in an unfavorable position, caught between wolves ahead and tigers behind, with a domineering parent above and rebellious offspring below. For rigorous transaction processing and data analysis, MySQL lags several streets behind fellow open-source relational database PostgreSQL. For quick and dirty agile methods, MySQL can’t compete with emerging NoSQL solutions. Meanwhile, MySQL faces suppression from its Oracle parent, competition from its MariaDB fork, and market share erosion from MySQL-compatible newcomers like TiDB and OceanBase. As a result, it has stagnated.
Only PostgreSQL is surging forward, maintaining nearly exponential growth. If a few years ago PG’s momentum was merely “potential,” that potential is now being realized as “impact,” posing a serious challenge to competitors.
In this life-or-death struggle, PostgreSQL holds three key advantages:
-
The spread of open-source software, eating away at the commercial software market
Against the backdrop of “de-IOE” (eliminating IBM, Oracle, EMC) and the open-source wave, the open-source ecosystem has effectively suppressed commercial software (Oracle).
-
Meeting users’ growing data processing requirements
With PostGIS, the de facto standard for geospatial data, PostgreSQL has established an unbeatable position, while its Oracle-comparable rich feature set gives it a technical edge over MySQL.
-
Market share regression to the mean
PG’s market share in China is far below the global average for historical reasons, which harbors enormous potential energy.
Oracle, as an established commercial software, unquestionably has talent, while as an industry parasite, its virtue needs no further comment—hence, “talented but lacking virtue.” MySQL has the virtue of being open-source, but it adopted the GPL license, which is less generous than PostgreSQL’s permissive BSD license, plus it was acquired by Oracle (accepting a thief as father), and it’s technically shallow and functionally crude—hence, “shallow talent, thin virtue.”
When virtue doesn’t match position, disaster inevitably follows. Only PostgreSQL occupies the right time with the rise of open source, the right place with powerful features, and the right people with its permissive BSD license. As the saying goes: “Store up your capabilities, act when the time is right. Silent until ready, then make a thunderous entrance.” With both virtue and talent, the advantage in both offense and defense is clear!
Virtue and Talent Combined
PostgreSQL’s Virtue
PG’s “virtue” lies in being open source. What is “virtue”? It’s behavior that conforms to the “Way.” And this “Way” is open source.
PG itself is a founding-level open-source software, a jewel in the open-source world, a successful example of global developer collaboration. More importantly, it uses the selfless BSD license: aside from fraudulently using the PG name, basically everything is permitted—including rebranding it as a domestic database for sale. PG can truly be called the bread and butter of countless database vendors. With countless descendants and beneficiaries, its merit is immeasurable.
Database genealogy chart. If all PostgreSQL derivatives were listed, this chart would likely explode.
PostgreSQL’s Talent
PG’s “talent” lies in being versatile while specialized. PostgreSQL is a full-stack database that excels in many areas, born as an HTAP, hyper-converged database that can do the work of ten. A single component can cover most database needs for small and medium enterprises: OLTP, OLAP, time-series, spatial GIS, full-text search, JSON/XML, graph databases, caching, and more.
PostgreSQL can play the role of a jack-of-all-trades within a considerable scale, using a single component where multiple would normally be needed. And a single data component selection can greatly reduce project complexity, which means significant cost savings. It turns what would require ten talented people into something one person can handle. If there’s truly a technology that can meet all your needs, using that technology is the best choice, rather than trying to re-implement it with multiple components.
Recommended reading: What’s Good About PG
The Virtue of Open Source
Open source has great virtue. The history of the internet is the history of open-source software. One of the core reasons the IT industry has today’s prosperity, allowing people to enjoy so many free information services, is open-source software. Open source is a truly successful form of communism (better translated as communitarianism) made up of developers: software, the core means of production in the IT industry, becomes the common property of developers worldwide—everyone for me, me for everyone.
When an open-source programmer works, their labor potentially embodies the crystallized wisdom of tens of thousands of top developers. Internet programmers are valuable because, in effect, they aren’t workers but foremen commanding software and machines. Programmers themselves are the core means of production, servers are easy to obtain (compared to research equipment and experimental environments in other industries), software comes from the public community, and one or a few senior software engineers can easily use the open-source ecosystem to quickly solve domain problems.
Through open source, all community developers join forces, greatly reducing the waste of reinventing wheels. This has propelled the entire industry’s technical level forward at an unimaginable speed. The momentum of open source is like a snowball, becoming unstoppable today. Basically, except for some special scenarios and path dependencies, developing software behind closed doors has become almost a joke.
So, whether in databases or software in general, if you want to work with technology, work with open-source technology. Closed-source things have too weak a vitality to be interesting. The virtue of open source is also PostgreSQL and MySQL’s greatest advantage over Oracle.
The Ecosystem Battle
The core of open source lies in the ecosystem (ECO). Every open-source technology has its own small ecosystem. An ecosystem is a system formed by various entities and their environment through intensive interactions. The open-source software ecosystem model can be described as a positive feedback loop consisting of three steps:
- Open-source software developers contribute to open-source software
- Open-source software itself is free, attracting more users
- Users use open-source software, generating demand and creating more open-source software-related jobs
The prosperity of an open-source ecosystem depends on this closed loop, and the scale (number of users/developers) and complexity (quality of users/developers) of the ecosystem directly determine the vitality of the software. Therefore, every piece of open-source software has a mandate to expand its scale. The scale of software usually depends on the ecological niche it occupies, and if different software occupy overlapping niches, competition occurs. In the ecological niche of open-source relational databases, PostgreSQL and MySQL are the most direct competitors.
Popular vs. Advanced
MySQL’s slogan is “The world’s most popular open-source relational database,” while PostgreSQL’s is “The world’s most advanced open-source relational database"—clearly a pair of old rivals. These two slogans nicely reflect the qualities of the two products: PostgreSQL is feature-rich, consistency-first, high-end, and academically rigorous; MySQL is feature-sparse, availability-first, quick and dirty, with an “engineering” approach.
MySQL’s primary user base is concentrated in internet companies. What are the typical characteristics of internet companies? They pursue trends with a “quick and dirty” approach. Quick because internet companies have simple business scenarios (mostly CRUD); data importance is low, unlike traditional industries (e.g., banks) that care deeply about data consistency (correctness); and availability is prioritized (they can tolerate data loss or errors more than service outages, while some traditional industries would rather stop service than have accounting errors). Dirty refers to the large volumes of data at internet companies—they need cement mixer trucks, not high-speed trains or manned spacecraft. Fast means internet companies have rapidly changing requirements, short delivery cycles, and demand quick response times, requiring out-of-the-box software suites (like LAMP) and CRUD developers who can get to work after minimal training. So the quick-and-dirty internet companies and quick-and-dirty MySQL are a perfect match.
PG users, meanwhile, tend toward traditional industries. Traditional industries are called “traditional” because they’ve already gone through the wild growth phase and have mature business models with deep foundations. They need correct results, stable performance, rich features, and the ability to analyze, process, and refine data. So in traditional industries, Oracle, SQL Server, and PostgreSQL dominate, with PostgreSQL having an irreplaceable position especially in geography-related scenarios. At the same time, many internet companies’ businesses are beginning to mature and settle, with one foot already in the “traditional industry” door. More and more internet companies are escaping the quick-and-dirty low-level loop, turning their attention to PostgreSQL.
Which is More Correct?
Those who understand a person best are often their competitors. PostgreSQL and MySQL’s slogans precisely target each other’s pain points. PG’s “most advanced” implies MySQL is too backward, while MySQL’s “most popular” says PG isn’t popular. Few users but advanced, many users but backward. Which is “better”? Such value judgments are difficult to answer.
But I believe time stands on the side of advanced technology: because advanced versus backward is the core measure of technology—it’s the cause, while popularity is the effect. Popularity is the result of internal factors (how advanced the technology is) and external factors (historical path dependencies) integrated over time. Today’s causes will be reflected in tomorrow’s effects: popular things become outdated because they’re backward, while advanced things become popular because they’re advanced.
While many popular things are garbage, popularity doesn’t necessarily mean backwardness. If MySQL merely lacked some features, it wouldn’t be labeled “backward.” The problem is that MySQL is so crude it has flaws in transactions, a basic feature of relational databases, which isn’t a question of backwardness but of qualification.
ACID
Some authors argue that supporting generalized two-phase commit is too expensive and causes performance and availability problems. It’s much better to have programmers deal with performance problems due to overuse of transactions than to have them program without transactions. — James Corbett et al., Spanner: Google’s Globally-Distributed Database (2012)
In my view, MySQL’s philosophy can be described as: “Better a bad life than a good death” and “After me, the flood.” Its “availability” is reflected in various “fault tolerances,” such as allowing erroneous SQL queries written by amateur programmers to run anyway. The most outrageous example is that MySQL actually allows partially successful transactions to commit, which violates the basic constraints of relational databases: atomicity and data consistency.
Image: MySQL actually allows partially successful transaction commits
Here, two records are inserted in a transaction, the first succeeding and the second failing due to a constraint violation. According to transaction atomicity, the entire transaction should either succeed or fail (with no records inserted in the end). But MySQL’s default behavior actually allows partially successful transactions to commit, meaning the transaction has no atomicity, and without atomicity, there is no consistency. If this transaction were a transfer (debit first, then credit) that failed for some reason, the accounts would be unbalanced. Using such a database for accounting would probably result in a mess, so the notion of “financial-grade MySQL” is likely a joke.
Of course, hilariously, some MySQL users call this a “feature,” saying it demonstrates MySQL’s fault tolerance. In reality, such “special fault tolerance” requirements can be perfectly implemented through the SAVEPOINT mechanism in the SQL standard. PG’s implementation is exemplary—the psql client allows the ON_ERROR_ROLLBACK
option to implicitly create a SAVEPOINT
after each statement and automatically ROLLBACK TO SAVEPOINT
when a statement fails, achieving the same seemingly convenient but actually compromising functionality using standard SQL, as a client-side option, without sacrificing ACID. In comparison, MySQL’s so-called “feature” comes at the cost of directly sacrificing transaction ACID properties by default at the server level (meaning users using JDBC, psycopg, and other application drivers are equally affected).
For internet businesses, losing a user’s avatar or comment during registration might not be a big deal. With so much data, what’s a few lost or incorrect records? Not only data, but the business itself might be in precarious condition, so why care about being crude? If it succeeds, someone else will clean up the mess later anyway. So many internet companies typically don’t care about these issues.
PostgreSQL’s so-called “strict constraints and syntax” might seem “unfriendly” to newcomers. For example, if a batch of data contains a few dirty records, MySQL might accept them all, while PG would strictly reject them. Although compromise might seem easier, it plants landmines elsewhere: engineers working overtime to troubleshoot logical bombs and data analysts forced to clean dirty data daily will certainly have complaints. In the long run, to be successful, doing the right thing is most important.
For a technology to succeed, reality must take precedence over public relations. You can fool others, but you can’t fool natural laws.
— Rogers Commission Report (1986)
MySQL’s popularity isn’t that far ahead of PG, yet its functionality lags significantly behind PostgreSQL and Oracle. Oracle and PostgreSQL were born around the same time and, despite their battles from different positions and camps, have a mutual respect as old rivals: both solid practitioners who have honed their internal skills for half a century, accumulating strength steadily. MySQL, on the other hand, is like an impetuous twenty-something youngster playing with knives and guns, relying on brute force and riding the golden two decades of wild internet growth to seize a kingdom.
The benefits bestowed by an era also recede with the era’s passing. In this time of transformation, without advanced features as a foundation, “popularity” may not last long.
Development Prospects
From a personal career development perspective, many programmers learn a technology to enhance their technical competitiveness (and thereby earn more money). PostgreSQL is the most cost-effective choice among relational databases: it can not only handle traditional CRUD OLTP business, but data analysis is its specialty. Its various special features provide opportunities to enter multiple industries: geographic spatiotemporal data processing and analysis based on PostGIS, time-series financial and IoT data processing based on Timescale, stream processing based on Pipeline stored procedures and triggers, search engines based on inverted index full-text search, and FDW for connecting various external data sources. It’s truly a versatile full-stack database, capable of implementing much richer functionality than a pure OLTP database, providing CRUD coders with paths for transformation and advancement.
From the enterprise user perspective, PostgreSQL can independently play multiple roles within a considerable scale, using one component where multiple would normally be needed. And a single data component selection can greatly reduce project complexity, which means significant cost savings. It turns what would require ten talented people into something one person can handle. Of course, this doesn’t mean PG will be one-against-ten and overturn all other databases’ bowls—professional components’ strengths in their domains are undeniable. But never forget, designing for scale you don’t need is wasted effort, actually a form of premature optimization. If there’s truly a technology that can meet all your needs, using that technology is the best choice, rather than trying to re-implement it with multiple components.
Taking Tantan as an example, at a scale of 2.5 million TPS and 200TB of data, a single PostgreSQL deployment still supports the business rock-solid. Being versatile for a considerable scale, PG served not only its primary OLTP role but also, for quite some time, as cache, OLAP, batch processing, and even message queue. Of course, even the divine turtle has a lifespan. Eventually, these secondary functions were gradually split off to be handled by specialized components, but that was only after approaching ten million daily active users.
From the business ecosystem perspective, PostgreSQL also has huge advantages. First, PG is technologically advanced, earning the nickname “open-source Oracle.” Native PG can achieve 80-90% compatibility with Oracle’s functionality, while EDB has a professional PG distribution with 96% Oracle compatibility. Therefore, in capturing market share from the Oracle exodus, PostgreSQL and its derivatives have overwhelming technical advantages. Second, PG’s protocol is friendly, using the permissive BSD license. As a result, various database vendors and cloud providers’ “self-developed databases” and many “cloud databases” are largely based on modified PostgreSQL. For example, Huawei’s recent move to create openGaussDB based on PostgreSQL is a very wise choice. Don’t misunderstand—PG’s license explicitly allows this, and such actions actually make the PostgreSQL ecosystem more prosperous and robust. Selling PostgreSQL derivatives is a mature market: traditional enterprises don’t lack money and are willing to pay for it. The genius fire of open source, fueled by commercial interests, continuously releases vigorous vitality.
vs MySQL
As an old rival, MySQL’s situation is somewhat awkward.
From a personal career development perspective, learning MySQL is primarily for CRUD work. Learning to handle create, read, update, and delete operations to become a qualified coder is fine, but who wants to keep doing “data mining” work forever? Data analysis is where the lucrative positions are in the data industry chain. With MySQL’s weak analytical capabilities, it’s difficult for CRUD programmers to upgrade and transform. Additionally, PostgreSQL market demand is there but currently faces supply shortage (leading to numerous PG training institutions of varying quality springing up like mushrooms after rain). It’s true that MySQL professionals are easier to recruit than PG professionals, but conversely, the degree of competition in the MySQL world is much greater—supply shortage reflects scarcity, and when there are too many people, skills devalue.
From the enterprise user perspective, MySQL is a single-function component specialized for OLTP, often requiring ES, Redis, MongoDB, and others to satisfy complete data storage needs, while PG basically doesn’t have this problem. Furthermore, both MySQL and PostgreSQL are open-source databases, both “free.” Between a free Oracle and a free MySQL, which would users choose?
From a business ecosystem perspective, MySQL’s biggest problem is that it gets praise but not purchases. It gets praise because the more popular it is, the louder the voice, especially since its main users—internet companies—occupy the high ground of discourse. Not getting purchases is also because internet companies themselves have extremely weak willingness to pay for such software: any way you calculate it, hiring a few MySQL DBAs and using the open-source version is more cost-effective. Additionally, because MySQL’s GPL license requires derivative software to be open source, software vendors have weak motivation to develop based on MySQL. Most adopt a “MySQL-compatible” protocol approach to share MySQL’s market cake, rather than developing based on MySQL’s code and contributing back, raising doubts about its ecosystem’s health.
Of course, MySQL’s biggest problem is that its ecological niche is increasingly narrow. For rigorous transaction processing and data analysis, PostgreSQL leaves it streets behind; for quick and dirty prototyping, NoSQL solutions are far more convenient than MySQL. For commercial profit, it has Oracle daddy suppressing it from above; for open-source ecosystem, it constantly faces new MySQL-compatible products trying to replace it. MySQL can be said to be in a position of living off past success, maintaining its current status only through historical accumulated points. Whether time will stand on MySQL’s side remains to be seen.
vs NewSQL
Recently, there have been some eye-catching NewSQL products on the market, such as TiDB, Cockroachdb, Yugabytedb, etc. How about them? I think they’re all good products with some nice technical highlights, all contributing to open-source technology. But they may also face the same praised but not purchased dilemma.
The general characteristics of NewSQL are: emphasizing the concept of “distributed,” using “distributed” to solve horizontal scalability and disaster recovery and high availability issues, and sacrificing many features due to the inherent limitations of distribution, providing only relatively simple and limited query support. Distributed databases don’t have a qualitative difference from traditional master-slave replication in terms of high availability and disaster recovery, so their features can mainly be summarized as “quantity over quality.”
However, for many enterprises, sacrificing functionality for scalability is likely a false requirement or weak requirement. Among the not-few users I’ve encountered, the data volume and load level in the vast majority of scenarios fall completely within single-machine Postgres’s processing range (the current record being 15TB in a single database, 400,000 TPS in a single cluster). In terms of data volume, the vast majority of enterprises won’t exceed this bottleneck throughout their lifecycle; as for performance, it’s even less important—premature optimization is the root of all evil, and many enterprises have enough DB performance margin to happily run all their business logic as stored procedures in the database.
NewSQL’s founding father, Google Spanner, was created to solve massive data scalability problems, but how many enterprises have Google’s business data volume? Probably only typical internet companies or certain parts of some large enterprises would have such scale of data storage needs. So like MySQL, NewSQL’s problem comes back to the fundamental question of who will pay. In the end, it’s probably only investors and state-owned asset commissions who will pay.
But at the very least, NewSQL’s attempts are always praiseworthy.
vs Cloud Databases
“I want to say bluntly: For years, we’ve been like idiots, and they’ve made a fortune off what we developed”.
— Ofer Bengal, Redis Labs CEO
Another noteworthy “competitor” is the so-called cloud database, including two types: one is open-source databases hosted in the cloud, such as RDS for PostgreSQL, and the other is self-developed new-generation cloud databases.
For the former, the main issue is “cloud vendor bloodsucking.” If cloud vendors sell open-source software, it will cause open-source software-related positions and profits to concentrate toward cloud vendors, and whether cloud vendors allow their programmers to contribute to open-source projects, and how much they contribute, is actually hard to say. Responsible major vendors usually give back to the community and ecosystem, but this depends on their conscience. Open-source software should keep its destiny in its own hands, preventing cloud vendors from growing too large and forming monopolies. Compared to a few monopolistic giants, multiple scattered small groups can provide greater ecosystem diversity, more conducive to healthy ecosystem development.
Gartner claims 75% of databases will be deployed to cloud platforms by 2022—this boast is too big. (But there are ways to rationalize it, after all, one machine can easily create hundreds of millions of sqlite file databases, would that count?). Because cloud computing can’t solve a fundamental problem—trust. In commercial activities, how technically impressive something is is a very secondary factor; trust is key. Data is the lifeline of many enterprises, and cloud vendors aren’t truly neutral third parties. Who can guarantee data won’t be peeked at, stolen, leaked, or even directly shut down by having their necks squeezed (like various cloud vendors hammered Parler)? Transparent encryption solutions like TDE are chicken ribs, thoroughly annoying yourself but unable to stop those with real intent. Perhaps we’ll have to wait for truly practical efficient fully homomorphic encryption technology to mature before solving the trust and security problem.
Another fundamental issue is cost: Given current cloud vendor pricing strategies, cloud databases only have advantages at micro-scale. For example, a high-end D740 machine with 64 cores, 400GB memory, 3TB PCI-E SSD has a four-year comprehensive cost of at most 150,000 yuan. However, the largest RDS specification I could find (much worse, 32 cores, 128GB) costs that much for just one year. As soon as data volume and node count rise even slightly, hiring a DBA and building your own becomes far more cost-effective.
The main advantage of cloud databases is management—essentially convenience, point-and-click. Daily operational functions are fairly comprehensively covered, with some basic monitoring support. In short, there’s a minimum standard—if you can’t find reliable database talent, using a cloud database at least won’t cause too many bizarre issues. However, while these management software are good, they’re basically closed-source and deeply bound to their vendors.
If you’re looking for an open-source one-stop PostgreSQL monitoring and management solution, why not try Pigsty.
The latter type of cloud database, represented by AWS Aurora, includes a series of similar products like Alibaba Cloud PolarDB and Tencent Cloud CynosDB. Basically, they all use PostgreSQL and MySQL as the base and protocol layer, customized based on cloud infrastructure (shared storage, S3, RDMA), optimizing scaling speed and performance. These products certainly have novelty and creativity in technology. But the soul-searching question is, what are the benefits of these products compared to using native PostgreSQL? The immediate visible benefit is that cluster expansion is much faster (from hours to 5 minutes), but compared to the high fees and vendor lock-in issues, it really doesn’t scratch where it itches.
Overall, cloud databases pose a limited threat to native PostgreSQL. There’s no need to worry too much about cloud vendors—they’re generally part of the open-source software ecosystem and contribute to the community and ecosystem. Making money isn’t shameful—when everyone makes money, there’s more spare capacity for public good, right?
Abandoning Darkness for Light?
Typically, Oracle programmers transitioning to PostgreSQL don’t have much baggage, as the two are functionally similar and most experience is transferable. In fact, many members of the PostgreSQL ecosystem are former Oracle camp members who switched to PG. For example, EnmoTech, a renowned Oracle service provider in China (founded by Gai Guoqiang, China’s first Oracle ACE Director), publicly announced last year that they were “entering the arena with humility” to embrace PostgreSQL.
There are also quite a few who’ve switched from the MySQL camp to PostgreSQL. These users have the deepest sense of the differences between the two: basically all with an attitude of “wish I’d found you sooner” and “abandoning darkness for light.” Actually, I myself started with MySQL 😆, but embraced PostgreSQL once I could choose my own stack. However, some veteran programmers have formed deep interest bindings with MySQL, shouting about how great MySQL is while not forgetting to come over and bash PostgreSQL (referring to someone specific). This is actually understandable—touching interests is harder than touching souls, and it’s certainly frustrating to see one’s skilled technology setting in the west 😠. After all, having invested so many years in MySQL, no matter how good PostgreSQL 🐘 is, asking me to abandon my beloved little dolphin 🐬 is impossible.
However, newcomers to the industry still have the opportunity to choose a brighter path. Time is the fairest judge, and the choices of the new generation are the most representative benchmarks. According to my personal observation, among the emerging and very vibrant Golang developer community, PostgreSQL’s popularity is significantly higher than MySQL’s. Many startup and innovative companies now choose Go+Pg as their technology stack, such as Instagram, TanTan, and Apple all using Go+PG.
I believe the main reason for this phenomenon is the rise of new-generation developers. Go is to Java as PostgreSQL is to MySQL. The new wave pushes the old wave forward—this is actually the core mechanism of evolution—metabolism. Go and PostgreSQL are slowly flattening Java and MySQL, but of course Go and PostgreSQL may also be flattened in the future by the likes of Rust and some truly revolutionary NewSQL databases. But fundamentally, in technology, we should pursue those with bright prospects, not those setting in the west. (Of course, diving in too early and becoming a martyr isn’t appropriate either). Look at what new-generation developers are using, what vibrant startups, new projects, and new teams are using—these can’t be wrong.
PG’s Problems
Of course, does PostgreSQL have its own problems? Certainly—popularity.
Popularity relates to user scale, trust level, number of mature cases, amount of effective demand feedback, number of developers, and so on. Although given the current popularity development trend, PG will surpass MySQL in a few years, so from a long-term perspective, I don’t think this is a problem. But as a member of the PostgreSQL community, I believe it’s very necessary to do some things to further secure this success and accelerate this progress. And the most effective way to make a technology more popular is to: lower the threshold.
So, I created an open-source software called Pigsty, aiming to smash the deployment, monitoring, management, and usage threshold of PostgreSQL from the ceiling to the floor. It has three core goals:
- Create the most top-notch, professional open-source PostgreSQL monitoring system (like tidashboard)
- Create the lowest-threshold, most user-friendly open-source PostgreSQL management solution (like tiup)
- Create an out-of-the-box integrated development environment for data analysis & visualization (like minikube)
Of course, details are limited by length and won’t be expanded here. Details will be left for the next article.
What Makes PostgreSQL So Awesome?
PostgreSQL’s slogan is “The World’s Most Advanced Open Source Relational Database”, but I find this tagline lacks punch. It also feels like a direct jab at MySQL’s “The World’s Most Popular Open Source Relational Database” - a bit too much like riding on their coattails. If you ask me, the description that truly captures PG’s essence would be: The Full-Stack Database That Does It All - one tool to rule them all.
The Full-Stack Database
Mature applications typically rely on numerous data components and functions: caching, OLTP, OLAP/batch processing/data warehousing, stream processing/message queuing, search indexing, NoSQL/document databases, geographic databases, spatial databases, time-series databases, and graph databases. Traditional architecture selection usually combines multiple components - typically something like Redis + MySQL + Greenplum/Hadoop + Kafka/Flink + ElasticSearch. This combo can handle most requirements, but the real headache comes from integrating these heterogeneous systems: endless boilerplate code just shuffling data from Component A to Component B.
In this ecosystem, MySQL can only play the role of an OLTP relational database. PostgreSQL, however, can wear multiple hats and handle them all:
-
OLTP: Transaction processing is PostgreSQL’s bread and butter
-
OLAP: Citus distributed plugin, ANSI SQL compatibility, window functions, CTEs, CUBE and other advanced analytics features, UDFs in any language
-
Stream Processing: PipelineDB extension, Notify-Listen, materialized views, rules system, and flexible stored procedures and functions
-
Time-Series Data: TimescaleDB plugin, partitioned tables, BRIN indices
-
Spatial Data: PostGIS extension (the silver bullet), built-in geometric type support, GiST indexes
-
Search Indexing: Full-text search indexing sufficient for simple scenarios; rich index types, support for functional indices, conditional indices
-
NoSQL: Native support for JSON, JSONB, XML, HStore, and Foreign Data Wrappers to NoSQL databases
-
Data Warehousing: Smooth migration to GreenPlum, DeepGreen, HAWK, and others in the PG ecosystem, using FDW for ETL
-
Graph Data: Recursive queries
-
Caching: Materialized views
With Extensions as the Six Instruments, to honor Heaven, Earth, and the Four Directions.
With Greenplum to honor Heaven,
With Postgres-XL to honor Earth,
With Citus to honor the East,
With TimescaleDB to honor the South,
With PipelineDB to honor the West,
With PostGIS to honor the North.
— “The Rites of PG”
In Tantan’s (a popular dating app) legacy architecture, the entire system was designed around PostgreSQL. With millions of daily active users, millions of global DB-TPS, and hundreds of terabytes of data, they used PostgreSQL as their only data component. Independent data warehouses, message queues, and caches were only introduced later. And this is just the validated scale - further squeezing PostgreSQL’s potential is entirely feasible.
So, within a considerable scale, PostgreSQL can play the role of a jack-of-all-trades, one component serving as many. While it may not match specialized components in certain domains, it still performs admirably in all of them. And choosing a single data component can dramatically reduce project complexity, which means massive cost savings. It turns what would require ten people into something one person can handle.
Designing for scale you don’t need is wasted effort - a form of premature optimization. Only when no single software can meet all your requirements does the trade-off between splitting and integration become relevant. Integrating heterogeneous technologies is incredibly tricky work. If there’s one technology that can satisfy all your needs, using it is the best choice rather than trying to reinvent it with multiple components.
When business scale grows to a certain threshold, you may have no choice but to use a microservice/bus-based architecture and split database functionality into multiple components. But PostgreSQL’s existence significantly pushes back the threshold for this trade-off, and even after splitting, it continues to play a crucial role.
Operations-Friendly
Beyond its powerful features, another significant advantage of Pg is that it’s operations-friendly. It offers many practical capabilities:
-
DDL can be placed in transactions: dropping tables, TRUNCATE, creating functions, and indices can all be placed in transactions for atomic effect or rollback.
This enables some clever maneuvers, like swapping two tables via RENAME in a single transaction (the database equivalent of a chess castling move).
-
Concurrent creation and deletion of indices, adding non-null fields, and reorganizing indices and tables (without table locks).
This means you can make significant schema changes to production systems without downtime, optimizing indices as needed.
-
Various replication methods: segment replication, streaming replication, trigger-based replication, logical replication, plugin replication, and more.
This makes zero-downtime data migration remarkably easy: replicate, redirect reads, redirect writes - three steps, and your production migration is rock solid.
-
Diverse commit methods: asynchronous commits, synchronous commits, quorum-based synchronous commits.
This means Pg allows trade-offs between consistency and availability - for example, using synchronous commits for transaction databases and asynchronous commits for regular databases.
-
Comprehensive system views make building monitoring systems straightforward.
-
FDW (Foreign Data Wrappers) makes ETL incredibly simple - often just a single SQL statement.
FDW conveniently allows one instance to access data or metadata from other instances. It’s incredibly useful for cross-partition operations, database monitoring metric collection, data migration, and connecting to heterogeneous data systems.
Healthy Ecosystem
PostgreSQL’s ecosystem is thriving with an active community.
Compared to MySQL, PostgreSQL has a huge advantage in its friendly license. PG uses a PostgreSQL license similar to BSD/MIT, which essentially means as long as you’re not falsely claiming to be PostgreSQL, you can do whatever you want - even rebrand and sell it. No wonder so many “domestic databases” or “self-developed databases” are actually just rebranded or extended PG products.
Of course, many derivative products contribute back to the trunk. For instance, timescaledb
, pipelinedb
, and citus
- originally “databases” based on PG - all eventually became native PG plugins. Often when you need some functionality, a quick search reveals existing plugins or implementations. That’s the beauty of open source - a certain idealism prevails.
PG’s code quality is exceptionally high, with crystal-clear comments. Reading the C code feels almost like reading Go - the code practically serves as documentation. You can learn a lot from it. In contrast, with other databases like MongoDB, one glance and I lost all interest in reading further.
As for MySQL, the community edition uses the GPL license, which is quite painful. Without GPL’s viral nature, would there be so many open-source MySQL derivatives? Plus, MySQL is in Oracle’s hands - letting someone else hold your future isn’t wise, especially when that someone is an industry toxin. Facebook’s React license controversy serves as a cautionary tale.
Challenges
Of course, there are some drawbacks or regrets:
- Due to MVCC, the database needs periodic VACUUM maintenance to prevent performance degradation.
- No good open-source cluster monitoring solution (or they’re ugly!), so you need to build your own.
- Slow query logs are mixed with regular logs, requiring custom parsing.
- Official Pg lacks good columnar storage, a minor disappointment for data analysis.
These are just minor issues. The real challenge might not be technical at all…
At the end of the day, MySQL truly is the most popular open-source relational database. Java developers, PHP developers - many people start with MySQL, making it harder to recruit PostgreSQL talent. Often you need to train people yourself. Looking at DB Engines popularity trends, though, the future looks bright.
Final Thoughts
Learning PostgreSQL has been fascinating - it showed me that databases can do far more than just CRUD operations. While SQL Server and MySQL were my gateway to databases, it was PostgreSQL that truly revealed the magical world of database possibilities.
I’m writing this because an old post of mine on Zhihu was dug up, reminding me of my early days discovering PostgreSQL (https://www.zhihu.com/question/20010554/answer/94999834). Now that I’ve become a full-time PG DBA, I couldn’t resist adding more to that old grave. “The melon seller praising her own melons” - it’s only right that I praise PG. Hehe…
Full-stack engineers deserve full-stack databases.
I’ve compared MySQL and PostgreSQL myself and had the rare freedom to choose at Alibaba, a MySQL-dominated world. From a purely technical perspective, I believe PG absolutely crushes MySQL. Despite significant resistance, I eventually implemented and promoted PostgreSQL. I’ve used it for numerous projects, solving countless requirements (from small statistical reports to major revenue-generating initiatives). Most requirements were handled by PG alone, with occasional use of MQ and NoSQL (Redis, MongoDB, Cassandra/HBase). PG is truly addictive.
Eventually, my love for PostgreSQL led me to specialize in it full-time.
In my first job, I experienced the sweetness firsthand - with PostgreSQL, one person’s development efficiency rivals that of a small team:
-
Don’t want to write backends? PostGraphQL generates GraphQL APIs directly from database schema definitions, automatically listening for DDL changes and generating corresponding CRUD methods and stored procedure wrappers. Similar tools include PostgREST and pgrest. They’re perfectly usable for small to medium-sized applications, eliminating half the backend development work.
-
Need Redis functionality? Just use Pg - it can simulate standard features effortlessly, and you can skip the cache layer entirely. Implement Pub/Sub using Notify/Listen/Trigger to broadcast configuration changes and implement controls conveniently.
-
Need analytics? Window functions, complex JOINs, CUBE, GROUPING, custom aggregates, custom languages - it’s exhilarating. If you need to scale out, use the citus extension (or switch to Greenplum). It might lack columnar storage compared to data warehouses, but it has everything else.
-
Need geographic functionality? PostGIS is magical - solving complex geographic requirements in a single SQL line that would otherwise require thousands of lines of code.
-
Storing time-series data? The timescaledb extension may not match specialized time-series databases, but it still handles millions of records per second. I’ve used it to solve hardware sensor log storage and monitoring system metrics storage requirements.
-
For stream computing functionality, PipelineDB can define streaming views directly: UV, PV, real-time user profiles.
-
PostgreSQL’s FDW (Foreign Data Wrappers) is a powerful mechanism allowing access to various data sources through a unified SQL interface. Its applications are endless:
-
- The built-in
file_fdw
extension can connect any program’s output to a data table. The simplest application is system information monitoring. - When managing multiple PostgreSQL instances, you can use the built-in
postgres_fdw
to import data dictionaries from all remote databases into a metadata database. You can access metadata from all database instances uniformly, pull real-time metrics from all databases with a single SQL statement - building monitoring systems becomes a breeze. - I once used hbase_fdw and MongoFDW to wrap historical batch data from HBase and real-time data from MongoDB into PostgreSQL tables. A single view elegantly implemented a Lambda architecture combining batch and stream processing.
- Use
redis_fdw
for cache update pushing;mongo_fdw
for data migration from MongoDB to PG;mysql_fdw
to read MySQL data into data warehouses; implement cross-database or even cross-component JOINs; complete complex ETL operations with a single SQL line that would otherwise require hundreds of lines of code - how marvelous is that?
- The built-in
-
Rich types and method support: JSON for generating frontend JSON responses directly from the database - effortless and comfortable. Range types elegantly solve edge cases that would otherwise require programmatic handling. Other examples include arrays, multidimensional arrays, custom types, enumerations, network addresses, UUIDs, and ISBNs. These out-of-the-box data structures save programmers tremendous wheel-reinventing effort.
-
Rich index types: general-purpose Btree indices; Brin indices that significantly optimize sequential access; Hash indices for equality queries; GIN inverted indices; GIST generalized search trees efficiently supporting geographic and KNN queries; Bitmap simultaneously leveraging multiple independent indices; Bloom efficient filtering indices; conditional indices that can dramatically reduce index size; function indices that elegantly replace redundant fields. MySQL offers pathetically few index types by comparison.
-
Stable, reliable, correct, and efficient. MVCC easily implements snapshot isolation, while MySQL’s RR isolation level is deficient, unable to avoid PMP and G-single anomalies. Plus, implementations based on locks and rollback segments have various pitfalls; PostgreSQL can implement high-performance serializability through SSI.
-
Powerful replication: WAL segment replication, streaming replication (appearing in v9: synchronous, semi-synchronous, asynchronous), logical replication (appearing in v10: subscription/publication), trigger replication, third-party replication - every type of replication you could want.
-
Operations-friendly: DDL can be executed in transactions (rollback-capable), index creation without table locks, adding new columns (without default values) without table locks, cleaning/backup without table locks. System views and monitoring capabilities are comprehensive.
-
Numerous extensions, rich features, and extreme customizability. In PostgreSQL, you can write functions in any language: Python, Go, JavaScript, Java, Shell, etc. Rather than calling Pg a database, it’s more accurate to call it a development platform. I’ve experimented with many useless but fun things: in-database crawlers, recommendation systems, neural networks, web servers, and more. There are various third-party plugins with powerful functions or creative ideas: https://pgxn.org/.
-
PostgreSQL’s license is friendly - BSD lets you do whatever you want. No wonder so many databases are rebranded PG products. MySQL has GPL viral infection and remains under Oracle’s thumb.